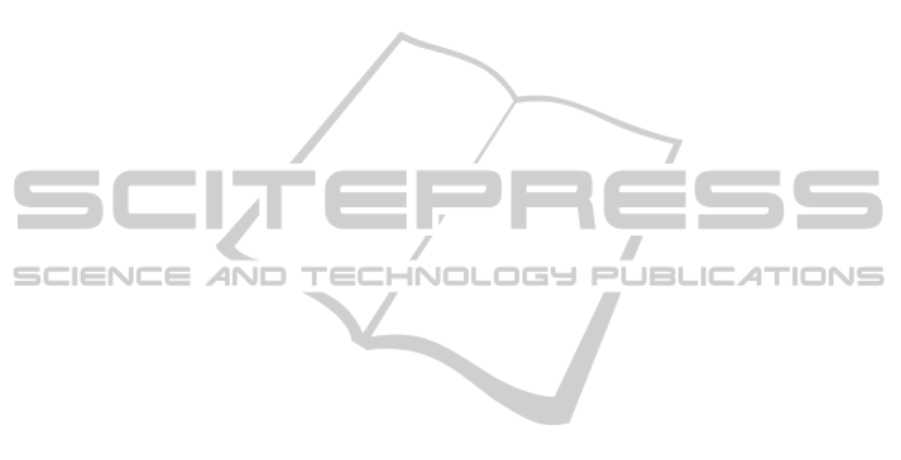
datasets in this tradition include the Face Recognition
Grand Challenge (FRGC) (Phillips et al., 2005) and
Multi-PIE (Gross et al., 2010) datasets. Approaches
based on synthetic data have fallen out of favor in re-
cent years. While synthetic tests clearly have limi-
tations: the variability within the class of synthetic
faces does not approach that of natural faces. Tests
with synthetic data also have numerous advantages.
In particular, appearance transformations can be spec-
ified with a level of detail that could neverbe obtained
in a dataset of natural photographs. Very large syn-
thetic datasets can be created with no extra cost, in the
case of the SUFR challenge, it was simple to include
tests that address interaction effects between transfor-
mations. This could not have been done in a set of
natural photographs without a costly investment.
We advocate an approach that combines tests on
unconstrained natural image datasets like Labeled
Faces in the Wild with detailed testing of particular
subtasks. However, the results presented here, and
(much more so) the work of Chen et al. (2013)—the
creators of the current (95%) state-of-the-art system
for LFW—argue that LFW may simply be too easy of
a dataset to guide future progress. We suggested that
the next generation of datasets ought to focus more on
the problem of transformations. To that end, we are
making the new SUFR-W dataset, as well as the com-
plete set of synthetic datasets, available to interested
researchers.
ACKNOWLEDGEMENTS
This material is based upon work supported by the
Center for Brains, Minds, and Machines (CBMM),
funded by NSF STC award CCF-1231216.
REFERENCES
Blender.org (2013). Blender 2.6.
Braje, W., Kersten, D., Tarr, M., and Troje, N. (1998). Illu-
mination effects in face recognition. Psychobiology,
26(4):371–380.
Chan, C., Tahir, M., Kittler, J., and Pietikainen, M.
(2013). Multiscale local phase quantization for robust
component-based face recognition using kernel fusion
of multiple descriptors. IEEE Transactions on Pattern
Analysis and Machine Intelligence, 35(5):1164–1177.
Chen, D., Cao, X., Wen, F., and Sun, J. (2013). Blessing of
Dimensionality: High-dimensional Feature and Its Ef-
ficient Compression for Face Verification. In IEEE In-
ternational Conference on Computer Vision and Pat-
tern Recognition (CVPR).
Dalal, N. and Triggs, B. (2005). Histograms of oriented gra-
dients for human detection. In Computer Vision and
Pattern Recognition, 2005. CVPR 2005. IEEE Com-
puter Society Conference on, volume 1, pages 886–
893. IEEE.
DiCarlo, J., Zoccolan, D., and Rust, N. (2012). How does
the brain solve visual object recognition? Neuron,
73(3):415–434.
Felzenszwalb, P. F., Girshick, R. B., McAllester, D., and
Ramanan, D. (2010). Object detection with discrim-
inatively trained part-based models. Pattern Analy-
sis and Machine Intelligence, IEEE Transactions on,
32(9):1627–1645.
Gross, R., Matthews, I., Cohn, J., Kanade, T., and Baker,
S. (2010). Multi-pie. Image and Vision Computing,
28(5):807–813.
Grother, P., Quinn, G., and Phillips, P. (2010). Report on
the evaluation of 2d still-image face recognition algo-
rithms. NIST Interagency Report, 7709.
Guillaumin, M., Verbeek, J., and Chmid, C. (2009). Is that
you? Metric learning approaches for face identifica-
tion. In IEEE International Conference on Computer
Vision, pages 498–505, Kyoto, Japan.
Huang, G. B., Mattar, M., Berg, T., and Learned-Miller,
E. (2008). Labeled faces in the wild: A database
for studying face recognition in unconstrained envi-
ronments. In Workshop on faces in real-life images:
Detection, alignment and recognition (ECCV), Mar-
seille, Fr.
Hung, C. P., Kreiman, G., Poggio, T., and DiCarlo, J. J.
(2005). Fast Readout of Object Identity from Macaque
Inferior Temporal Cortex. Science, 310(5749):863–
866.
Hussain, S., Napol´eon, T., and Jurie, F. (2012). Face recog-
nition using local quantized patterns. In Proc. British
Machine Vision Conference (BMCV), volume 1, pages
52–61, Guildford, UK.
Leibo, J. Z., Mutch, J., Rosasco, L., Ullman, S., and Pog-
gio, T. (2010). Learning Generic Invariances in Object
Recognition: Translation and Scale. MIT-CSAIL-TR-
2010-061, CBCL-294.
Lowe, D. G. (1999). Object recognition from local scale-
invariant features. In Computer vision, 1999. The pro-
ceedings of the seventh IEEE international conference
on, volume 2, pages 1150–1157. Ieee.
Mutch, J., Knoblich, U., and Poggio, T. (2010). CNS:
a GPU-based framework for simulating cortically-
organized networks. MIT-CSAIL-TR, 2010-013(286).
Ojala, T., Pietikainen, M., and Maenpaa, T. (2002). Mul-
tiresolution gray-scale and rotation invariant texture
classification with local binary patterns. Pattern Anal-
ysis and Machine Intelligence, IEEE Transactions on,
24(7):971–987.
Ojansivu, V. and Heikkil¨a, J. (2008). Blur insensitive
texture classification using local phase quantization.
In Image and Signal Processing, pages 236–243.
Springer.
Phillips, P. J., Flynn, P. J., Scruggs, T., Bowyer, K. W.,
Chang, J., Hoffman, K., Marques, J., Min, J., and
Worek, W. (2005). Overview of the face recogni-
tion grand challenge. In Computer vision and pattern
recognition, 2005. CVPR 2005. IEEE computer soci-
ety conference on, volume 1, pages 947–954. IEEE.
VISAPP2014-InternationalConferenceonComputerVisionTheoryandApplications
120