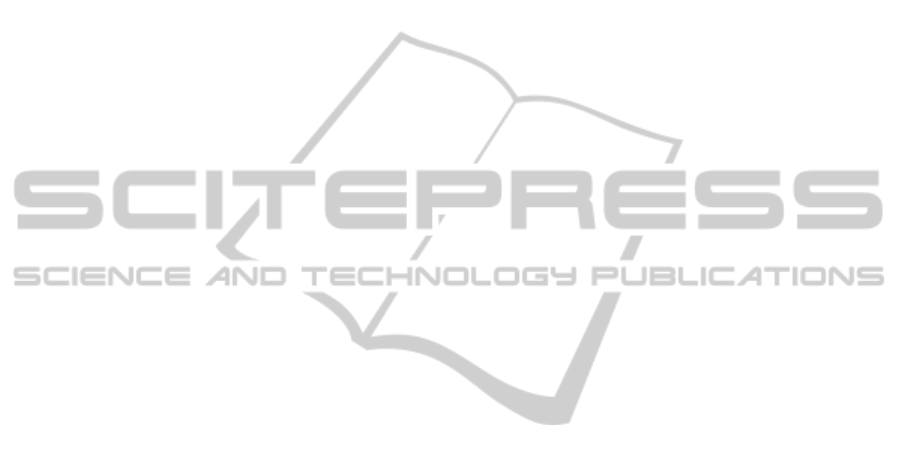
resolution images produced by monochromatic smart
sensors. The edge-based method outperformstexture-
based foreground segmentation at low resolutions. In
this work, we demonstrated that edge information be-
comes more relevant than texture information when
the image resolution scales down.
ACKNOWLEDGEMENTS
The work was financially supported by iMinds and
IWT through the Project ‘LittleSister’.
REFERENCES
Ahonen, T., Hadid, A., and Pietik¨ainen, M. (2006). Face
description with local binary patterns: application to
face recognition. IEEE Trans. on Pattern Recogn. and
Machine Intelligence, 28(12):2037–2041.
Ahonen, T. and Pietik¨ainen, M. (2009). Image description
using joint distribution of filter bank responses. Pat-
tern Recognition Letters, 30(4):368–376.
Barnich, O. and Droogenbroeck, M. V. (2009). Vibe: a pow-
erful random technique to estimate the background in
video sequences. In IEEE Int. Conf. on Acoustics,
Speech and Signal Processing, pages 945–948.
Camilli, M. and Kleihorst, R. P. (2011). Demo: Mouse sen-
sor networks, the smart camera. In ACM/IEEE Int.
Conf. on Distributed Smart Cameras, pages 1–3.
Gonzalez, R. C. and Woods, R. E. (2001). Digital Im-
age Processing. Addison-Wesley Longman Publish-
ing Co., Boston, MA, USA, 2nd edition.
Gr¨uenwedel, S., Hese, P. V., and Philips, W. (2011). An
edge-based approach for robust foreground detection.
In Proc. of Advanced Concepts for Intelligent Vision
Systems, pages 554–565.
Gr¨unwedel, S., Jelaˇca, V., Hese, P. V., Kleihorst, R., and
Philips, W. (2011). Phd forum : Multi-view occu-
pancy maps using a network of low resolution visual
sensors. In 2011 Fifth ACM/IEEE Int. Conf. on Dis-
tributed Smart Cameras. IEEE.
Gr¨unwedel, S., Jelaˇca, V., no Casta˜neda, J. N., Hese, P. V.,
Cauwelaert, D. V., Haerenborgh, D. V., Veelaert, P.,
and Philips, W. (2013). Low-complexity scalable
distributed multi-camera tracking of humans. ACM
Transactions on Sensor Networks, 10(2).
Heikkil¨a, M. and Pietik¨ainen, M. (2006). A texture-based
method for modeling the background and detecting
moving objects. IEEE Trans. on Pattern Recognition
and Machine Intelligence, 28(4):657–662.
Heikkil¨a, M., Pietik¨ainen, M., and Schmid, C. (2009). De-
tection of interest regions with local binary patterns.
Pattern Recognition, 42(3):425–436.
Hengstler, S. and Aghajan, H. (2006). A smart camera mote
architecture for distributed intelligent surveillance. In
ACM SenSys Workshop on Distributed Smart Cam-
eras.
Huang, X., Li, S., and Wang, Y. (2004). Shape localiza-
tion based on statistical method using extended local
binary pattern. In Proc. of Int. Conf. on Image and
Graphics, pages 184–187.
M¨aenp¨a¨a, T., Ojala, T., Pietik¨ainen, M., and Soriano, M.
(2000). Robust texture classification by subsets of lo-
cal binary patterns. In Proc. of Int. Conf. on Pattern
Recognition, pages 935–938.
Marr, D. and Hildreth, E. (2000). Theory of edge detection.
In Proc. of Int. Conf. on Pattern Recognition, volume
207, pages 935–938.
Movshon, J., Adelson, E. H., Gizzi, M. S., and Newsome,
W. T. (1986). The analysis of moving visual patterns.
Pattern Recognition Mechanisms, 54:117–151.
Ojala, T., Pietik¨ainen, M., and Harwood, D. (1996). A com-
parative study of texture measures with classification
based on feature distributions. Pattern Recognition,
29(1):51–59.
Ojala, T., Pietik¨ainen, M., and M¨aenp¨a¨a, T. (2002). Mul-
tiresolution gray-scale and rotation invariant texture
classification with local binary patterns. IEEE Trans.
on Pattern Recognition and Machine Intelligence,
24(7):971–987.
Pietik¨ainen, M., Nurmela, T., M¨aenp¨a¨a, T., and Turtinen,
M. (2004). View-based recognition of real-world tex-
tures. Pattern Recognition, 37(2):313–323.
Tenorth, M., , M., Bandouch, J., and Beetz, M. (2009). The
tum kitchen data set of everyday manipulation activ-
ities for motion tracking and action recognition. In
Computer Vision Workshops (ICCV Workshops), 2009
IEEE 12th International Conference on, pages 1089–
1096.
Yao, C.-H. and Chen, S.-Y. (2003). Retrieval of translated,
rotated and scaled color textures. Pattern Recognition,
36(4):913–929.
Zhang, B., Gao, Y., Zhao, S., and Liu, J. (2010). Lo-
cal derivative pattern versus local binary pattern: face
recognition with high-order local pattern descriptor.
IEEETrans.onIm. Proc., 19(2):533–544.
Zhao, S., Gao, Y., and Zhang, B. (2008). Sobel-lbp. In IEEE
Int. Conf on Image Processing, pages 2144–2147.
Zivkovic, Z. (2004). Improved adaptive gaussian mixture
model for background subtraction. In Proc. of Int.
Conf. on Pattern Recogn., pages 28–31.
VISAPP2014-InternationalConferenceonComputerVisionTheoryandApplications
346