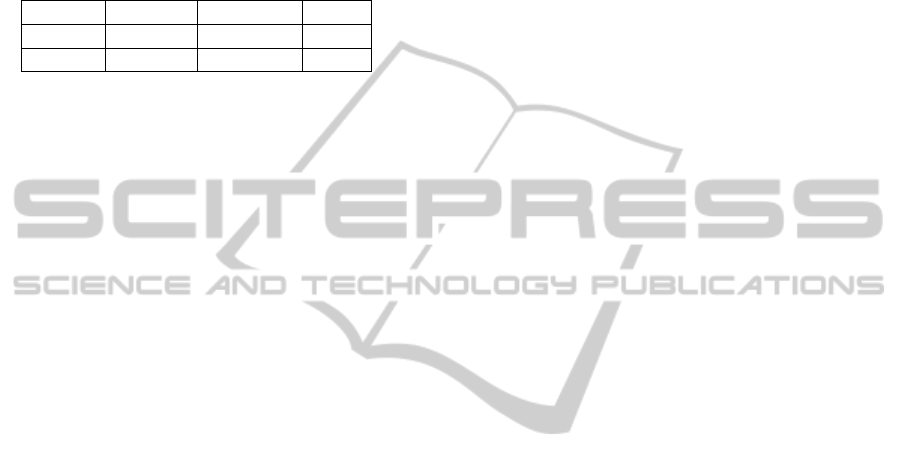
results for printed papers is more muted. This is pos-
sibly because while much of the 3D structure of the
substrate is obliterated by the laser toner its interac-
tion with the substrate itself (which is evident equally
in 2D and 3D) still provides a useful key for classifi-
cation.
Table 3: Classification results with 3D and 2D information
for dataset 2.
Feature Training Validation Test
3D 91.72 95.4 92.55
2D 92.14 92.75 89.36
6 CONCLUSIONS
The 3D surface texture presented in this paper shows
promising attributes for a measure to be used for ac-
curate and robust classification of paper materials.
We presented an ultra-high resolution image sensing
device adapted to capture photometric images at a
micro-scale level. Using a 4-light source PS approach
we have demonstrated that we can recover 3D micro-
geometry for different types of paper substrates. Con-
sequently, we show that features extracted from the
recovered 3D micro-geometry can be used to charac-
terise and classify different categories of paper types
at significantly high-level of accuracy and easily out-
performs classification based on features extract from
2D surface information. Additionally the steps in-
volved in deriving the 3D signature have low com-
putational complexity and more importantly is very
cost-effective. We believe that a system based on the
model presented in this paper can have wide use in
several industries where document forgery is a con-
siderable threat.
REFERENCES
Adams, G. (2010). Hand held dyson relay lens for anti-
counterfeiting. In Imaging Systems and Techniques
(IST), 2010 IEEE International Conference on, pages
273–278. IEEE.
Barsky, S. and Petrou, M. (2003). The 4-source photomet-
ric stereo technique for three-dimensional surfaces in
the presence of highlights and shadows. Pattern Anal-
ysis and Machine Intelligence, IEEE Transactions on,
25(10):1239–1252.
Buchanan, J. D. R., Cowburn, R. P., Jausovec, A.-V., Pe-
tit, D., Seem, P., Xiong, G., Atkinson, D., Fenton, K.,
Allwood, D. A., and Bryan, M. T. (2005). Forgery:
‘fingerprinting’ documents and packaging. Nature,
436(7050):475.
Chantler, M., Petrou, M., Penirsche, A., Schmidt, M., and
MGunnigle, G. (2005). Classifying surface texture
while simultaneously estimating illumination direc-
tion. International Journal of Computer Vision, 62(1-
2):83–96.
Chiang, P.-J., Khanna, N., Mikkilineni, A., Segovia, M.
V. O., Suh, S., Allebach, J., Chiu, G., and Delp, E.
(2009). Printer and scanner forensics. Signal Process-
ing Magazine, IEEE, 26(2):72–83.
Clarkson, W., Weyrich, T., Finkelstein, A., Heninger, N.,
Halderman, J. A., and Felten, E. W. (2009). Finger-
printing blank paper using commodity scanners. In
Security and Privacy, 2009 30th IEEE Symposium on,
pages 301–314. IEEE.
Cula, O. G. and Dana, K. J. (2001). Recognition methods
for 3d textured surfaces. In Proceedings of SPIE con-
ference on human vision and electronic imaging VI,
volume 4299, pages 209–220.
Frankot, R. T. and Chellappa, R. (1988). A method for
enforcing integrability in shape from shading algo-
rithms. Pattern Analysis and Machine Intelligence,
IEEE Transactions on, 10(4):439–451.
Haralick, R. M., Shanmugam, K., and Dinstein, I. H.
(1973). Textural features for image classification. Sys-
tems, Man and Cybernetics, IEEE Transactions on,
(6):610–621.
Johnson, M. K., Cole, F., Raj, A., Adelson, E. H., et al.
(2011). Microgeometry capture using an elastomeric
sensor. ACM Trans. Graph., 30(4):46.
Khanna, N. and Delp, E. J. (2010). Intrinsic signatures for
scanned documents forensics: effect of font shape and
size. In Circuits and Systems (ISCAS), Proceedings of
2010 IEEE International Symposium on, pages 3060–
3063. IEEE.
Koenderink, J. J. and van Doorn, A. J. (1992). Surface shape
and curvature scales. Image and vision computing,
10(8):557–564.
Kuparinen, T., Kyrki, V., Mielik
¨
ainen, J., and Toivanen, P. J.
(2007). Paper surface topography using three-light
photometric stereo. In MVA, pages 45–48. Citeseer.
Marquardt, D. W. (1963). An algorithm for least-squares es-
timation of nonlinear parameters. Journal of the Soci-
ety for Industrial & Applied Mathematics, 11(2):431–
441.
McDaniel, T. and Panchanathan, S. (2007). Perceptual sur-
face roughness classification of 3d textures using sup-
port vector machines. In Haptic, Audio and Visual
Environments and Games, 2007. HAVE 2007. IEEE
International Workshop on, pages 154–159. IEEE.
Simske, S. J. and Adams, G. (2010). High-resolution glyph-
inspection based security system. In Acoustics Speech
and Signal Processing (ICASSP), 2010 IEEE Interna-
tional Conference on, pages 1794–1797. IEEE.
Woodham, R. J. (1980). Photometric method for determin-
ing surface orientation from multiple images. Optical
engineering, 19(1):191139–191139.
PaperSubstrateClassificationbasedon3DSurfaceMicro-geometry
453