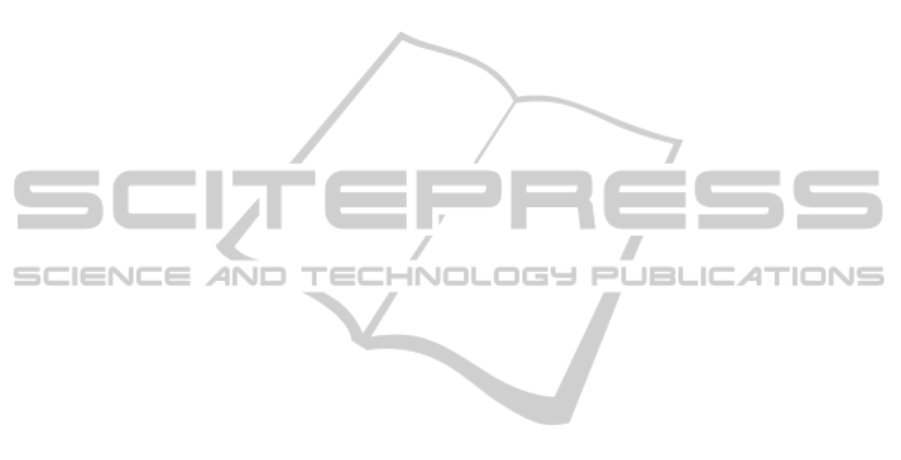
matching methods have several applications such as
2D and 3D image analysis, biometrics, biomedical
and biological frameworks, etc (Conte et al., 2004).
1.1 Literature Review
There are in the literature previous versions of the
watershed from propagated markers include the one
based on binding of markers (Flores and Lotufo,
2010a), spatial temporal gradient (Flores and Lotufo,
2010b), and border tracking by graph matching (Or-
toncelli and Flores, 2013).
In (Flores and Lotufo, 2010a) binding of markers
created around the border of the segmentation mask
were propagated by the Lucas and Kanade optical
flow computation method (Beauchemin and Barron,
1995) in orther to segment the next frame, the same
method is used to do the propagation in (Flores and
Lotufo, 2010b), but this watershed from propagated
markers variation also uses a spatio-temporal gradi-
ent to segment the images. This gradient is computed
with a 3-D structuring element.
Another version of the watershed from propagated
markers is based on graph matching (Ortoncelli and
Flores, 2013). In this version, graph matching is ap-
plied to propagate markers from the previous frame
to the current one. A model graph represents markers
in the previus frames, and a frame graph represents
the hierarchical segmentation of current frame. An
energy function is used to match each edge of model
graph with one edge of the frame graph, the result of
this matching it is used as markers to segment the ob-
ject of interest in the current frame.
In the literature, graph matching is also used to
segment images without the wathershed from propa-
gated markers. In (Noma et al., 2012), the user inputs
markers that will be used to create a model graph.
Then, a graph matching method is used to compute
the correspondence between this graph and another
one based on the image regions, the result of this
matching is the segmented image. In (Noma et al.,
2012) also is presented a semi-automatic variation of
the proposed method, in wich a image sequence is
segmented with the same set of markers computing
the matching for each image of the sequence.
The seeded region growing (SRG) algorithm
(Adams and Bischof, 1994) is similar to graph match-
ing methods in the context of image segmentation.
The SRG algorithm consists in a sequential labelling
technique, in which each iteraction labels only one
pixel that neighbours the already labelled pixels and
the aggregation criterion is given by a dissimilarity
measure (in graph matching methods the all the re-
gions are labelled in only one iteraction). In the liter-
ature there are automatic (Fan et al., 2001) and semi-
automatic (Zhi and Jie, 2004) methods that extends
SRG algorithm from the pixel level for the regional
one in order to segment image sequences. In (Zhi
and Jie, 2004) it is also proposed a method variation
in which is possible correct interactively the segmen-
tation errors.
1.2 Contributions
Despite the good segmentation results providedby the
support of graph matching techniques, The interac-
tive correction of errors and resegmentation due the
mispropagation of the markers (or in the segmenta-
tion) may be costly, because graph matching tech-
niques are sometimes heavy tasks, the interactive ap-
proach would contradict one of the desirable proper-
ties of the interactive segmentation methods: rapid re-
sponse (Flores and Lotufo, 2010a).
To solve this problem the combination between
watershed from propagated markers and graph match-
ing is a good alternative, that is comproved in (Orton-
celli and Flores, 2013). In this paper it was proposed a
new way to combine these methods. Given the mask
of segmentation from the previous frame, the model
graph is computed from this mask, and this model is
matched (with a graph matching method (Noma et al.,
2012)) to the previously computed graph (that rep-
resents the hierarchical segmentation of the current
frame) in order to find a pre-segmentation mask of
the objects of interest in the current frame in order
to create the markers that will be applied to the seg-
mentation of the current frame with watershed from
markers.
This new approach is better than use only a graph
matching method, because the matching is done just
once in the beginning of the processing of the current
frame, in order to create markers. Given the com-
puted markers, watershed from markers is applied to
compute the segmentation of the objects of interest. If
there are errors in the segmentation, the user can cor-
rect then interactively, adding or removing markers,
but how this time the segmentation is done with wa-
tershed from markers, the response for the user is very
fast. Figure 1 illustrates the watershed from propa-
gated markers variation proposed in this paper.
This approach differs from the watershed from
propagated markers variation proposed in (Ortoncelli
and Flores, 2013), because in this approach it was
computed a pre-segmentation mask of the frame by
a graph matching method in order to compute de
markers, and in (Ortoncelli and Flores, 2013) a graph
matching method is used to directly propagate the
markers. This new approach showed more efficient
WatershedfromPropagatedMarkersbasedonMorphologicalHierarchicalSegmentationandGraphMatching
321