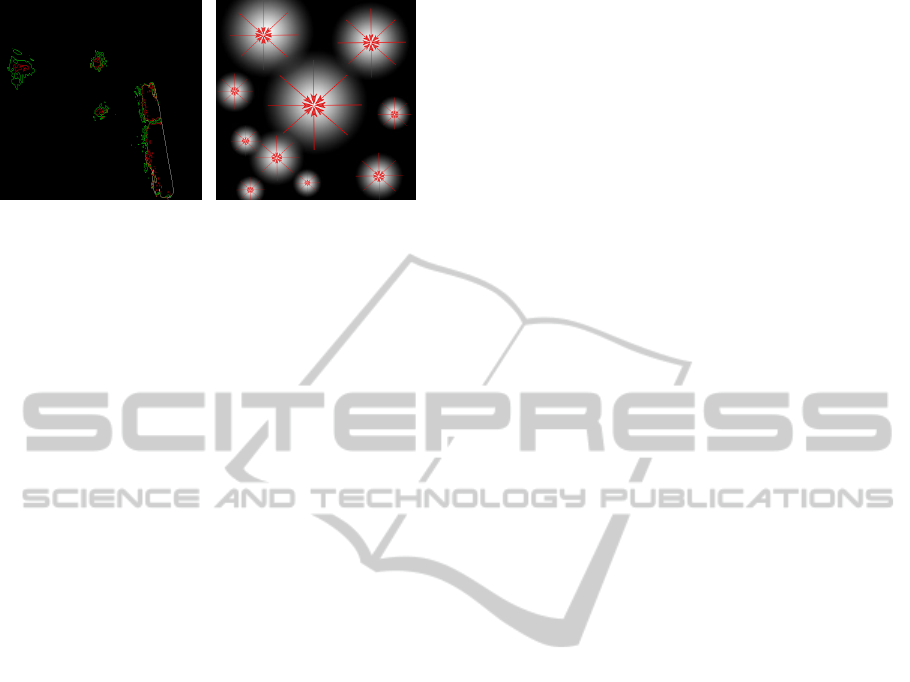
(a) (b)
Figure 8: Our proposed evaluation methods for specularity
detection : contour and gravity center evaluation. (a) shows
differences between the two methods using the ground truth
(in green) and the current detection (in red). Common pix-
els are colored in gray. (b) illustrates the gravity center es-
timation. The red lines represent the direction vector of the
gradient around the specularity.
experimental protocol based on two properties: accu-
racy of the contour and the gravity center of a specular
reflection.
6 DISCUSSION AND FUTURE
WORK
State-of-the art approaches on specular reflections de-
tection are threshold based methods. Nevertheless, re-
lying on strong reflection models such as Lambertian
model (Brelstaff and Blake, 1988) or separating dif-
fuse and specular components (Tan et al., 2004) could
be relevant to increase accuracy and noise reduction.
Some applications such as (Jachnik et al., 2012) and
(Lagger et al., 2008) for 3D pose estimation or refine-
ment of the light sources or (Karsch et al., 2011) re-
quire a real-time specular reflections detector because
they are dealing with video stream. Moreover, a video
stream contains a huge amount of information to pro-
vide accurate misdetection correction such as white
textures in an image to another (see example in (Lee
and Bajcsy, 1992) or (Feris et al., 2006)). Our method
could be further improved by using multi-view infor-
mation and is fast enough to handle each frame in
real-time.
REFERENCES
Arnold, M., Ghosh, A., Ameling, S., and Lacey, G. (2010).
Automatic segmentation and inpainting of specular
highlights for endoscopic imaging. Journal on Image
and Video Processing, 2010:9.
Blake, A. and Brelstaff, G. (1988). Geometry from spec-
ularities. International Conference on Computer Vi-
sion, pages 394–403.
Brelstaff, G. and Blake, A. (1988). Detecting specular
reflections using lambertian constraints. In Interna-
tional Conference on Computer Vision, ICCV.
Deng, Y., Manjunath, B., and Shin, H. (1999). Color image
segmentation. In Computer Vision and Pattern Recog-
nition, CVPR.
Feris, R., Raskar, R., Tan, K.-H., and Turk, M. (2006).
Specular highlights detection and reduction with
multi-flash photography. Journal of the Brazilian
Computer Society, 12(1):35–42.
Jachnik, J., Newcombe, R. A., and Davison, A. J. (2012).
Real-time surface light-field capture for augmentation
of planar specular. In International Symposium on
Mixed and Augmented Reality, ISMAR.
Karsch, K., Hedau, V., Forsyth, D., and Hoiem, D. (2011).
Rendering synthetic objects into legacy photographs.
ACM Transactions on Graphics (TOG), 30(6):157.
Lagger, P., Salzmann, M., Lepetit, V., and Fua, P. (2008). 3d
pose refinement from reflections. In Computer Vision
and Pattern Recognition, CVPR.
Lambert, J. H. and DiLaura, D. L. (2001). Photometry, or,
on the measure and gradations of light, colors, and
shade:translation from the Latin of photometria, sive,
de mensura et gradibus luminis, colorum et umbrae.
Lee, S.-T., Yoon, T.-H., Kim, K.-S., Kim, K.-D., and Park,
W. (2010). Removal of specular reflections in tooth
color image by perceptron neural nets. In Interna-
tional Conference on Signal Processing Systems, IC-
SPS.
Lee, S. W. and Bajcsy, R. (1992). Detection of specularity
using color and multiple views. In European Confer-
ence on Computer Vision, ECCV.
Oh, J., Hwang, S., Lee, J., Tavanapong, W., Wong, J., and
de Groen, P. C. (2007). Informative frame classifica-
tion for endoscopy video. Medical Image Analysis,
11(2):110–127.
Ortiz, F. and Torres, F. (2005). A new inpainting method
for highlights elimination by colour morphology. In
Pattern Recognition and Image Analysis, pages 368–
376.
Ortiz, F. and Torres, F. (2006). Automatic detection and
elimination of specular reflectance in color images by
means of ms diagram and vector connected filters.
Systems, Man, and Cybernetics, Part C: Applications
and Reviews, 36(5):681–687.
Park, J. B. and Kak, A. C. (2003). A truncated least
squares approach to the detection of specular high-
lights in color images. In International Conference
on Robotics and Automation, ICRA.
Saint-Pierre, C.-A., Boisvert, J., Grimard, G., and Cheriet,
F. (2011). Detection and correction of specular reflec-
tions for automatic surgical tool segmentation in tho-
racoscopic images. Machine Vision and Applications,
22(1):171–180.
Shafer, S. A. (1985). Using color to separate reflection com-
ponents. Color Research & Application, 10(4):210–
218.
Sillion, F. and Puech, C. (1989). A general two-pass method
integrating specular and diffuse reflection. In Special
Interest Group on GRAPHics and Interactive Tech-
niques, SIGGRAPH.
GenericandReal-timeDetectionofSpecularReflectionsinImages
281