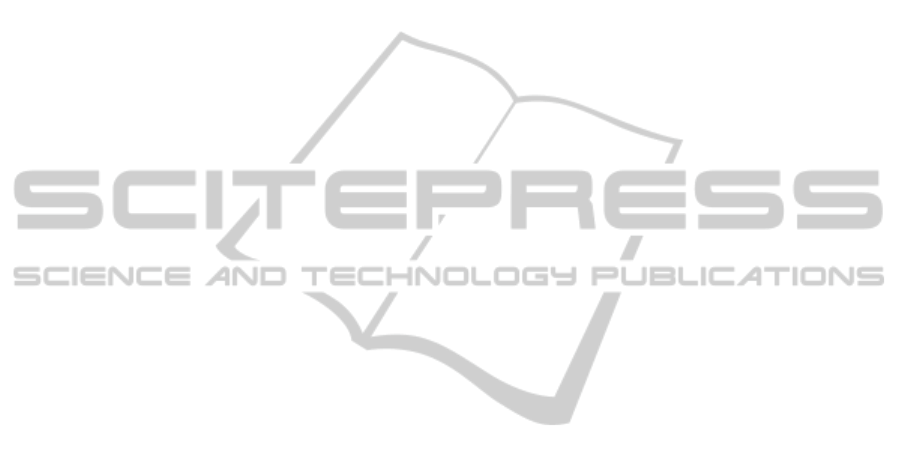
5 CONCLUSIONS
In this work we studied how to combine person detec-
tors and re-identification algorithms towards the de-
velopment of fully automated surveillance systems.
Whereas current work on re-identification focuses on
matching “clean data”, we analyse the effect of the
unavoidable “noise” produced by automatic detec-
tions methods and devise ways to deal with it. In
particular, a correct treatment of false positives and
occlusions can recover performance levels that are
lost if the modules are naively combined. This re-
quires some additional characterisation of the acqui-
sition process, in terms of collecting false positives
in the environment where the system is deployed and
performing a geometrical analysis to define the over-
lap filter criteria. This effort is largely compensated
by the obtained improvements. In future work we aim
at extending our methodology and evaluation criteria
to drop the closed-space assumption and address the
effect of missed detections in the quality and usability
of fully automatic re-identification systems.
ACKNOWLEDGEMENTS
This work was partially supported by the FCT
project [PEst-OE/EEI/LA0009/2013], the European
Commission project POETICON++ (FP7-ICT-
288382), the FCT project VISTA [PTDC/EIA-
EIA/105062/2008] and the project High Definition
Analytics (HDA), QREN - I&D em Co-Promoc¸
˜
ao
13750.
REFERENCES
Andriluka, M., Roth, S., and Schiele, B. (2009). Picto-
rial Structures Revisited: People Detection and Artic-
ulated Pose Estimation. CVPR.
Bak, S., Corv
´
ee, E., Br
´
emond, F., and Thonnat, M. (2012).
Boosted human re-identification using Riemannian
manifolds. ImaVis.
Cheng, D. S., Cristani, M., Stoppa, M., Bazzani, L., and
Murino, V. (2011). Custom pictorial structures for re-
identification. In BMVC.
Corvee, E., Bak, S., and Bremond, F. (2012). People detec-
tion and re-identification for multi surveillance cam-
eras. VISAPP.
Dikmen, M., Akbas, E., Huang, T., and Ahuja, N. (2011).
Pedestrian recognition with a learned metric. In
ACCV.
Doll
´
ar, P., Belongie, S., and Perona, P. (2010). The Fastest
Pedestrian Detector in the West. BMVC.
Ess, A., Leibe, B., and Van Gool, L. (2007). Depth and
Appearance for Mobile Scene Analysis. ICCV.
Everingham, M., Van Gool, L., Williams, C., Winn, J., and
Zisserman, A. (2010). The Pascal visual object classes
(VOC) challenge. IJCV.
Farenzena, M., Bazzani, L., Perina, A., Murino, V., and
Cristani, M. (2010). Person re-identification by
symmetry-driven accumulation of local features. In
CVPR.
Figueira, D., Bazzani, L., Minh, H. Q., Cristani, M.,
Bernardino, A., and Murino, V. (2013). Semi-
supervised multi-feature learning for person re-
identification. AVSS.
Gheissari, N., Sebastian, T., and Hartley, R. (2006). Person
reidentification using spatiotemporal appearance. In
CVPR.
Girshick, R., Felzenszwalb, P., and McAllester, D. (2011).
Object detection with grammar models. PAMI.
Gray, D. and Tao, H. (2008). Viewpoint invariant pedestrian
recognition with an ensemble of localized features. In
ECCV.
Hamdoun, O., Moutarde, F., Stanciulescu, B., and Steux.,
B. (2008). Person re-identification in multi-camera
system by signature based on interest point descriptors
collected on short video sequences. In ICDSC.
Harandi, M. T., Sanderson, C., Wiliem, A., and Lovell,
B. C. (2012). Kernel analysis over Riemannian mani-
folds for visual recognition of actions, pedestrians and
textures. In WACV.
Hirzer, M., Roth, P., Kostinger, M., and Bischof, H.
(2012). Relaxed pairwise learned metric for person
re-identification. In ECCV.
Jungling, K., Bodensteiner, C., and Arens, M. (2011). Per-
son re-identification in multi-camera networks. In
CVPRW.
Li, W. and Wang, X. (2013). Locally aligned feature trans-
forms across views. In CVPR.
Li, W., Zhao, R., and Wang, X. (2012). Human reidentifi-
cation with transferred metric learning. In ACCV.
Liu, C., Gong, S., Loy, C., and Lin, X. (2012a). Person re-
identification: What features are important? In ECCV.
Liu, C., Wang, G., Lin, X., and Li, L. (2012b). Person re-
identification by spatial pyramid color representation
and local region matching. IEICE.
Ma, B., Su, Y., and Jurie., F. (2012). Bicov: a novel
image representation for person re-identification and
face verification. In BMVC.
Mignon, A. and Jurie, F. (2012). Pcca: A new approach for
distance learning from sparse pairwise constraints. In
CVPR.
Mogelmose, A., Bahnsen, C., and Moeslung, T. B. (2013a).
Tri-modal person re-identification with RGB, depth
and thermal features. In IEEE WPBVS.
Mogelmose, A., Moeslund, T. B., and Nasrollahi, K.
(2013b). Multimodal person re-identification us-
ing RGB-D sensors and a transient identification
database. In IWBF.
Pedagadi, S., Orwell, J., Velastin, S., and Boghossian, B.
(2013). Local fisher discriminant analysis for pedes-
trian re-identification. In CVPR.
Prosser, B., Zheng, W., Gong, S., Xiang, T., and Mary.,
Q. (2010). Person re-identification by support vector
ranking. In BMCV.
VISAPP2014-InternationalConferenceonComputerVisionTheoryandApplications
146