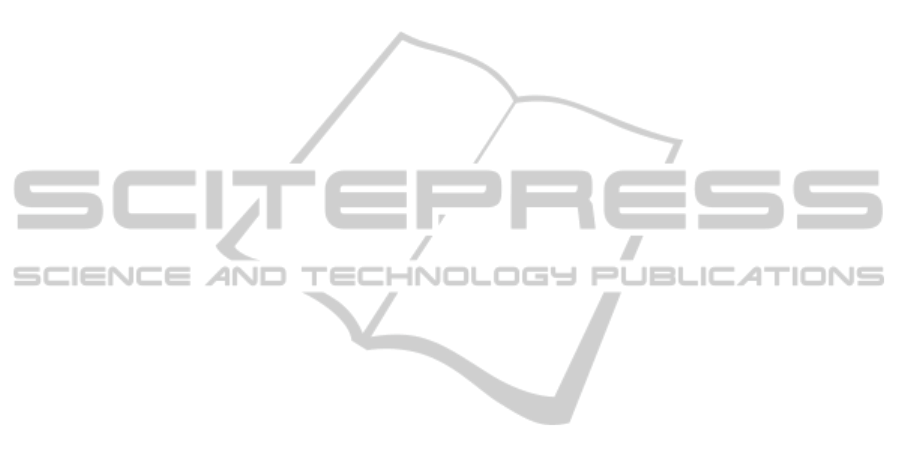
used. They can not be detected by the appliance de-
tection. An increased use of saving bulbs could lead
to future problems of activity recognition, because
the recognized activities of the two studies often in-
clude lamps. Furthermore, when at the beginning and
at the end of the day an appliance is switched (e.g.
“aquarium lighting”) the presented algorithm for ac-
tivity recognition would identify the various activities
during the day as one activity. This case did not oc-
cur in the two studies. One approach to solve this
could be a maximum time duration that a valid activ-
ity can have. Some recognized activities can be easily
determined by specifying significant appliances (e.g.
activity “breakfast” often contains appliance “toaster”
and “kettle”). But other activities that are not previ-
ously known or are very individual turn out to be dif-
ficult to detect (e.g. “afternoon nap”). The presented
approach is able to recognize such activities in an un-
supervised kind of way. In future works, we plan to
investigate, if the number of days with daily activi-
ties can be increased by recognizing larger transpo-
sitions of elements in sequences instead of only ad-
jacent transpositions. Furthermore, it will be inves-
tigated, how stand-alone running appliances, e.g., re-
frigerators, can be detected automatically.
REFERENCES
Barger, T. S., Brown, D. E., and Alwan, M. (2005). Health-
status monitoring through analysis of behavioral pat-
terns. Trans. Sys. Man Cyber. Part A, 35(1):22–27.
Chen, J., Zhang, J., Kam, A., and Shue, L. (2005). An auto-
matic acoustic bathroom monitoring system. In Inter-
national Symposium on Circuits and Systems (ISCAS
2005), pages 1750–1753 Vol. 2. IEEE.
Chen, L., Hoey, J., Nugent, C., Cook, D., and Yu, Z. (2012).
Sensor-based activity recognition. IEEE Transactions
on Systems, Man, and Cybernetics, Part C, 42(6):790
–808.
Cover, T. and Hart, P. (1967). Nearest neighbor pattern clas-
sification. IEEE Transactions on Information Theory,
13(1):21 –27.
Guralnik, V. and Haigh, K. Z. (2002). Learning mod-
els of human behaviour with sequential patterns. In
Proceedings of the AAAI-02 workshop Automation as
Caregiver, pages 24–30. AAAI Press.
Hart, G. (1992). Nonintrusive appliance load monitoring.
Proceedings of the IEEE, 80(12):1870–1891.
Kasteren, T. v., Noulas, A., Englebienne, G., and Kr
¨
ose, B.
(2008). Accurate activity recognition in a home set-
ting. In Proceedings of the 10th international confer-
ence on Ubiquitous computing, pages 1–9. ACM.
Katz, S. (1983). Assessing self-maintenance: activities of
daily living, mobility, and instrumental activities of
daily living. Journal of the American Geriatrics Soci-
ety, 31(12):721–727.
Katz, S., Moskowitz, R., Ba, J., and Jaffe, M. (1963). Stud-
ies of illness in the aged: The index of adl: a stan-
dardized measure of biological and psychosocial func-
tion. Journal of the American Medical Association,
185(12):914–919.
Levenshtein, V. I. (1966). Binary Codes Capable of Cor-
recting Deletions, Insertions and Reversals. Soviet
Physics Doklady, 10:707–710.
Magnetics (2013). CR Magnetics CRD5110.
http://www.crmagnetics.com/Products/CRD5100-
Series-P30.aspx. Accessed: 2013-07-28.
Mitchell, T. M. (1997). Machine Learning. McGraw-Hill,
Inc., New York, NY, USA, 1 edition.
Moeslund, T. B., Hilton, A., and Kr
¨
uger, V. (2006). A
survey of advances in vision-based human motion
capture and analysis. Comput. Vis. Image Underst.,
104(2):90–126.
Nguyen, N. T., Phung, D. Q., Venkatesh, S., and Bui, H.
(2005). Learning and detecting activities from move-
ment trajectories using the hierarchical hidden markov
models. In In Proceedings of IEEE International Con-
ference on Computer Vision and Pattern Recognition,
pages 955–960. IEEE Computer Society.
Ni Scanaill, C., Carew, S., Barralon, P., Noury, N., Lyons,
D., and Lyons, G. M. (2006). A review of approaches
to mobility telemonitoring of the elderly in their liv-
ing environment. Annals of Biomedical Engineering,
34(4):547–563.
Noury, N., Berenguer, M., Teyssier, H., Bouzid, M. J., and
Giordani, M. (2011). Building an index of activity of
inhabitants from their activity on the residential elec-
trical power line. IEEE Transactions on Information
Technology in Biomedicine, 15(5):758–766.
Oliver, N., Horvitz, E., and Garg, A. (2002). Layered rep-
resentations for human activity recognition. In Pro-
ceedings of the 4th IEEE International Conference on
Multimodal Interfaces, page 3. IEEE Computer Soci-
ety.
Oommen, B. J. (1997). Pattern recognition of strings
with substitutions, insertions, deletions and general-
ized transpositions. Pattern Recognition, 30(5):789–
800.
Philipose, M., Fishkin, K., Perkowitz, M., Patterson, D.,
Fox, D., Kautz, H., and Hahnel, D. (2004). Inferring
activities from interactions with objects. IEEE Perva-
sive Computing, 3(4):50–57.
Poppe, R. (2010). A survey on vision-based human action
recognition. Image Vision Comput., 28(6):976–990.
Quinlan, J. R. (1993). C4.5: programs for machine learn-
ing. Morgan Kaufmann Publishers Inc., San Fran-
cisco, CA, USA.
Virone, G., Alwan, M., Dalal, S., Kell, S. W., Turner, B.,
Stankovic, J. A., and Felder, R. A. (2008). Behavioral
patterns of older adults in assisted living. IEEE Trans-
actions on Information Technology in Biomedicine,
12(3):387–398.
PECCS2014-InternationalConferenceonPervasiveandEmbeddedComputingandCommunicationSystems
48