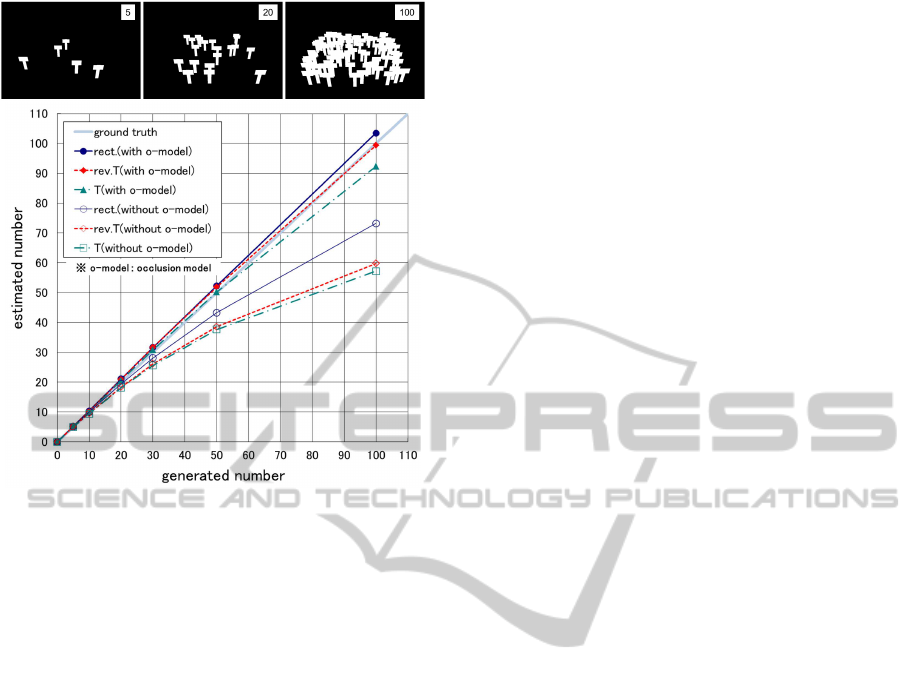
Figure 14: Estimation result in crowded situations.
the effectiveness of the proposed number estimation
algorithm for arbitrary silhouettes. Three types of sil-
houette shapes with various numbers of silhouettes
were generated, see examples in Figure 14. The hori-
zontal axis plots the actual number of silhouettes gen-
erated, and the vertical axis indicates the estimated
numbers. As can be seen, when the number of silhou-
ettes is below 20 or 30, the estimated numbers with-
out occlusion model are very close to the generated
number, the ground-truth. On the other hand, with
more than 30, the lines of estimated numbers without
occlusion model deviate from the ground-truth. Ap-
plying the occlusion model mentioned in 4.2 makes
the estimated numbers approach the ground-truth.
6 CONCLUSIONS
In this paper, we extended the basic theory of surface
area analysis by introducing two improved techniques
to better estimate the number of objects in images.
We extended the basic theory to be able to deal with
the directly downward capture case and with arbitrary
silhouettes. The slant silhouette analysis realizes re-
liable estimation even if the object is directly under a
camera. The silhouette decomposition technique ex-
tends object shape from a simple rectangle to arbitrary
silhouettes. Experiments showed the validity of the
proposed theory and the effectiveness of our crowd
estimation technique.
REFERENCES
Antonini, G. and Thiran, J. (2006). Counting pedestrians
in video sequences using trajectory clustering. IEEE
Transactions on Circuits and Systems for Video Tech-
nology, 16(issue 8):1008–1020.
Arai, H., Miyagawa, I., Koike, H., and Haseyama, M.
(2009). Estimating number of people using calibrated
monocular camera based on geometrical analysis of
surface area. IEICE Transactions, 92-A(8):1932–
1938.
Cho, S.-Y., Chow, T., and Leung, C.-T. (1999). A neural-
based crowd estimation by hybrid global learning al-
gorithm. IEEE Transactions on Systems, Man, and
Cybernetics, 29(PartB)(issue 4):535–541.
Kong, D., Gray, D., and Tao, H. (2006). A viewpoint in-
variant approach for crowd counting. Proceedings of
the 18th International Conference on Pattern Recog-
nition, 03:1187–1190.
Marana, A., Costa, L., Lotufo, R., and Velastin, S. (1998).
On the efficacy of texture analysis for crowd monitor-
ing. Proceedings of the International Symposium on
Computer Graphics, Image Processing, 6:3521–3524.
Min, L., Zhaoxiang, Z., Kaiqi, H., and Tieniu, T. (2008).
Estimating the number of people in crowded scenes
by mid based foreground segmentation and head-
shoulder detection. International Conference on Pat-
tern Recognition(ICPR 2008), pages 1–4.
Rabaud, V. and Belongie, S. (2006). Counting crowded
moving objects. Proceedings of the 2006 IEEE Com-
puter Society Conference on Computer Vision and
Pattern Recognition, 1:705–711.
Sheng-Fuu Lin, J.-Y. C. and Chao, H.-X. (2001). Estima-
tion of number of people in crowded scenes using per-
spective transformation. IEEE Transactions on Man
and Cybernetics, 31(PartA)(Issue 6):645–654.
Sidla, O., Lypetskyy, Y., Brandle, N., and Seer, S. (2006).
Pedestrian detection and tracking for counting appli-
cations in crowded situations. Proceedings of the
IEEE International Conference on Video and Signal
Based Surveillance(AVSS06), pages 70–.
Wen, Q., JIA, C., Yu, Y., Chen, G., Yu, Z., and Zhou, C.
(2011). People number estimation in the crowded
scenes using texture analysis based on gabor fil-
ter. Journal of Computational Information Systems,
7:11:3754–3763.
Zhao, T., Nevatia, R., and Wu, B. (2007). Segmentation
and tracking of multiple humans in crowded environ-
ments. IEEE Transactions on Pattern Analysis and
Machine Intelligence, 30(7):1198–1211.
SurfaceAreaAnalysisforPeopleNumberEstimation
93