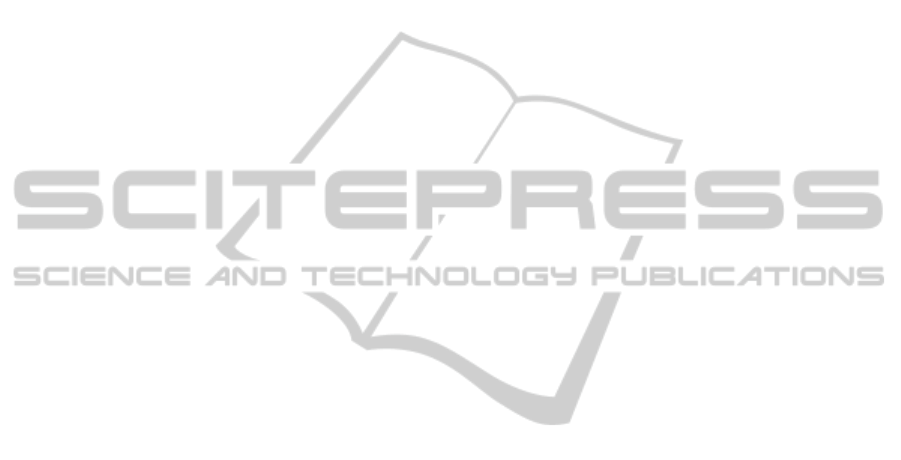
proposed approach has achieved these very compet-
itive scores by using a single feature space (gray
SIFT features), which was not the case for the other
participants that relied on more than one feature
spaces (Thomee and Popescu, 2012).
5 CONCLUSIONS
In this paper, we propose an automatic variation of
active learning for image classification adjusted in
the context of social media. This adjustment con-
sists in replacing the typical human oracle with user
tagged images obtained from social sites and in us-
ing a probabilistic approach for jointly maximizing
the informativeness of the samples and the oracle’s
confidence. The results show that in this context it
is critical to jointly consider these two quantities for
successfully selecting additional samples to enhance
the initial training set. Additionally, we noticed that
the na¨ıve oracle performs very well on concepts that
depict strong visual content corresponding to typical
foreground visual objects (e.g fish, spider, bird and
baby), while the proposed approach copes better with
more abstract and ambiguous concepts (e.g. flames,
smoke, strangers and circular wrap), since the utilized
textual classifier accounts for the context of the tags
as well.
Finally, an interesting note is that the difficult con-
cepts (i.e. models with low performance) tend to gain
much more in terms of effectiveness from such boot-
strapping methods, as shown in Fig. 5. Similar con-
clusions are drawn when comparing the proposed ap-
proach, which trained a simple SVM classifier using
a single feature space to the more sophisticated ap-
proaches of the ImageCLEF 2012 challenge, which
typically used many feature spaces. Especially in the
case of difficult concepts, as shown by the superiority
of the proposed approach based on the GmiAP metric,
we can also conclude that it is more important to find
more positive samples than more sophisticated algo-
rithms.
Our plans for future work include the use of flickr
groups as a richer and more large-scale pool of can-
didates for positive samples and the extension of the
proposed approach to an on-line continuous learning
scheme.
ACKNOWLEDGEMENTS
This work was supported by the EU 7th Framework
Programme under grant number IST-FP7-288815 in
project Live+Gov (www.liveandgov.eu).
REFERENCES
Campbell, C., Cristianini, N., and Smola, A. J. (2000).
Query learning with large margin classifiers. In Pro-
ceedings of the Seventeenth International Conference
on Machine Learning, ICML ’00, pages 111–118, San
Francisco, CA, USA. Morgan Kaufmann Publishers
Inc.
Chatfield, K., Lempitsky, V., Vedaldi, A., and Zisserman,
A. (2011). The devil is in the details: an evaluation of
recent feature encoding methods. In British Machine
Vision Conference.
Chatzilari, E., Nikolopoulos, S., Kompatsiaris, Y., and Kit-
tler, J. (2012). Multi-modal region selection approach
for training object detectors. In Proceedings of the
2nd ACM International Conference on Multimedia
Retrieval, ICMR ’12, pages 5:1–5:8, New York, NY,
USA. ACM.
Cohn, D., Atlas, L., and Ladner, R. (1994). Improving
generalization with active learning. Mach. Learn.,
15(2):201–221.
Fang, M. and Zhu, X. (2012). I don’t know the label: Active
learning with blind knowledge. In Pattern Recognition
(ICPR), 2012 21st International Conference on, pages
2238–2241.
Freytag, A., Rodner, E., Bodesheim, P., and Denzler, J.
(2013). Labeling examples that matter: Relevance-
based active learning with gaussian processes. In We-
ickert, J., Hein, M., and Schiele, B., editors, GCPR,
volume 8142 of Lecture Notes in Computer Science,
pages 282–291. Springer.
Joachims, T. (1998). Text categorization with support vec-
tor machines: Learning with many relevant features.
In Ndellec, C. and Rouveirol, C., editors, Machine
Learning: ECML-98, volume 1398 of Lecture Notes
in Computer Science, pages 137–142. Springer Berlin
Heidelberg.
Li, X., Snoek, C. G. M., Worring, M., Koelma, D. C., and
Smeulders, A. W. M. (2013). Bootstrapping visual
categorization with relevant negatives. IEEE Transac-
tions on Multimedia, In press.
Lin, H.-T., Lin, C.-J., and Weng, R. C. (2007). A note
on platt’s probabilistic outputs for support vector ma-
chines. Machine Learning, 68(3):267–276.
Mark J. Huiskes, B. T. and Lew, M. S. (2010). New
trends and ideas in visual concept detection: The mir
flickr retrieval evaluation initiative. In MIR ’10: Pro-
ceedings of the 2010 ACM International Conference
on Multimedia Information Retrieval, pages 527–536,
New York, NY, USA. ACM.
Ng, V. and Cardie, C. (2003). Bootstrapping coreference
classifiers with multiple machine learning algorithms.
In Proceedings of the 2003 conference on Empirical
methods in natural language processing, EMNLP ’03,
pages 113–120.
Nowak, S. and R¨uger, S. (2010). How reliable are annota-
tions via crowdsourcing: a study about inter-annotator
agreement for multi-label image annotation. In Pro-
ceedings of the international conference on Multime-
dia information retrieval, MIR ’10, pages 557–566,
New York, NY, USA. ACM.
VISAPP2014-InternationalConferenceonComputerVisionTheoryandApplications
84