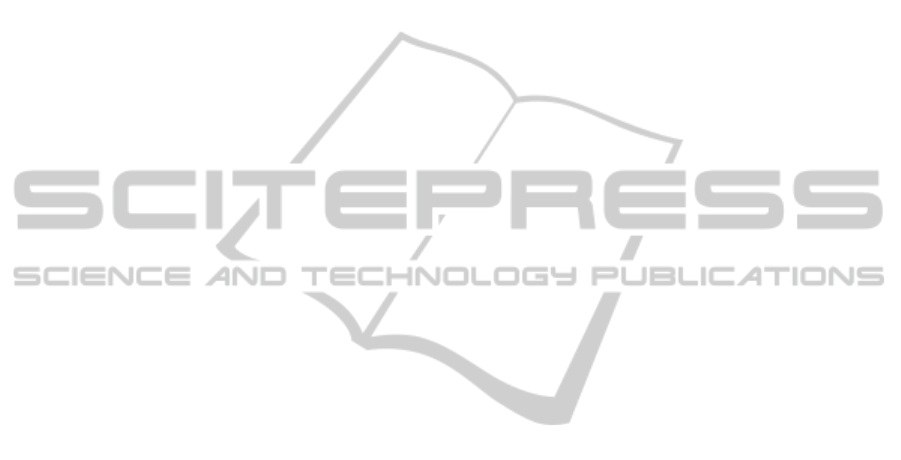
inference Ψ = f (Φ). When the relationship f is used
on a one-to-many basis (a psychological state
reflects various physiological variables), the
elements of Φ react according to different temporal
scales (e.g. EDA at 4 seconds and ECG at 1 second
post stimulus). Until now, the feature extraction
methods used in the literature neglected this
phenomenon and segmented all signals according to
a stimulus using a single window.
Our temporal construction technique provides a
solution to the problem of signal asynchrony and
allows for a more optimal triangulation of multiple
signals and recording instruments by individually
optimising each extraction window for both latency
and duration. Results from this experiment showed
how the technique improved the quality of
recognition model of arousal by 9 % and of
cognitive load by 18 %. The valence recognition
model was not improved (0%) on the average and
reduced for two algorithms (SVM and ANN). A
possible explanation for this can be found in the
bipolar nature of the valence scale. As opposed to
arousal and cognitive load which increase in a
monotonous way, valence can be conceived as
evolving in two directions (positive or negative).
Indeed, it has been suggested to replace the bipolar
scale with two unipolar scales (van den Broek,
2011). With this in mind it is logical that a unique
relationship between values from the bipolar scale
and optimal temporal windows is hard to establish.
We now believe that different optimal windows can
exist for a given physiological signal, depending
upon the positivity or negativity of valence. Future
works should also include looking for gender, age or
personality effects on the value of the optimal
windows’ latency and duration. It could therefore be
possible to tailor more precisely the extraction
windows for specific subjects.
Following the large sample size of this study
(n=44 for valence and arousal and n=31 for
cognitive load), it can be expected that the
empirically optimised values for the extraction
windows can be used successfully in other studies.
To do so, we included in the Appendix (Figure 9)
the aforementioned values. Researchers working on
the physiological recognition of valence, arousal or
cognitive load could use these values while
segmenting signals according to their stimuli – being
that they are alike – and look for a gain in
recognition accuracy. The proposed approach could
also be adapted to different recognition contexts by
optimising extraction windows for various
physiological signals, psychological constructs or
stimuli.
ACKNOWLEDGEMENTS
This work was supported by NSERC (Natural
Sciences and Engineering Research Council of
Canada), the Canadian Space Agency and Bell
Canada. The authors would like to thank the Bell
Web Solutions User Experience Center for
providing the eye-tracker system used in this
research. We also wish to thank Laurence Dumont
for early comments on the manuscript.
REFERENCES
Allanson, J. & Fairclough, S. H. 2004. A research agenda
for physiological computing. Interacting with
Computers, 16, 857-878.
Anttonen, J. & Surakka, V. 2005. Emotions and heart rate
while sitting on a chair. SIGCHI conference on Human
factors in computing systems. Portland, Oregon, USA:
ACM.
Bamidis, P., Frantzidis, C., Konstantinidis, E., Luneski,
A., Lithari, C., Klados, M., Bratsas, C., Papadelis, C.
& Pappas, C. 2009. An Integrated Approach to
Emotion Recognition for Advanced Emotional
Intelligence. In: JACKO, J. (ed.) Human-Computer
Interaction. Ambient, Ubiquitous and Intelligent
Interaction. Springer Berlin / Heidelberg.
Bradley, M. M. & Lang, P. J. 1994. Measuring emotion:
The self-assessment manikin and the semantic
differential. Journal of Behavior Therapy and
Experimental Psychiatry, 25, 49-59.
Bradley, M. M. & Lang, P. J. 2007. The International
affective digitized sounds (2nd Edition; IADS-2):
Affective Ratings of Sounds and Instruction Manual.
Technical report B-3. University of Florida,
Gainesville, FI.
Cacioppo, J. T. & Tassinary, L. G. 1990. Inferring
psychological significance from physiological signals.
American Psychologist, 45, 16-28.
Cacioppo, J. T., Tassinary, L. T. & Berntson, G. 2007.
Handbook of Psychophysiology, New York:
Cambridge university Press.
Chanel, G., Kierkels, J. J. M., Soleymani, M. & Pun, T.
2009. Short-term emotion assessment in a recall
paradigm. International Journal of Human-Computer
Studies, 67, 607-627.
Christie, I. C. & Friedman, B. H. 2004. Autonomic
specificity of discrete emotion and dimensions of
affective space: a multivariate approach. International
Journal of Psychophysiology, 51, 143-153.
Coan, J. A. & Allen, J. J. B. 2004. Frontal EEG
asymmetry as a moderator and mediator of emotion.
Biological Psychology, 67, 7-50.
Cowie, R., Douglas-Cowie, E., Mcrorie, M., Sneddon, I.,
Devillers, L. & Amir, N. 2011. Issues in Data
Collection. In: COWIE, R., PELACHAUD, C. &
PETTA, P. (eds.) Emotion-Oriented Systems. Springer
AddressingSignalsAsynchronicityduringPsychophysiologicalInference-ATemporalConstructionMethod
125