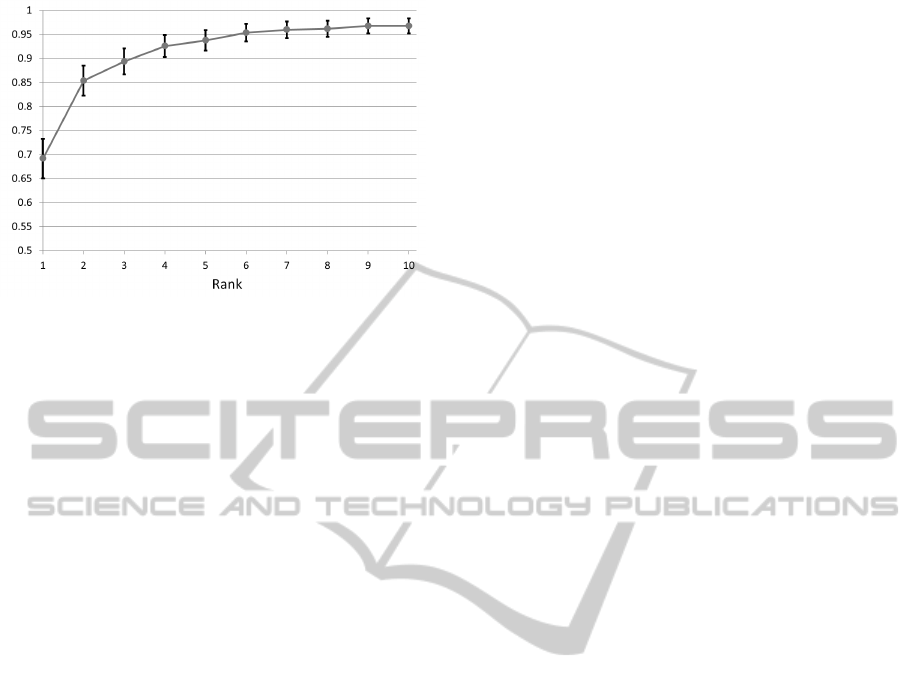
Figure 4: Rate of appropriate candidate utterance without
use of contexts.
However, as described at the beginning of this pa-
per, existing response methods cannot use contexts
for response generation. Various problems exist be-
cause of such information loss. For instance, a dia-
logue agent broaches a topic that was discussed previ-
ously or makes contradictory comments to what it had
said before. In fact, this experimentally obtained re-
sult indicates that using not only the last utterance but
also contexts are necessary for realizing superior non-
task-oriented dialogue agents. Therefore, in terms of
the availability of contexts, the effectiveness of the
statistical response method was clarified.
5 CONCLUSIONS
As described in this paper, we proposed a statistical
response method that automatically ranks previously
prepared candidate utterances in order of suitability
to the context by application of a machine learning
algorithm. Non-task-oriented dialogue agents that ap-
plied the method use the top utterance from the rank-
ing result for carrying out their dialogues. To col-
lect learning data for ranking, we used crowdsourc-
ing and gamification. We opened a gamified crowd-
sourcing website and collected learning data through
it. Thereby, we achieved low-cost and continuous
learning data acquisition. To prove the performance
of the proposed method, we checked the ranked ut-
terances to contexts and conclude that the method is
effective because a suitable utterance is ranked on the
top at 82.6% and within the top 10 at 98.6%.
The non-task-oriented dialogue agents are basi-
cally evaluated by hand work and the task requires a
tremendous amount of time and effort. By using pro-
posed gamified crowdsourcing platform, we can eval-
uate the performance of non-task-oriented dialogue
agents in a low-cost way. We prepare several types
of agents which we want to evaluate and each agent
generates a response to the given context. The plat-
form shows the context and the generated responses
to participants in the same way as our website. The re-
sponses which ware generated by a high-performance
agent should be selected more than others.
The candidate utterances are created manually.
Future work includes automatic candidate utterance
generation. Our crowdsourcing website has a func-
tion that collects new utterances. However, these ut-
terances present some problems such as spelling er-
rors, phraseology, etc. because they are written by
users in free description format. We need to fix them
to use the new utterances. As an alternative utterance
generation method, using microblog data is promis-
ing. Using microblog data, it can be expected to gen-
erate a new utterances set that includes numerous or
newest topics.
We also intend to improve the feature vector. It
is important to devise new effective features because
the performance of our method depends heavily on
the features. The features used in the experiment (not
illustrated in detail here) did not deeply consider the
semantics of contexts and utterances. Realizing ap-
propriate responses requires semantical features. We
are now deliberating on such features.
REFERENCES
Banchs, R. E. and Li, H. (2012). Iris: a chat-oriented di-
alogue system based on the vector space model. In
Proceedings of the ACL 2012 System Demonstrations,
pages 37–42. Association for Computational Linguis-
tics.
Bickmore, T. and Cassell, J. (2001). Relational agents: a
model and implementation of building user trust. In
Proceedings of the SIGCHI conference on Human fac-
tors in computing systems, pages 396–403.
Cao, Z., Qin, T., Liu, T., Tsai, M., and Li, H. (2007). Learn-
ing to rank: from pairwise approach to listwise ap-
proach. In Proceedings of the 24th international con-
ference on Machine learning, pages 129–136.
Chu-Carroll, J. and Nickerson, J. (2000). Evaluating auto-
matic dialogue strategy adaptation for a spoken dia-
logue system. In Proceedings of the 1st North Ameri-
can chapter of the Association for Computational Lin-
guistics conference, pages 202–209.
Deterding, S., Sicart, M., Nacke, L., O’Hara, K., and Dixon,
D. (2011). Gamification. using game-design elements
in non-gaming contexts. In Proceedings of the 2011
annual conference extended abstracts on Human fac-
tors in computing systems, pages 2425–2428. ACM.
Isomura, N., Toriumi, F., and Ishii, K. (2009). Statistical
Utterance Selection using Word Co-occurrence for a
Dialogue Agent. Lecture Notes in Computer Science,
5925/2009:68–79.
ICAART2014-InternationalConferenceonAgentsandArtificialIntelligence
20