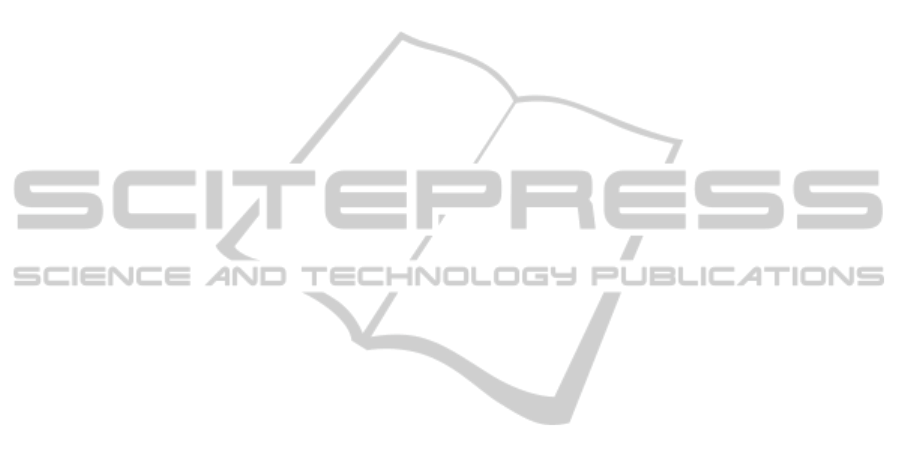
ACKNOWLEDGEMENTS
This research is supported by the FES project COM-
MIT. Furthermore, we would like to thank Renaud
P
´
eteri for providing access to the DynTex database.
REFERENCES
Beauchemin, S. and Barron, J. (1995). The computation
of optical flow. ACM Computing Surveys, 27(3):433–
466.
Bochkanov, S. (1999-2013). Alglib software library
(www.alglib.net).
Boykov, Y. and Kolmogorov, V. (2004). An experimental
comparison of min-cut/max-flow algorithms for en-
ergy minimization in vision. PAMI.
Chan, A. and Vasconcelos, N. (2008). Modeling, cluster-
ing, and segmenting video with mixtures of dynamic
textures. PAMI, 30(5):909–926.
Criminisi, A., Shotton, J., and Konukoglu, E. (2012). De-
cision forests. Foundations and Trends in Computer
Graphics and Vision, 7(2):81–227.
Doretto, G., Cremers, D., Favaro, P., and Soatto, S. (2003).
Dynamic texture segmentation. ICCV, 2:1236–1242.
Fazekas, S. and Chetverikov, D. (2007). Analysis and per-
formance evaluation of optical flow features for dy-
namic texture recognition. SPIC, 22:680–691.
Hu, D., Bo, L., and Ren, X. (2011). Toward robust material
recognition for everyday objects. BMVC, pages 48.1–
48.11.
Kontschieder, P., Kohli, P., Shotton, J., and Criminisi, A.
(2013). Geof: Geodesic forests for learning coupled
predictors. CVPR.
Mumtaz, A., Coviello, E., Lanckriet, G., and Chan, A.
(2013). Clustering dynamic textures with the hier-
archical em algorithm for modeling video. PAMI,
35(7):1606–1621.
P
´
eteri, R. and Chetverikov, D. (2005). Dynamic texture
recognition using normal flow and texture regularity.
PRIA, 3523:223–230.
P
´
eteri, R., Fazekas, S., and Huiskes, M. (2010). Dyntex: A
comprehensive database of dynamic textures. Pattern
Recognition Letters, 31(12):1627–1632.
Rankin, A. and Matthies, L. (2006). Daytime water de-
tection and localization for unmanned ground vehicle
autonomous navigation. Proceeding of the 25th Army
Science Conference.
Saisan, P., Doretto, G., Wu, Y. N., and Soatto, S. (2001).
Dynamic texture recognition. CVPR, 2:II–58–II–63.
Schwind, R. (1991). Polarization vision in water insects and
insects living on a moist substrate. Journal of Com-
parative Physiology A, 169(5):531–540.
Sharan, L., Liu, C., Rosenholtz, R., and Adelson, E.
(2013). Recognizing materials using perceptually in-
spired features. IJCV, pages 1–24.
Sharan, L., Rosenholtz, R., and Adelson, E. (2009). Mate-
rial perception: What can you see in a brief glance?
[abstract]. Journal of Vision, 9(8):784.
Smith, A., Teal, M., and Voles, P. (2003). The statisti-
cal characterization of the sea for the segmentation of
maritime images. Video/Image Processing and Multi-
media Communications, 2:489–494.
Snoek, C., Worring, M., and Smeulders, A. (2005). Early
versus late fusion in semantic video analysis. In Pro-
ceedings of the 13th annual ACM international con-
ference on Multimedia, pages 399–402. ACM.
Tenenbaum, J., de Silva, V., and Langford, J. (2000). A
global geometric framework for nonlinear dimension-
ality reduction. Science, 290(5500):2319–2323.
Varma, M. and Zisserman, A. (2005). A statistical approach
to texture classification from single images. IJCV,
62(1):61–81.
Varma, M. and Zisserman, A. (2009). A statistical ap-
proach to material classification using image patch ex-
emplars. PAMI, 31(11):2032–2047.
Zhao, G. and Pietik
¨
ainen, M. (2006). Local binary pattern
descriptors for dynamic texture recognition. ICPR,
2:211–214.
Zhao, G. and Pietik
¨
ainen, M. (2007). Dynamic texture
recognition using local binary patterns with an appli-
cation to facial expressions. PAMI, 29(6):915–928.
VISAPP2014-InternationalConferenceonComputerVisionTheoryandApplications
292