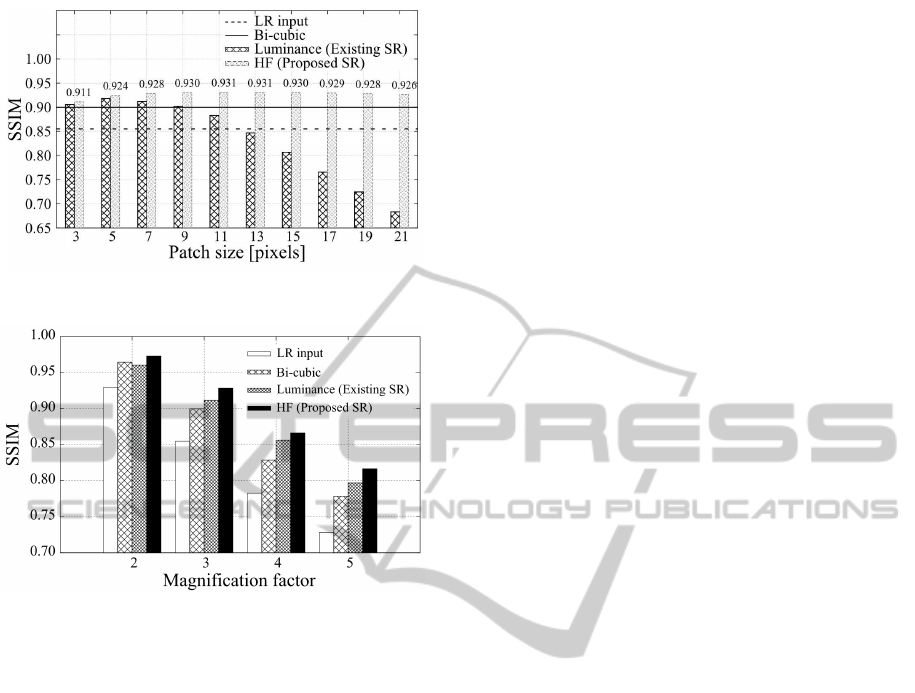
Figure 7: SSIM for different patch sizes.
Figure 8: SSIM for different magnification factors.
ACKNOWLEDGEMENTS
This work was supported by the “R&D Program for
Implementation of Anti-Crime and Anti-Terrorism
Technologies for a Safe and Secure Society,” Special
Coordination Fund for Promoting Science and Tech-
nology of the Ministry of Education, Culture, Sports,
Science and Technology, the Japanese Government.
The authors wish to thank the members of Murase
laboratory participating in the video recording used
in the experiment.
REFERENCES
Baker, S. and Kanade, T. (2000). Hallucinating faces. In
Proc. IEEE Fourth Int’l Conf. Automatic Face and
Gesture Recognition (FG’00), pages 83–88.
Baker, S. and Kanade, T. (2002). Limits on super-resolution
and how to break them. IEEE Trans. Pattern Analysis
and Machine Intelligence, 24(9):1167–1183.
Bonet, J. S. D. (1997). Multiresolution sampling procedure
for analysis and synthesis of texture images. In Proc.
ACM 24th Int’l Conf. Computer Graphics and Inter-
active Techniques (SIGGRAPH’97), pages 361–368.
Freeman, W. T., Jones, T. R., and Pasztor, E. C. (2002).
Example-based super-resolution. IEEE Trans. Com-
puter Graphics and Applications, 22(2):56–65.
Ho, T. and Zeng, B. (2012). Super-resolution image
by curve fitting in the threshold decomposition do-
main. Visual Communication and Image Represen-
tation, 23(1):208–221.
Jiang, J., , Hu, R., Han, Z., Lu, T., and Huang, K. (2012a).
Position-patch based face hallucination via locality-
constrained representation. In Proc. IEEE 13th Int’l
Conf. on Multimedia and Expo (ICME’12), pages
212–217.
Jiang, J., Hu, R., Han, Z., Huang, K., and Lu, T. (2012b).
Efficient single image super-resolution via graph em-
bedding. In Proc. IEEE 13th Int’l Conf. on Multimedia
and Expo (ICME’12), pages 610–615.
Lin, Z. and Shum, H. Y. (2004). Fundamental limits of
reconstruction-based superresolution algorithms un-
der local translation. IEEE Trans. Pattern Analysis
and Machine Intelligence, 26(1):83–97.
Liu, C., Shum, H. Y., and Freeman, W. T. (2007). Face hal-
lucination: Theory and practice. ACM Trans. Com-
puter Vision, 75(1):115–134.
Ma, X., Li, W., Xu, H., Yang, X., and Song, H. (2013). A
general residue compensation framework of learning-
based face super-resolution. Computational Informa-
tion Systems, 9(10):4049–4056.
Milanfar, P. (2011). Super-Resolution Imaging (Digital
Imaging and Computer Vision). CRC Press.
Muja, M. and Lowe, D. G. (2009). Fast approximate nearest
neighbors with automatic algorithm configuration. In
Proc. Fourth Int’l Conf. Computer Vision Theory and
Applications (VISSAP’09), pages 331–340.
Nakajima, C., Pontil, M., Heisele, B., and Poggio, T.
(2003). Full-body person recognition system. Trans.
Pattern Recognition in Kernel and Subspace Methods
for Computer Vision, 36(9):1997–2006.
Shibata, T., Iketani, A., and Senda, S. (2013). Single image
super resolution reconstruction in perturbed exemplar
sub-space. In Proc. IEEE 12th Conf. Asian Confer-
ence on Computer Vision (ACCV’13), pages 401–412.
Wang, J. T., Liang, K. W., Chang, S. F., and Chang, P. C.
(2009). Super-resolution image with estimated high
frequency compensated algorithm. In Proc. 9th Int’l
Symp. Communications and Information Technology
(ISCIT’09), pages 175–180.
Wang, Z., Bovik, A. C., Sheikh, H. R., and Simoncelli, E. P.
(2004). Image quality assessment: From error visi-
bility to structural similarity. IEEE Trans. Image Pro-
cessing, 13(4):600–612.
Yoshida, T., Takahashi, T., Deguchi, D., Ide, I., and Murase,
H. (2012). Robust face super-resolution using free-
form deformations for low-quality surveillance video.
In Proc. IEEE 13th Int’l Conf. on Multimedia and
Expo (ICME’12), pages 368–373.
Zeyde, R., Elad, M., and Protter, M. (2010). On single im-
age scale-up using sparse representation. In Proc. 7th
Int’l Conf. Curves and Surfaces, pages 711–730.
Exemplar-basedHumanBodySuper-resolutionforSurveillanceCameraSystems
121