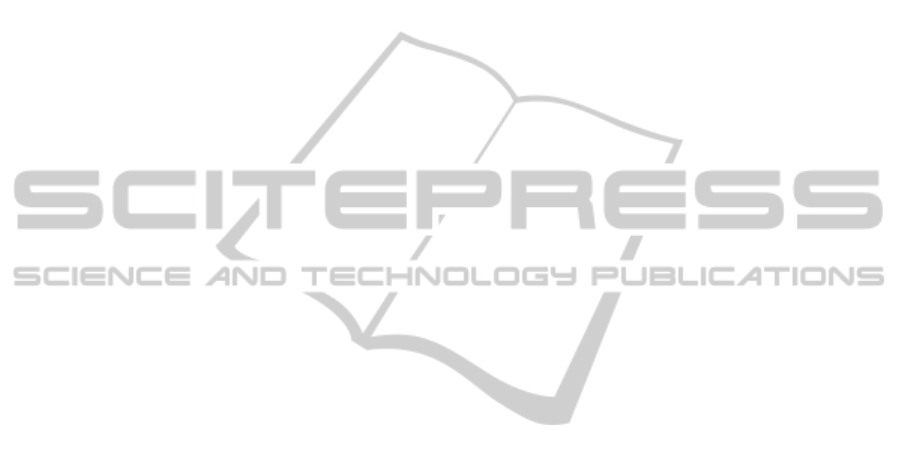
Bregler, C. 1997. Learning and recognizing human
dynamics in video sequences. IEEE Computer Society
Conference on Computer Vision and Pattern
Recognition. pp. 568-574.
Broggi, A., Bertozzi, M., Fascioli, A. & Sechi, M. 2000.
Shape-based pedestrian detection. IEEE Intelligent
Vehicles Symposium. pp. 215-220.
Dalal, N. & Triggs, B. 2005. Histograms of oriented
gradients for human detection. IEEE Computer
Society Conference on Computer Vision and Pattern
Recognition. pp. 886-893 vol. 1.
Danescu, R., Nedevschi, S., Meinecke, M. M. & Graf, T.
2007. Stereovision Based Vehicle Tracking in Urban
Traffic Environments. Intelligent Transportation
Systems Conference. pp. 400-404.
Dollar, P., Wojek, C., Schiele, B. & Perona, P. 2012.
Pedestrian Detection: An Evaluation of the State of the
Art. IEEE Transactions on Pattern Analysis and
Machine Intelligence, 34, pp. 743-761.
Elgammal, A., Duraiswami, R., Harwood, D. & Davis, L.
S. 2002. Background and foreground modeling using
nonparametric kernel density estimation for visual
surveillance. Proceedings of the IEEE, 90, pp. 1151-
1163.
Fardi, B., Schuenert, U. & Wanielik, G. 2005. Shape and
motion-based pedestrian detection in infrared images:
a multi sensor approach. IEEE Intelligent Vehicles
Symposium. pp. 18-23.
Gandhi, T. & Trivedi, M. M. 2006. Pedestrian collision
avoidance systems: a survey of computer vision based
recent studies. Intelligent Transportation Systems
Conference. pp. 976-981.
Gavrila, D. 2000. Pedestrian Detection from a Moving
Vehicle. Proceedings of the 6th European Conference
on Computer Vision-Part II, pp. 37-49.
Gavrila, D. M., Giebel, J. & Munder, S. 2004. Vision-
based pedestrian detection: the PROTECTOR system.
IEEE Intelligent Vehicles Symposium. pp. 13-18.
Gavrila, D. M. & Munder, S. 2007. Multi-cue Pedestrian
Detection and Tracking from a Moving Vehicle.
International Journal of Computer Vision, 73, pp. 41-
59.
Gavrila, D. M. & Philomin, V. 1999. Real-time object
detection for “smart” vehicles.
Proceedings of the Seventh IEEE International
Conference on Computer Vision. pp. 87-93 vol.1.
Giosan, I. & Nedevschi, S. 2009. Building Pedestrian
Contour Hierarchies for Improving Detection in
Traffic Scenes. Proceedings of the International
Conference on Computer Vision and Graphics:
Revised Papers, pp. 154-163.
Giosan, I., Nedevschi, S. & Bota, S. 2009. Real time
stereo vision based pedestrian detection using full
body contours. IEEE 5th International Conference on
Intelligent Computer Communication and Processing
.
pp. 79-86.
Haller, I. & Nedevschi, S. 2010. GPU optimization of the
SGM stereo algorithm. IEEE International
Conference on Intelligent Computer Communication
and Processing (ICCP). pp. 197-202.
Haritaoglu, I., Harwood, D. & Davis, L. S. 2000. W4:
real-time surveillance of people and their activities.
IEEE Transactions on Pattern Analysis and Machine
Intelligence, 22, pp. 809-830.
Havasi, L., Szlavik, Z. & Sziranyi, T. 2004. Pedestrian
Detection Using Derived Third-Order Symmetry of
Legs. Proceedings of the IEEE International
Conference on Computer Vision and Graphics, pp. 11-
17.
Hilario, C., Collado, J. M., Armingol, J. M. & Escalera, A.
D. L. 2005. Pedestrian detection for intelligent
vehicles based on active contour models and stereo
vision. Proceedings of the 10th international
conference on Computer Aided Systems Theory, pp.
537-542.
Javed, O. & Shah, M. 2002. Tracking and Object
Classification for Automated Surveillance.
Proceedings of the 7th European Conference on
Computer Vision-Part IV, pp. 343-357.
Keller, C. G., Enzweiler, M. & Gavrila, D. M. 2011a. A
new benchmark for stereo-based pedestrian detection.
IEEE Intelligent Vehicles Symposium (IV). pp. 691-
696.
Keller, C. G., Enzweiler, M., Rohrbach, M., Fernandez
Llorca, D., Schnorr, C. & Gavrila, D. M. 2011b. The
Benefits of Dense Stereo for Pedestrian Detection.
IEEE Transactions on Intelligent Transportation
Systems, 12, pp. 1096-1106.
Khammari, A., Nashashibi, F., Abramson, Y. & Laurgeau,
C. 2005. Vehicle detection combining gradient
analysis and AdaBoost classification. Proceedings of
Intelligent Transportation Systems. pp. 66-71.
Koller, D., Danilidis, K. & Nagel, H.-H. 1993. Model-
based object tracking in monocular image sequences
of road traffic scenes. International Journal of
Computer Vision, 10, pp. 257-281.
Lipton, A. J., Fujiyoshi, H. & Patil, R. S. 1998. Moving
target classification and tracking from real-time video.
Fourth IEEE Workshop on Applications of Computer
Vision. pp. 8-14.
Llorca, D. F., Sotelo, M. A., Hellín, A. M., Orellana, A.,
Gavilan, M., Daza, I. G. & Lorente, A. G. 2012. Stereo
regions-of-interest selection for pedestrian protection:
A survey. Transportation research part C: emerging
technologies, 25, pp. 226-237.
Lun, Z., Li, S. Z., Xiaotong, Y. & Shiming, X. 2007. Real-
time Object Classification in Video Surveillance
Based on Appearance Learning. IEEE Conference on
Computer Vision and Pattern Recognition. pp. 1-8.
Marita, T., Oniga, F., Nedevschi, S., Graf, T. & Schmidt,
R. 2006. Camera Calibration Method for Far Range
Stereovision Sensors Used in Vehicles. IEEE
Intelligent Vehicles Symposium. pp. 356-363.
Masoud, O. & Papanikolopoulos, N. P. 2001. A novel
method for tracking and counting pedestrians in real-
time using a single camera. IEEE Transactions on
Vehicular Technology, 50, pp. 1267-1278.
Nedevschi, S., Bota, S. & Tomiuc, C. 2009. Stereo-Based
Pedestrian Detection for Collision-Avoidance
Multi-featureRealTimePedestrianDetectionfromDenseStereoSORT-SGMReconstructedUrbanTrafficScenarios
141