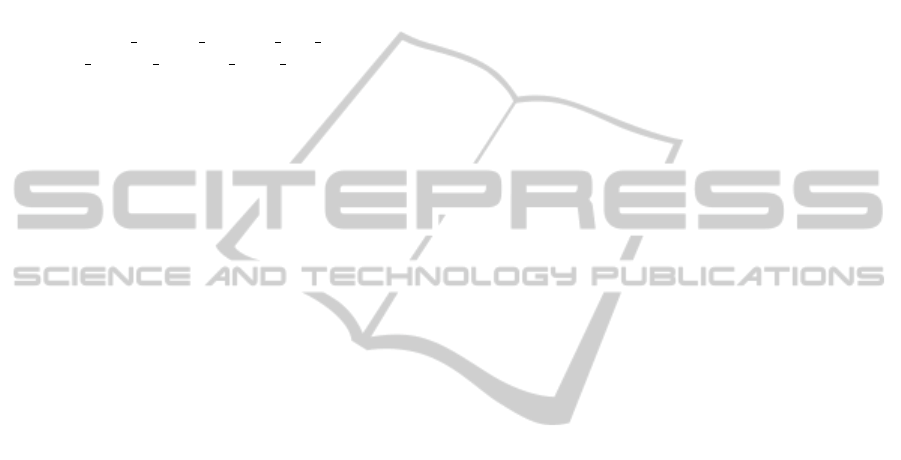
left side, which might explain low detection quality.
Nevertheless, Figure 12 shows that stagnancy in those
unusual zones mostly occurs outside of peak hours.
During peak hours, there are too many people for a
group to comfortably stagnate in one of those transit
zones.
Figure 12 also shows other trajectories taken
by groups but the occurrence rate of these tra-
jectories is lower than those in Figure 10. We
have detected a few groups passing through the
station without entering the turnstiles (17 occur-
rences of “Group Passing Through Left Right” and
1 of “Group Passing Through Right Left”), 2 occur-
rences of groups waiting in the hall and then leaving
the station. Finally, we have detected 8 occurrences of
groups waiting in the hall before entering the station
and taking the stairs. The rarity of these detections
might be explained by the difficulty to detect such
events, especially during peak hours.
5 CONCLUSIONS
We have presented an approach for group tracking
and behavior recognition. Our approach works in real
time and in long surveillance videos (1 month per
view). In the experiment section, we have shown that
our method is capable of recognizing multiple and
different events at the same time. This demonstrates
the robustness of our approach to processing videos
regardless of their length. Thanks to the simplicity of
using the event recognition language (ScReK), it was
possible to model complex scenarios for the Tornelli
scene as shown in Figure 8.
On the other hand, the amount of videos processed
allows us to analyze the results, obtaining the different
comparisons presented in the graphs of the previous
section.
We consider for future work the addition of prob-
abilities to the event recognition phase, which could
help to predict dangerous and criminal events. The
results obtained here show us a big challenge for pre-
diction of such events because there are not many ex-
amples with this kind of behaviors.
ACKNOWLEDGEMENTS
The research leading to these results has received
funding from the European Communitys Seventh
Framework Programme FP7/2007-2013 - Challenge
2- Cognitive Systems, Interaction, Robotics - under
grant agreement n 248907-VANAHEIM.
REFERENCES
Badie, J., Bak, S., Serban, S., and Bremond, F. (2012).
Recovering people tracking errors using enhanced
covariance-based signatures. In International Work-
shop on Performance Evaluation of Tracking and
Surveillance (PETS).
Bak, S., Corvee, E., Bremond, F., and Thonnat, M. (2011).
Multiple shot human re-identification by mean rie-
mannian covariance grid. In International Confer-
ence on Advanced Video and Signal Based Surveil-
lance (AVSS).
Chang, M., Krahnstoever, N., and Ge, W. (2011). Prob-
abilistic group-level motion analysis and scenario
recognition. In International Conference on Computer
Vision (ICCV).
Chau, D., Thonnat, M., and Bremond, F. (2013). Automatic
parameter adaptation for multi-object tracking. In In-
ternational Conference on Computer Vision Systems
(ICVS).
Fukunaga, K. and Hostetler, L. (1975). The estimation of
the gradient of a density function, with applications in
pattern recognition. In IEEE trans. Information The-
ory.
Guo, P., Miao, Z., Shen, Y., and Cheng, H. (2010). Real
time human action recognition in a long video se-
quence. In International Conference on Advanced
Video and Signal-Based Surveillance (AVSS).
McKenna, S. J., Jabri, S., Duric, Z., Rosenfeld, A., and
Wechsler, H. (2000). Tracking groups of people. In
Computer Vision and Image Understanding.
McPhail, C. and Wohlstein, R. T. (1982). Using film to
analyze pedestrian behavior. In Sociological Methods
& Research.
Nghiem, A., Bremond, F., and Thonnat, M. (2009). Con-
trolling background subtraction algorithms for robust
object detection. In International Conference on
Imaging for Crime Detection and Prevention (ICDP).
Ryoo, M. and Aggarwal, J. (2010). Stochastic representa-
tion and recognition of high-level group activities. In
International Journal of Computer Vision (IJCV).
Vishwakarma, S. and Agrawal, A. (2012). A survey on ac-
tivity recognition and behavior understanding in video
surveillance. In The Visual Computer.
Zaidenberg, S., Boulay, B., and Breemond, F. (2012). A
generic framework for video understanding applied to
group behavior recognition. In International Confer-
ence on Advanced Video and Signal-Based Surveil-
lance (AVSS).
Zaidenberg, S., Boulay, B., Garate, C., Chau, D., Corvee,
E., and Bremond, F. (2011). Group interaction and
group tracking for video-surveillance in underground
railway stations. In International Workshop on Be-
haviour Analysis and Video Understanding (ICVS).
VISAPP2014-InternationalConferenceonComputerVisionTheoryandApplications
402