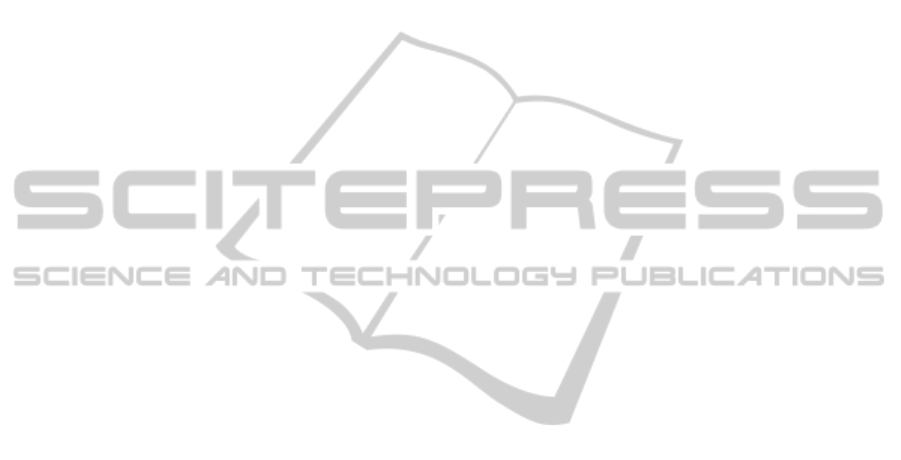
physiological measurements are the most common
approach used to interpret stress levels and
fluctuations.
In contrast to earlier work in psychology and
affective computing that focused on understanding
how a single modality (physiological signal)
changes with stress level, more recent studies have
exploited multiple modalities to improve stress
recognition performance as well as to build
automated stress recognition systems working in
real-world situations (Stäger et al., 2007). For
example, Healey and Picard collected data from four
types of physiological sensors, including an
electrocardiogram (ECG), electromyogram (EMG),
skin conductivity (also known as GSR), and
respiration while participants were performing real-
world driving tasks to measure their stress levels
(Healey and Picard, 2005). Nasoz (2010) and
colleagues developed a multi-modal driving
interface that modelled stress-related states such as
panic/fear, frustration/anger and boredom/fatigue
and used skin conductance, heart activity,
respiration, muscle activity and finger pressure.
For the development of automated stress
recognition systems, Liao et al. (2006) proposed a
framework for a dynamic probabilistic decision-
theoretic model that not only includes stress/fatigue
recognition but also optimizes the feature set used in
their model dynamically. They exploited four
different types of inputs: physiological responses,
physical appearance features, user performance and
behavioural data, and found an optimal feature set to
improve recognition performance. More recently,
Sharma and Gedeon (2012) performed a survey of
stress recognition and classification research to
provide a broad overview of investigation efforts on
a variety of physiological responses, including skin
conductivity, heart activity, brain activity, and
various computational techniques Giakoumis et al.
(2012) presented an in-depth analysis of
physiological features in stress detection and
proposed, using subject-dependent features, to
increase recognition accuracy. They extracted
subject-dependent features from skin conductivity
and ECG modalities and improved recognition
performance over a multi-subject data set collected
through an experiment using natural stress induction.
All the aforementioned work has introduced
advanced techniques and systems, but most of them
have focused on only stress as a factor of changing
physiological responses. Since there are a number of
potential factors that affect human physiology in
natural environments, we still need further
investigation about how to apply physiological stress
recognition in natural environments and what are the
effects of other factors on it, such as whether an
individual is speaking or exercising. To make a
stress recognition system that uses physiological
responses work in practice, we must be able to
detect stress even during other activities that may
affect one’s physiological responses. Since few
studies have studied the impact of such real-world
factors, our goal is to address the effect of physical
activity, as an example of a daily common event, on
physiological responses and stress recognition.
2.2 Stress and Activity Recognition
To the best of our knowledge, recognition of stress
in the presence of physical activity has only been
addressed in our previous work (Hong et al. 2012).
There is some work, in the field of
psychophysiology (Novak et al., 2011; Roth et al.,
1990; Webb et al., 2008; Yao et al., 2008) that
highlights the effect of stress while performing a
physical activity. However, this work is limited to
the understanding of the phenomena and not on
creating and validating a system or method for stress
recognition. Here, we use the same data set used in
(Hong et al., 2012) which contained physiological
measurements of stress with no stress, noise, cold
water pressor, and verbal math as the stress inducers
in the presence of different activities: sitting,
walking and biking. However, in this work, we
address the problem of stress recognition in a
different way while generalizing on our previous
approach.
The general assumption in our previous work
was that different kinds of activity have a
recognizable effect on the physiological responses.
Hence, a stress recognition model will perform
better if it is modelled for specific activities, but this
means that this model must be supervised and that
labels need to be provided. Here, we re-evaluate this
hypothesis and find that those activities, even in very
controlled scenarios do not have a stable or
unimodal distribution across the different
physiological responses recorded. Additionally, in
our previous work, we constrained the activities to
sitting, walking, and biking, these only cover a very
small spectrum of all the possible activities that a
person can perform regularly, narrowing the
applicability of our previous work to a very
unrealistic scenario. This does not mean classifying
activity is not helpful, because we have
demonstrated that it does provide an improvement
over stress classification-only systems. However, it
does mean that a more general approach is needed
PhyCS2014-InternationalConferenceonPhysiologicalComputingSystems
108