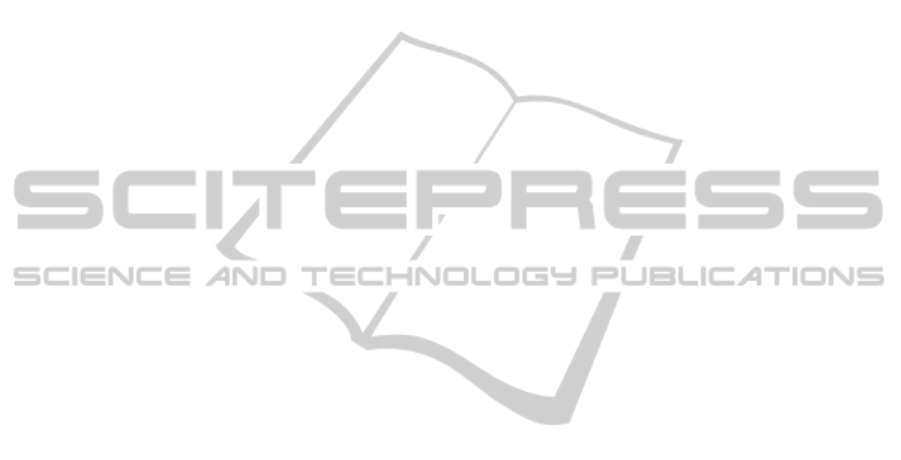
REFERENCES
Andreassi, J. L., 2007. Psychophysiology: Human
behavior and physiological response. Psychology
Press, 5th edition.
Auvray, M., & O'Regan, J.K., 2003. Influence of semantic
factors on blindness to progressive changes in visual
scenes / L'influence des facteurs sémantiques sur la
cécité aux changements progressifs dans les scènes
visuelles. L'année Psychologique. 103(1), 9-32.
Bayliss, J. D., & Ballard, D. H., 2000. A virtual reality
testbed for brain-computer interface research. IEEE
transactions on rehabilitation engineering: a
publication of the IEEE Engineering in Medicine and
Biology Society, 8(2), 188–190.
Bolter, J. D. and Gromala, D. J., 2005. Windows and
Mirrors: Interaction Design, Digital Art, and the Myth
of Transparency. Cambridge: the MIT Press.
Bartram, Lyn, and Rob Woodbury. Smart Homes or Smart
Occupants? Reframing, 2011. Artificial Intelligence
and Sustainable Design, AAAI Spring Symposium.
Rodgers, Johnny. 2010. Westhouse. Accessed 13 Sept.
2013, http://johnny.hcssl.iat.sfu.ca/tag/ambient-infovis.
Frank, D. L., Khorshid, L., Kiffer, J. F., Moravec, C. S., &
McKee, M. G., 2010. Biofeedback in medicine: who,
when, why and how? Mental Health in Family
Medicine, 7(2), 85–91.
Friesen C. K., Kingstone A., 1998. The eyes have it!
Reflexive orienting is triggered by nonpredictive gaze.
Psychonomic Bulletin & Review, 5:490–495.
Helmholtz, H., 1995. Science and Culture: Popular and
Philosophical Essays. Chicago: University of Chicago
Press, 1
st
ed.
Hoffman, H., 2000. “Virtual reality as an adjunctive pain
control during burn wound care in adolescent patients,”
Pain, 85, no. 1 (2000), 305–309.
Itier R. J., Alain C, Sedore K, McIntosh AR, 2007. Early
face processing specificity: it’s in the eyes!. J Cogn
Neurosci 19:1815–1826.
Leder, D., 1990. The Absent Body. Chicago: the
University of Chicago Press.
Monastra, V. J., Lynn, S., Linden, M., Lubar, J. F.,
Gruzelier, J., & LaVaque, T. J. , 2005.
Electroencephalographic biofeedback in the treatment
of attention-deficit/hyperactivity disorder. Applied
psychophysiology and biofeedback, 30(2), 95–114.
NeuroSky’s eSense Meters and Detection of Mental State,
2013. Neurosky. Retrieved from http://
company.neurosky.com/files/neurosky_esense_whitep
aper.pdf.
Norman, D., 1988. The Psychology of Everyday Things.
New York: Basic Books.
Norman, D., 1999. The Invisible Computer: Why Good
Products Can Fail, the Personal Computer Is So
Complex, and Information Appliances Are the Solution.
Cambridge: the MIT Press.
Rice, K. M., Blanchard, E. B., & Purcell, M., 1993.
Biofeedback treatments of generalized anxiety
disorder: preliminary results. Biofeedback and self-
regulation, 18(2), 93–105.
Schwartz, M., 1987. Biofeedback: A Practitioner's Guide.
New York: The Guilford Press.
Sellers, E. W., & Donchin, E., 2006. A P300-based brain–
computer interface: Initial tests by ALS patients.
Clinical Neurophysiology, 117(3), 538–548.
doi:10.1016/j.clinph.2005.06.027.
Shaw, C., Gromala, D , and Seay, F., 2007. “The
Meditation Chamber” Enacting Autonomic Senses.
Proceedings of ENACTIVE/07, 4th International
Conference on Enactive Interfaces.
Sterman, M. B., 2000. Basic concepts and clinical findings
in the treatment of seizure disorders with EEG operant
conditioning. Clinical EEG (electroencephalography),
31(1), 45–55.
Vecera, S. P., & Rizzo, M. , 2006. Eye gaze does not
produce reflexive shifts of visual attention: Evidence
from frontal-lobe damage. Neuropsychologia, 44, 150-
159.
Wolpaw, J. R., Birbaumer, N., McFarland, D. J.,
Pfurtscheller, G., & Vaughan, T. M., 2002. Brain-
computer interfaces for communication and control.
Clinical neurophysiology: official journal of the
International Federation of Clinical Neurophysiology,
113(6), 767–791.
Wright, R. D. and Ward, L. M., 2008. Orienting of
Attention. Oxford: Oxford University Press.
Yucha, C; Montgomery D, 2008. Evidence-based practice
in biofeedback and neurofeedback (PDF). Wheat
Ridge, CO: AAPB. Archived from the original on
2010-10-09.
OrientationofAttentioninVisualFeedbacksduringNeurofeedbackRelaxation
203