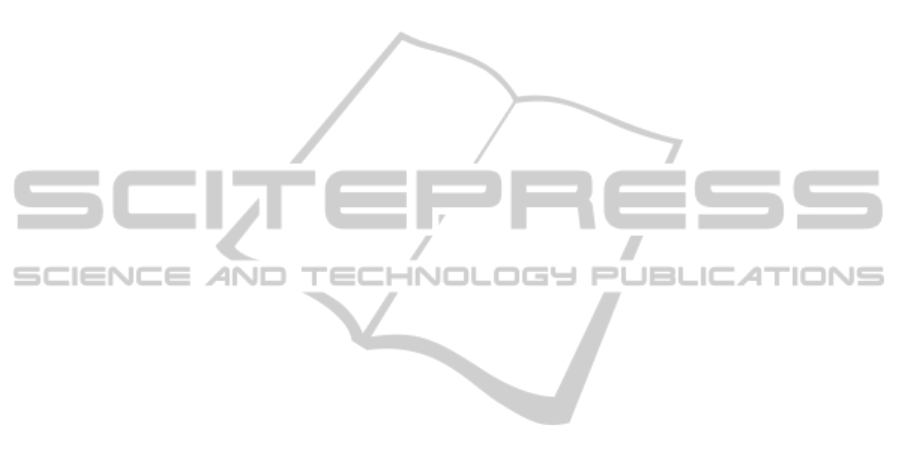
Assistive Healthcare (MoCAsH)“, 2010 IEEE Asia-
Pacific Services Computing Conference (APSCC),
Conference Proceedings, pp.325 – 332.
Huang, D., 2011, “Mobile Cloud Computing”, Multimedia
Communications Technical Committee, IEEE
Communications Society, E-Letter, Vol. 6, No. 10, pp.
1-4.
International Telecommunication Union (ITU), 2005,
“ITU Internet Reports 2005: The Internet of Things”.
Kang, B. et al. 2011, “Design of an Internet of Things-
based smart home system”, the 2nd International
Conference on Intelligent Control and Information
Processing, Conference Proceedings, pp.921-924.
Kangas, M. et al., 2007, “Determination of simple
thresholds for accelerometers for fall detection”, The
29th Annual International Conference of the IEEE
EMBS”, Conference Proceedings, p.1367-1370.
Koch, S., 2006, “Home telehealth – Current state and
future trends”, International Journal of Medical
Informatics, Volume 75, Issue 8, Pages 565–576.
Liang, P.C. & Krause P., 2013, “Cost-effective Healthcare
Telemonitoring”, Conference on Mobile and
Information Technologies in Medicine 2013
(MobileMed), Conference Paper 4.
Limburg, M., Gemert-Pijnen, J., Nijland, N., Ossebaard,
H., Hendrix, R. & Seydel, E., 2011, “Why Business
Modeling is Crucial in the Development of eHealth
Technologies”, Journal of Medical Internet Research
(JMIR), 13(4): e124.
Marinelli, E., 2009, “Hyrax: Cloud Computing on Mobile
Device Using MapReduces”, Carnegie Mellon
University, MSc Thesis.
McCullagh, P. & Augusto, J., 2011, “The Internet of
Things: The Potential to Facilitate Health and
Wellness”, CEPIS UPGRADE, Vol. XII, Issue no. 1,
pp. 59-68.
McLean, S., Prott, D. & Sheikh, A., 2011, “Telehealthcare
for long term conditions”, BMJ 2011; 342:d120, doi:
10.1136/bmj.d120.
Muenning, P., 2008, “Cost-Effectiveness Analysis in
Health: A Practical Approach”, 2
nd
ed., Jossey-Bass,
Hoboken, NJ, USA.
National Institute for Health and Care Excellence, 2013,
“Guide to the methods of technology appraisal 2013”.
Phillips, C., 2009, “What is cost-effectiveness?”, Health
economics, 2
nd
ed., viewed 17 Apr. 2013,
<http://www.medicine.ox.ac.uk/bandolier/painres/dow
nload/whatis/Cost-effect.pdf>.
Qureshi, S. et al. 2011, “Mobile cloud computing as future
for mobile applications - Implementation methods and
challenging issues”, IEEE International Conference on
Cloud Computing and Intelligence Systems (CCIS),
Conference Proceedings, pp.467 – 471.
Sanders, C. et al., 2012, “Exploring barriers to
participation and adoption of telehealth and telecare
within the Whole System Demonstrator trial: a
qualitative study”, BMC Health Services Research
2012.
Sarasohn-Kahn, J., 2010, “How Smartphones Are
Changing Health Care for Consumers and Providers”,
California Healthcare Foundation.
Steventon, A. et al., 2012, “Effect of telehealth
interventions on use of secondary care and mortality:
findings from the Whole System Demonstrator cluster
randomised trial”, BMJ2012;344:e3874 doi:
10.1136/bmj.e3874.
Steventon, A. et al., 2013, “Effect of telecare on use of
health and social care services: findings from the
Whole System Demonstrator cluster randomised trial”,
Age and Ageing 2013; 0: 1-8 doi:
10.1093/ageing/aft008.
Sundmaeker, H. et al., 2010, “Vision and Challenges for
Realising the Internet of Things”, European
Commission.
Viet, V. Q., Lee, G. & Choi, D., 2012, “Fall Detection
based on Movement and Smart Phone Technology”,
2012 IEEE-RIVF International Conference on
Computing and Communication Technologies,
Conference Proceedings, pp.1-4.
Wang, C. C. et al., 2008, “Development of a Fall
Detecting System for the Elderly Residents”, The 2nd
International Conference on Bioinformatics and
Biomedical Engineering 2008, Conference
Proceedings, pp.1359-1362.
Wei, Z. et al,. 2010, “A Residential Gateway Architecture
Based on Cloud Computing”, 2010 IEEE International
Conference on Software Engineering and Service
Sciences (ICSESS), IEEE Conference Publications,
pp.245-248.
Wu, C. Y. et al. 2009, “Introduction and Application of
Important Digital Home Standard Alliances” (in
Chinese), TLTECHNICAL Journal, Vol. 39, No.5, pp.
601-620.
Yang, Y. & Zhao, X., 2011, “Development of a Fall
Detection Algorithm based on a Tri-axial
Accelerometer”, The 4th International Conference on
Biomedical Engineering and Informatics (BMEI),
Conference Proceedings, Vol. 3, pp.1371-1374.
ZigBee Alliance, 2009, “ZigBee Wireless Sensor
Applications for Health, Wellness and Fitness”.
ZigBee Alliance, 2012, “ZigBee 2012 Specification
Features At-A-Glance”.
Zimmermann1, G. & Vanderheiden, G., 2007, “The
Universal Control Hub : An Open Platform for
Remote User Interfaces in the Digital Home”, Lecture
Notes in Computer Science, Vol. 4551, pp.1040-1049.
Cost-effectiveDesignofReal-timeHomeHealthcareTelemonitoring
15