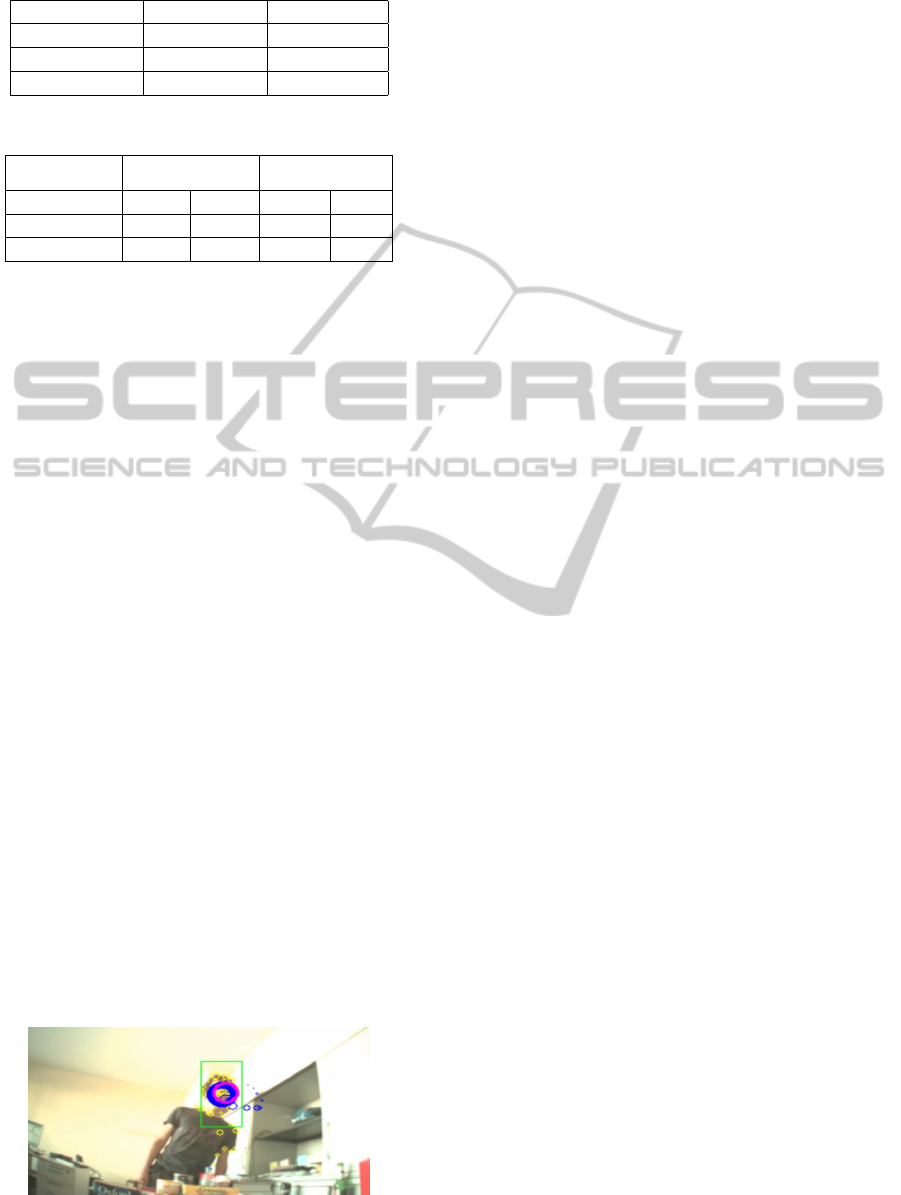
Table 2: Performance evaluation : No occlusion scenario.
No learning Full system
Displacement 53% 73%
Distraction 86% 93%
Merging 0% 80%
Table 3: Performance evaluation : Occlusion scenario.
No distractor
learning
Full system
Occlusions Mask Hide Mask Hide
Momentary 90% 66% 100% 96%
Long-term 63% 60% 90% 73%
updating the object model when it is occluded.
This is even more evident in the case of long-term
occlusions. We also observe that the performance
for masking is better than hiding because of object
perspective change, camera jitter etc. Any mistake in
the distractor identification would harm the system
more in the case of long term occlusions.
Searching. In the end, we also test the searching per-
formance when the object is lost. We count how many
times the object was discovered in less than 30 sec-
onds. We observed that the searching was successful
in 73% of the trials (15 in all).
The tracker performance recorded as a video
can be viewed at https://fias.uni-frankfurt.de/neuro/
triesch/videos/icub/tracking/.
6 CONCLUSIONS
We developed and demonstrated an active stereo-
vision based tracking system on the robot. In con-
trast to other systems in the literature, our system at-
tempts to identify unusual events in the scene and take
necessary actions. The system is also generic enough
to be applied for applications such as face/pedestrian
tracking on static/active cameras (an instance of face
tracking is shown in Fig. 13). There is a scope for fur-
ther improvement in every part of our system. How-
ever, the present system clearly demonstrates how ob-
ject and distractor models allow to recognize difficult
Figure 13: An instant of face tracking.
tracking situations and to respond to them appropri-
ately. This brings us one step closer to building track-
ing systems with human level performance.
ACKNOWLEDGEMENTS
This work was supported by the BMBF Project
“Bernstein Fokus: Neurotechnologie Frankfurt, FKZ
01GQ0840”. We thank S
´
ebastien Forestier for the
participation and help with the experiments.
REFERENCES
Chandrashekhariah, P., Spina, G., and Triesch, J. (2013).
Let it learn: A curious vision system for autonomous
object learning. In VISAPP.
Erdem, C., Sankur, B., and Tekalp, A. (2004). Performance
measures for video object segmentation and tracking.
Image Processing, IEEE Transactions on, 13(7):937–
951.
Falotico, E. and Laschi, C. (2009). Predictive tracking
across occlusions in the icub robot. In Humanoid
Robots, 2009, pages 486–491.
Ginhoux, R. and Gutmann, S. (2001). Model-based object
tracking using stereo vision. In ICRA, Volume: 2, cole
Nationale Suprieure de Physique de.
Kalal, Z., Mikolajczyk, K., and Matas, J. (2012). Tracking-
learning-detection. Pattern Analysis and Machine In-
telligence, IEEE Transactions on, 34(7):1409–1422.
Nelson, R. C. and Green, I. A. (2002). Tracking objects
using recognition. In In International Conference on
Pattern Recogntion, pages 1025–1030. Prentice Hall.
Pattacini, U. (2010). Modular Cartesian Controllers for
Humanoid Robots: Design and Implementation on the
iCub. Ph.D. dissertation, RBCS, IIT, Genova.
Rosten, E. and Drummond, T. (2006). Machine learning for
high-speed corner detection. In ECCV, pages 430–
443.
Ta, D., Chen, W., Gelfand, N., and Pulli, K. (2009). Surf-
trac: Efficient tracking and continuous object recogni-
tion using local feature descriptors. In CVPR09.
Triesch, J., Ballard, D. H., and Jacobs, R. A. (2002). Fast
temporal dynamics of visual cue integration. Percep-
tion, 31(4):421–434.
Wiskott, L., Fellous, J., Kr
¨
uger, N., and v.d. Malsburg,
C. (1997). Face recognition by elastic bunch graph
matching. IEEE Trans. Pattern Anal. Mach. Intell.
Yin, F., Makris, D., and Velastin, S. A. (2007). Perfor-
mance Evaluation of Object Tracking Algorithms. In
PETS2007.
HideandSeek-AnActiveBinocularObjectTrackingSystem
591