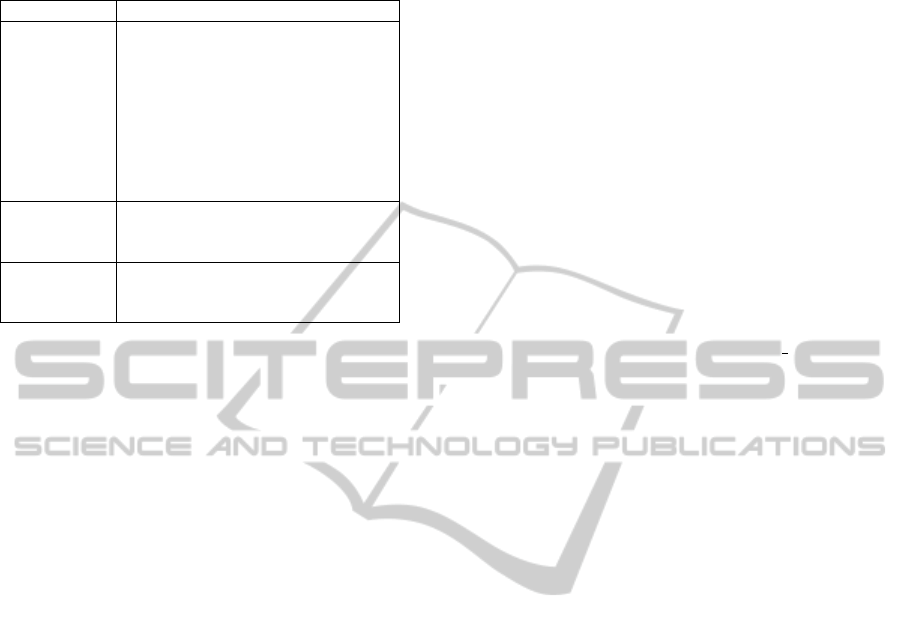
Table 2: Results on the competition dataset in terms of
Kappa values. The mean accuracy is included at the bot-
tom.
Subject GLS GLS + Mutual Information
C-1 0.754 0.763
C-2 0.410 0.419
C-3 0.800 0.805
C-4 0.484 0.475
C-5 0.243 0.257
C-6 0.317 0.364
C-7 0.629 0.758
C-8 0.661 0.707
C-9 0.698 0.721
Mean Kappa 0.555 0.586
± ±
0.19 0.20
Mean Acc 0.666 0.689
± ±
0.15 0.15
graphs by using the mutual information among the
different channels. This new strategy for building the
graph also has an impact on the filter design, allowing
an automatic way to weight the contribution of the
different spatial locations.
Comparing the mutual information matrices from
different subjects we can observe how the initial static
graph approach, where the surrounding electrodes
were linked together, was appropriate as close elec-
trodes tend to share similar information.
After applying the proposed methodology on the
two data sets Kappa value was increased for an 80%
percent of the subjects obtaining for several subjects
an improvement of 0.1 in their Kappa value.
The present study is also an example of the possi-
bilities offered by the lifting transform, where MRA
approaches can be easily implemented without the in-
herent complexity of the first generation wavelets.
The positive results presented hereby encourage
us to explore new ways for optimising the graph rep-
resentation of EEG data. Although mutual infor-
mation has helped to improve the classification rate,
other techniques such as Granger causality or phase
synchronisation indexes, which are more robust when
coping with non-stationarity, should be examined in
future work.
ACKNOWLEDGEMENTS
The first author would like to thank the EPSRC for
funding his Ph.D. study via an EPSRC DTA award.
REFERENCES
Allison, B., Graimann, B., and Grser, A. (2008). Why use a
BCI if you are healthy. Proceedings of BRAINPLAY,
playing with your brain.
Asensio-Cubero, J., Gan, J. Q., and Palaniappan, R. (2013).
Multiresolution analysis over simple graphs for brain
computer interfaces. Journal of Neural Engineering,
10(4):046014.
Blankertz, B., Muller, K. R., Krusienski, D. J., Schalk, G.,
Wolpaw, J. R., Schlogl, A., Pfurtscheller, G., Millan,
J. R., Schroder, M., and Birbaumer, N. (2006). The
BCI competition III: validating alternative approaches
to actual BCI problems. IEEE Transactions on Neural
Systems and Rehabilitation Engineering, 14(2):153–
159.
Brunner, C., Leeb, R., Muller-Putz, G. R.,
Schlogl, A., and Pfurtscheller, G. (2008).
BCI competition 2008 - Graz data set A.
http://www.bbci.de/competition/iv/desc 2a.pdf.
Carrera-Leon, O., Ramirez, J. M., Alarcon-Aquino, V.,
Baker, M., D’Croz-Baron, D., and Gomez-Gil, P.
(2012). A motor imagery bci experiment using
wavelet analysis and spatial patterns feature extrac-
tion. In Engineering Applications (WEA), 2012 Work-
shop on, pages 1–6. IEEE.
Claypoole Jr, R. L., Baraniuk, R. G., and Nowak, R. D.
(1998). Adaptive wavelet transforms via lifting. In
Proceedings of IEEE International Conference on
Acoustics, Speech and Signal Processing, volume 3,
pages 1513–1516.
Cohen, J. (1960). A coefficient of agreement for nominal
scales. Educational and Psychological Measurement,
20(1):37–46.
Cover, T. M. and Thomas, J. A. (2012). Elements of Infor-
mation Theory. John Wiley & Sons.
Daubechies, I. (2006). Ten Lectures on Wavelets. Society
for Industrial and Applied Mathematics.
Dornhege, G. (2007). Toward Brain-Computer Interfacing.
The MIT Press.
Mallat, S. G. (1989). A theory for multiresolution sig-
nal decomposition: The wavelet representation. IEEE
Transactions on Pattern Analysis and Machine Intel-
ligence., 11(7):674–693.
Martinez-Enriquez, E. and Ortega, A. (2011). Lifting trans-
forms on graphs for video coding. In Data Compres-
sion Conference, pages 73–82. IEEE.
Narang, S. K. and Ortega, A. (2009). Lifting based wavelet
transforms on graphs. In Conference of Asia-Pacific
Signal and Information Processing Association, pages
441–444.
Peng, H., Long, F., and Ding, C. (2005). Feature se-
lection based on mutual information criteria of max-
dependency, max-relevance, and min-redundancy.
Pattern Analysis and Machine Intelligence, IEEE
Transactions on, 27(8):1226–1238.
Perseh, B. and Sharafat, A. R. (2012). An efficient p300-
based bci using wavelet features and ibpso-based
channel selection. Journal of Medical Signals and
Sensors, 2(3):128.
MultiresolutionAnalysisofanInformationbasedEEGGraphRepresentationforMotorImageryBrainComputerInterfaces
11