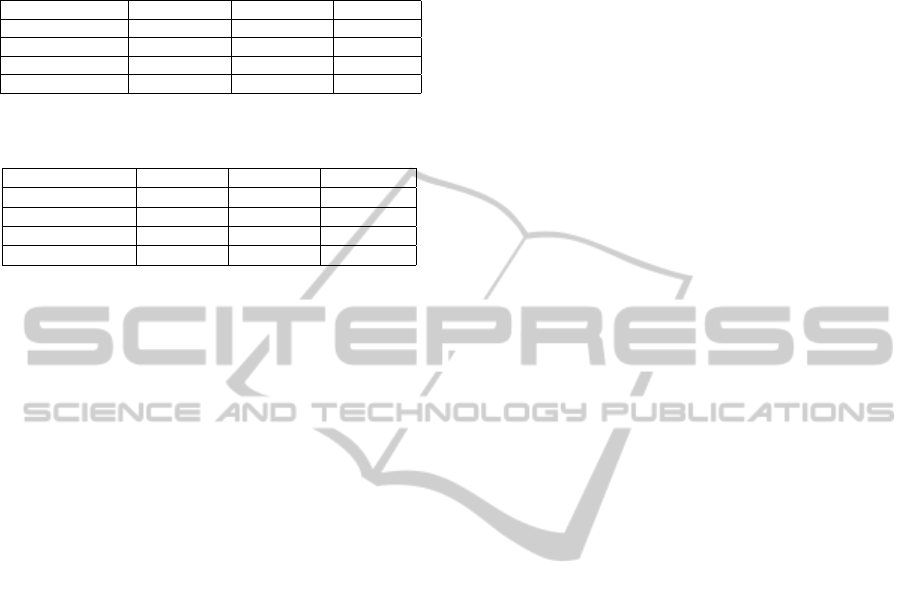
Table 2: Mean and standard deviation of the errors for the
3D face localization and the rotation angles. Tracking er-
rors are computed from four sequences recorded in chang-
ing and severe illumination conditions.
Method Rekik et al. IC- Our method
localization (mm) 50.50 (36.11) 59.11 (31.26) 9.50 (3.61)
yaw (
◦
) 6.90 (5.59) 33.87 (15.73) 3.17 (1.83)
pitch (
◦
) 15.78 (12.82) 12.52 (6.42) 3.32 (2.93)
roll (
◦
) 9.91 (7.22) 31.15 (11.85) 4.53 (3.32)
Table 3: Variation of the localization and orientation errors
according to the number of regions of the face.
Region number 3 regions 4 regions 6 regions
localization (mm) 9.50 (3.61) 4.40 (2.40) 3.60 (2.10)
yaw (
◦
) 3.17 (1.83) 2.36 (1.47) 1.18 (0.85)
pitch (
◦
) 3.32 (2.93) 2.87 (2.27) 3.55 (2.94)
roll (
◦
) 4.53 (3.32) 5.86 (3.14) 2.84 ( 1.87)
figure 3). Then, we have applied our method with the
different grouping. Table 3 presents the position and
the orientation errors according to the number of re-
gions of the face.
5 CONCLUSIONS
This paper presents a new approach for 3D face pose
tracking in illumination condition changes using color
and depth data from low-quality RGB-D cameras.
Our approach is based on a minimisation process
where the objective function combines photometric
and geometric energies. We have performed a quanti-
tative evaluation of the proposed method on the Biwi
Kinect Head Pose database, and we have demon-
strated the robustness of our method in case of ar-
bitrary illumination changes. Future work, will try
to ameliorate our tracking speed and will extend our
tracker to handle non-rigid facial motions by integrat-
ing the Candide facial deformation parameters in the
optimization process.
REFERENCES
Ahlberg, J. (2001). Candide-3 - an updated parameterised
face. Technical report.
Baltru
ˇ
saitis, T., Robinson, P., and Morency, L.-P. (2012). 3d
constrained local model for rigid and non-rigid facial
tracking. In IEEE CVPR, pages 2610–2617.
Ben-Hamadou, A., Soussen, C., Daul, C., Blondel, W., and
Wolf, D. (2010). Flexible projector calibration for ac-
tive stereoscopic systems. In 2010 IEEE International
Conference on Image Processing, pages 4241–4244,
Hong Kong, Hong Kong.
Ben-Hamadou, A., Soussen, C., Daul, C., Blondel, W., and
Wolf, D. (2013). Flexible calibration of structured-
light systems projecting point patterns. Computer Vi-
sion and Image Understanding, 117(10):1468–1481.
Cai, Q., Gallup, D., Zhang, C., and Zhang, Z. (2010). 3d de-
formable face tracking with a commodity depth cam-
era. In ECCV, pages 229–242.
Fanelli, G., Dantone, M., Gall, J., Fossati, A., and Van Gool,
L. (2013). Random forests for real time 3d face
analysis. International Journal of Computer Vision,
101(3):437–458.
Fanelli, G., Weise, T., Gall, J., and Gool, L. V. (2011). Real
time head pose estimation from consumer depth cam-
eras. In IEEE ICPR, pages 101–110.
Maurel, P. (2008). Shape gradients, shape warping and
medical application to facial expression analysis. PhD
thesis, Ecole Doctorale de Sciences Math
´
ematiques de
Paris Centre.
Murphy-Chutorian, E. and Trivedi, M. M. (2009). Head
pose estimation in computer vision: A survey. Pat-
tern Analysis and Machine Intelligence, IEEE Trans-
actions on, 31(4):607–626.
Nelder, J. A. and Mead, R. (1965). A simplex method
for function minimization. The computer journal,
7(4):308–313.
Padeleris, P., Zabulis, X., and Argyros, A. A. (2012). Head
pose estimation on depth data based on particle swarm
optimization. In IEEE CVPR Workshops, pages 42–
49.
Press, W. H., Teukolsky, S. A., Vetterling, W. T., and Flan-
nery, B. P. (2007). Numerical recipes: The art of sci-
entific computing. Cambridge Univ. Press, New York,
3rd edition.
Rekik, A., Ben-Hamadou, A., and Mahdi, W. (2013). 3d
face pose tracking using low quality depth cameras.
In VISAPP, pages 223–228.
Silveira, G. and Malis, E. (2007). Real-time visual tracking
under arbitrary illumination changes. In IEEE CVPR,
pages 1–6.
Smisek, J., Jancosek, M., and Pajdla, T. (2013). 3d with
kinect. In Consumer Depth Cameras for Computer
Vision, pages 3–25. Springer.
Valstar, M. F., Martinez, B., Binefa, X., and Pantic, M.
(2010). Facial point detection using boosted regres-
sion and graph models. In IEEE CVPR, pages 2729–
2736.
Yin, L., Wei, X., Longo, P., and Bhuvanesh, A. (2006). An-
alyzing facial expressions using Intensity-Variant 3D
data for human computer interaction. In IEEE ICPR,
pages 1248–1251.
Zhou, S., Chellappa, R., and Jacobs, D. (2004). Character-
ization of human faces under illumination variations
using rank, integrability, and symmetry constraints.
Computer Vision-ECCV 2004, pages 588–601.
FacePoseTrackingunderArbitraryIlluminationChanges
575