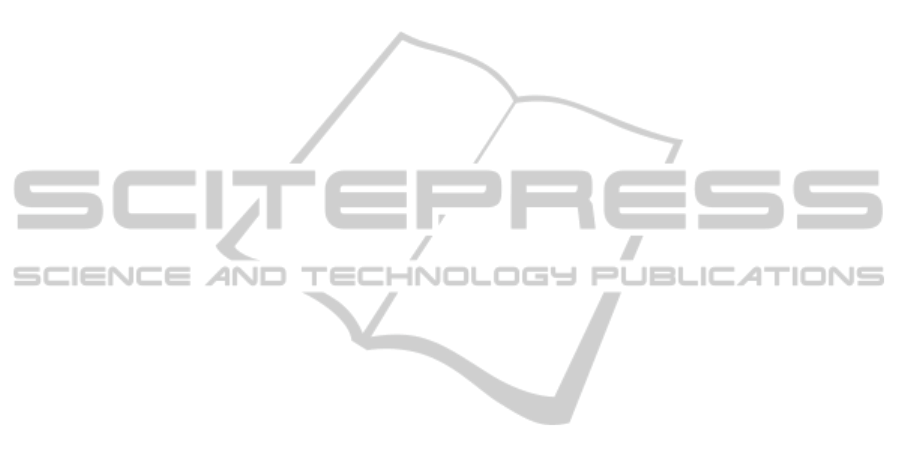
compare the vectors computed for the subsequence
to the left and the subsequence to the right of the
pointer could not detect the difference between two
TP matrixes. Therefore in the work we introduced a
new mathematical method to detect PCP based on
measures of similarity and difference between TP
matrixes.
The method could reveal the fusion and
insertions events in genes without any additional
information. Study of sequences with artificial
insertions/fusions and distribution of TP among
genes inside genome support the idea that not all
cases of insertions or fusions could be found using
the TP changes. Only fusions/insertions of
sequences with different TP matrixes would lead to
TP change points. We suppose that real number of
genes formed by insertions or fusions events could
be 5-7 greater than we obtained in the work. Now it
is difficult to say whether the function of the protein
was changed after these events and whether such
events led to creation of new genes and new
biological functions of the encoded proteins. Some
answers to the question could be found after the
experimental work.
REFERENCES
Altschul, S. F. et al., 1990. Basic local alignment search
tool. Journal of molecular biology, 215(3), pp.403–410.
Aroul-Selvam, R., Hubbard, T. & Sasidharan, R., 2004.
Domain insertions in protein structures. Journal of
molecular biology, 338(4), pp.633–641.
Bernaola-Galván, P. et al., 2000. Finding borders between
coding and noncoding DNA regions by an entropic
segmentation method. Physical Review Letters, 85(6),
pp.1342–1345.
Bhattacharya, P., 1994. Some aspects of change-point
analysis. In Carlstein, E., Müller, H.-G., Siegmund, D.
(eds.), Change Point Problems, IMS Lecture Notes -
Monograph Series, 23(1980), pp.28–56.
Boeckmann, B. et al., 2003. The SWISS-PROT protein
knowledgebase and its supplement TrEMBL in 2003.
Nucleic acids research, 31(1), pp.365–370.
Boys, R. J., Henderson, D. A. & Wilkinson, D. J., 2000.
Detecting homogeneous segments in DNA sequences
by using hidden Markov models. Journal of the Royal
Statistical Society: Series C (Applied Statistics), 49(2),
pp.269–285.
Braun, J. V & Müller, H.-G., 1998. Statistical methods for
DNA sequence segmentation. Statistical Science, 13(2),
pp.142–162.
Churchill, G. A., 1989. Stochastic models for heterogeneous
DNA sequences. Bulletin of mathematical biology,
51(1), pp.79–94.
Craig, C. C., 1936. On the frequency function of xy. he
Annals of Mathematical Statistics, 7(1), pp.1–15.
Deng, S. et al., 2012. Detecting the borders between coding
and non-coding DNA regions in prokaryotes based on
recursive segmentation and nucleotide doublets
statistics. BMC Genomics, 13(Suppl 8), p.S19.
Elton, R. A., 1974. Theoretical models for heterogeneity for
base composition in DNA. Journal of Theoretical
Biology, 45(2), pp.533–553.
Evans, G. E. et al., 2010. Estimating Change-Points in
Biological Sequences via the Cross-Entropy Method.
Annals of Operations Research, 189(1), pp.155–165.
Fickett, J. W., Torney, D. C. & Wolf, D. R., 1992. Base
compositional structure of genomes. Genomics, 13(4),
pp.1056–1064.
Frenkel, F. E. & Korotkov, E. V, 2008. Classification
analysis of triplet periodicity in protein-coding regions
of genes. Gene, 421(1-2), pp.52–60.
Frenkel, F. E. & Korotkov, E. V, 2009. Using triplet
periodicity of nucleotide sequences for finding potential
reading frame shifts in genes. DNA research: an
international journal for rapid publication of reports on
genes and genomes, 16(2), pp.105–14.
Hovmoller, S. & Zhou, T., 2004. Protein shape strings and
DNA sequences.
Korotkov, E. V et al., 2003. The informational concept of
searching for periodicity in symbol sequences.
Molekuliarnaia Biologiia, 37(3), pp.436–451.
Korotkov, E. V & Korotkova, M.A., 2010. Study of the
triplet periodicity phase shifts in genes. Journal of
integrative bioinformatics, 7(3).
Korotkova, M. A., Kudryashov, N. A. & Korotkov, E. V,
2011. An approach for searching insertions in bacterial
genes leading to the phase shift of triplet periodicity.
Genomics, proteomics & bioinformatics, 9(4-5),
pp.158–70.
Kullback, S., 1997. Information Theory and Statistics. S.
Kullback, ed., New York: Dover publications.
Li, W. et al., 2002. Applications of recursive segmentation
to the analysis of DNA sequences. Computers &
chemistry, 26(5), pp.491–510.
Li, W., 1997. The study of correlation structures of DNA
sequences: a critical review. Computers chemistry,
21(4), pp.257–271.
Melodelima, C., Gautier, C. & Piau, D., 2007. A markovian
approach for the prediction of mouse isochores. Journal
of Mathematical Biology, 55(3), pp.353–364.
Nicorici, D. & Astola, J., 2004. Segmentation of DNA into
Coding and Noncoding Regions Based on Recursive
Entropic Segmentation and Stop-Codon Statistics.
EURASIP Journal on Advances in Signal Processing,
2004(1), pp.81–91.
Nur, D. et al., 2009. Bayesian hidden Markov model for
DNA sequence segmentation: A prior sensitivity
analysis. Computational Statistics & Data Analysis,
53(5), pp.1873–1882.
Ogata, H. et al., 1999. KEGG: Kyoto Encyclopedia of
Genes and Genomes. Nucleic Acids Research, 27(1),
pp.29–34.
Papapetrou, P., Benson, G. & Kollios, G., 2012. Mining
poly-regions in DNA. International journal of data
mining and bioinformatics, 6(4), pp.406–28.
SearchofPossibleInsertionsinBacterialGenes
107