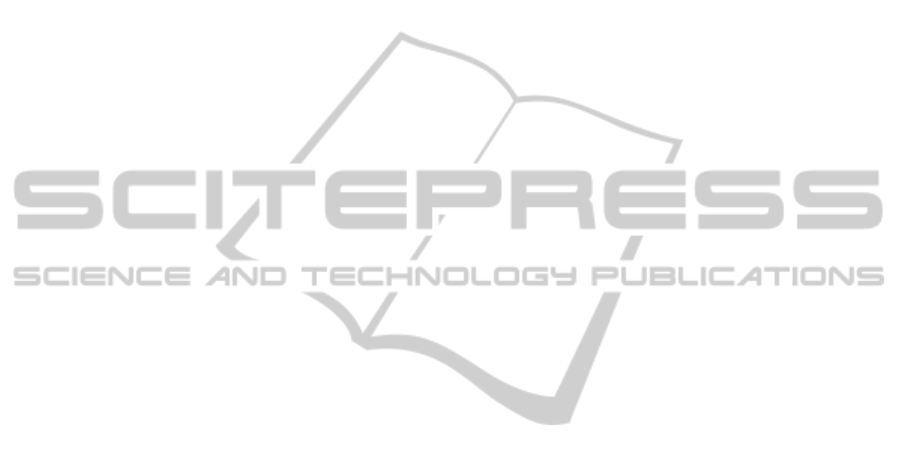
Mathematical Society Conf. Math Challenges of the
21st Century.
Doytchinova, I. A., Guan, P., and Flower, D. R. (2004).
Identifying human MHC supertypes uisng bioin-
formatics methods. The Journal of Immunology,
172:4314–4323.
Hormann, K. and Agathos, A. (2001). The point in poly-
gon problem for arbitrary polygons. Comput. Geom.
Theory Appl., 20(3):131–144.
Iwata, T., Duvenaud, D., and Ghahramani, Z. (2012).
Warped mixtures for nonparametric cluster shapes.
arXiv preprint arXiv:1206.1846.
Lampinen, J. and Oja, E. (1992). Clustering properties of
hierarchical self-organizing maps. Journal of Mathe-
matical Imaging and Vision, 2:261–272.
Larkin, J. H. and Simon, H. A. (1987). Why a diagram
is (sometimes) worth ten thousand words. Cognitive
Science, 11(1):65–100.
Lawrence, N. and Hyvrinen, A. (2005). Probabilistic
non-linear principal component analysis with gaus-
sian process latent variable models. Journal of Ma-
chine Learning Research, 6:1783–1816.
Lawrence, N. D. (2004). Gaussian process latent variable
models for visualisation of high dimensional data. In
Thrun, S., Saul, L., and Sch
¨
olkopf, B., editors, Ad-
vances in Neural Information Processing Systems 16,
pages 329–336, Cambridge, MA. MIT Press.
Lawrence, N. D. (2006). Local distance preservation in the
GPLVM through back constraints. In In ICML, pages
513–520. ACM Press.
Lawrence, N. D. (2008). Large scale learning with the gaus-
sian process latent variable model. Technical report,
university of sheffield, United Kingdom.
Lawrence, N. D., Seeger, M., and Herbrich, R. (2003).
Fast sparse gaussian process methods: The informa-
tive vector machine. In Advances in Neural Infor-
mation Processing Systems 15, pages 609–616. MIT
Press.
Lee, D., Redfern, O., and Orengo, C. (2007). Predicting
protein function from sequence and structure. Nature
Reviews Molecular Cell Biology, 8:995–1005.
Lowe, D. and Tipping, M. E. (1996). Neuroscale: Novel
topographic feature extraction using RBF networks.
In NIPS, pages 543–549.
MacQueen, J. (1967). Some methods for classification and
analysis of multivariate observations. In Le Cam,
L. M. and Neyman, J., editors, Proceedings of the 5th
Berkeley Symposium on Mathematical Statistics and
Probability - Vol. 1, pages 281–297. University of Cal-
ifornia Press, Berkeley, CA, USA.
McLachlan, G. and Basford, K. (1988). Mixture Mod-
els: Inference and Applications to Clustering. Marcel
Dekker, New York.
Miikkulainen, R. (1990). Script recognition with hierarchi-
cal feature maps. Connection Science, 2:83–101.
Mumtaz, S., Flower, D. R., and Nabney, I. T.
(2013). Multi-level visualisation. Technical re-
port, NCRG, Aston University, Birmingham, UK.
http://eprints.aston.ac.uk/.
Mumtaz, S., Nabney, I. T., and Flower, D. (2012). Novel
visualization methods for protein data. In Compu-
tational Intelligence in Bioinformatics and Computa-
tional Biology (CIBCB), 2012 IEEE Symposium on,
pages 198 –205.
Murtagh, F. and Contreras, P. (2011). Methods of hierarchi-
cal clustering. CoRR, abs/1105.0121.
Quionero-candela, J., Rasmussen, C. E., and Herbrich, R.
(2005). A unifying view of sparse approximate gaus-
sian process regression. Journal of Machine Learning
Research, 6:1939–1959.
Schreck, T., von Landesberger, T., and Bremm, S. (2010).
Techniques for precision-based visual analysis of pro-
jected data. Information Visualization, 9(3):181–193.
Shneiderman, B. (2002). Inventing discovery tools: com-
bining information visualization with data mining. In-
formation Visualization, 1(1):5–12.
Tino, P. and Nabney, I. T. (2002). Hierarchical GTM: Con-
structing localized nonlinear projection manifolds in
a principled way. In IEEE Transactions on Pattern
Analysis and Machine Intelligence, volume 24, pages
639–656.
Tipping, M. E. and Bishop, C. M. (1999). Probabilistic prin-
cipal component analysis. Journal of the Royal Statis-
tical Society, Series B, 61:611–622.
Venna, J. and Kaski, S. (2001). Neighborhood preserva-
tion in nonlinear projection methods: An experimen-
tal study. In Proceedings of the International Con-
ference on Artificial Neural Networks, ICANN ’01,
pages 485–491, London, UK, UK. Springer-Verlag.
Versino, C. and Gambardella, L. M. (1996). Learning fine
motion by using the hierarchical extended kohonen
map. In MAP, Proceedings Proc. ICANN96, Inter-
national Conference on Artificial Neural Networks,
pages 221–226. Springer-Verlag.
Vicente, D. and Vellido, A. (2004). Review of hierarchi-
cal models for data clustering and visualization. Ten-
dencias de la Minera de Datos en Espaa, Espaola de
Minera de Datos.
Wang, X., Ti
ˇ
no, P., and Fardal, M. A. (2008). Multiple man-
ifolds learning framework based on hierarchical mix-
ture density model. In Proceedings of the European
conference on Machine Learning and Knowledge Dis-
covery in Databases - Part II, ECML PKDD ’08,
pages 566–581, Berlin, Heidelberg. Springer-Verlag.
Multi-levelVisualisationusingGaussianProcessLatentVariableModels
129