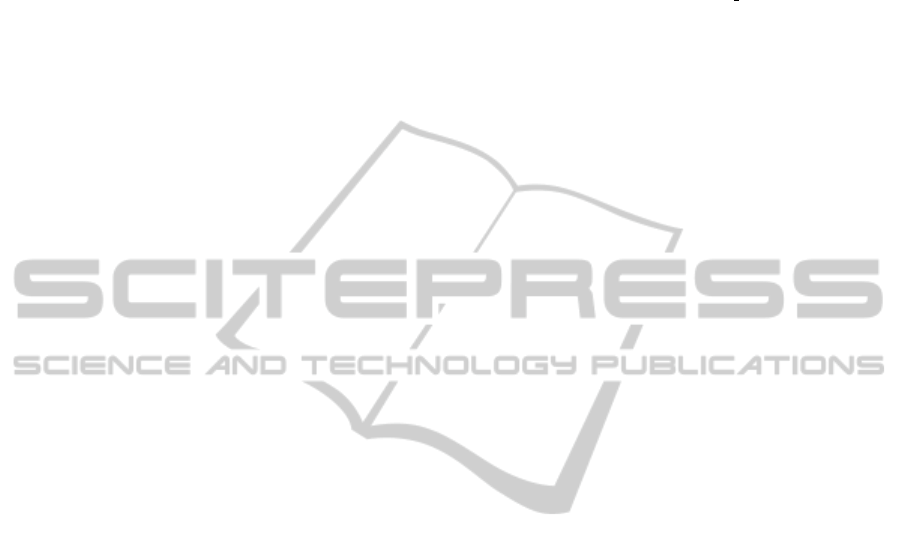
H
¨
ame, Y., Angelini, E. D., Hoffman, E. A., Barr, R. G.,
and Laine, A. F. (2013). Robust quantification of pul-
monary emphysema with a hidden Markov measure
field model. In Proc. 10th IEEE Int’l Symposium on
Biomedical Imaging, pages 382–385. IEEE.
Hara, T., Yamamoto, A., Zhou, X., Iwano, S., Itoh, S., Fu-
jita, H., and Ishigaki, T. (2004). Automated detection
system for pulmonary emphysema on 3D chest CT
images. In Proc. of SPIE, pages 915–919.
Kashyap, R. L. and Khotanzad, A. (1986). A model-based
method for rotation invariant texture classification.
IEEE Trans. on Pattern Analysis and Machine Intel-
ligence, (4):472–481.
Kim, N., Seo, J. B., Lee, Y., Lee, J. G., Kim, S. S., ,
and Kang, S. (2009). Development of an automatic
classification system for differentiation of obstructive
lung disease using hrct. Journal of digital imaging,
22(2):136–148.
Li, S. Z. (2009). Markov Random Field Modeling in Im-
age Analysis. Springer-Verlag London Ltd, 3rd edn
edition.
Litmanovich, D., Boiselle, P. M., and Bankier, A. (2009).
CT of pulmonary emphysema - current status, chal-
lenges, and future directions. 19:537–51.
Madani, A., Keyzer, C., and AGevenois, P. (2001). Quanti-
tative computed tomography assessment of lung struc-
ture and function in pulmonary emphysema. 18
(4):720–30.
Mahmoodi, S. and Gunn, S. (2011). Snake based unsuper-
vised texture segmentation using Gaussian Markov
random field models. In Proc. 18th IEEE Int’l Conf.
Image Processing, pages 1–4.
Manjunath, B. S. and Chellappa, R. (1991). Unsuper-
vised texture segmentation using Markov random
field models. IEEE Trans. on pattern analysis and
machine intelligence, 13(5):478–482.
Mishima, M., T. Hirai, H. I., Nakano, Y., Sakai, H., Muro,
S., Nishimura, K., Oku, Y., Chin, K., Ohi, M., Naka-
mura, T., Bates, J. H. T., Alencar, A. M., and Suki,
B. L. (1999). Complexity of terminal airspace ge-
ometry assessed by lung computed tomography in
normal subjects and patients with chronic obstructive
pulmonary disease. In Proceedings of the National
Academy of Sciences, volume 96, pages 8829–8834.
Muller, N., Staples, C., and R. Miller, R. A. (1988). Density
mask, an objective method to quantitate emphysema
using computed tomography. 94:782–787.
Ojala, T., Pietikainen, M., and Maenpaa, T. (2002). Mul-
tiresolution gray-scale and rotation invariant texture
classification with local binary patterns. IEEE Trans.
on pattern analysis and machine intelligence, 24:971–
987.
Petrou, M. and Sevilla, P. G. (2006). Image Processing,
Dealing with Texture. John Wiley & Sons Ltd.
Rue, H. and Held, L. (2005). Gaussian Markov Ran-
dom Fields; Theory and Applications. Chapman &
Hall/CRC.
Sluimer, I. C., Waes, P. F., Viergever, M., and Ginneken, B.
(2003). Computer-aided diagnosis in high resolution
CT of the lungs. 30:3081.
Sørensen, L., Shaker, S. B., and de Bruijne, M. (2010).
Quantitative analysis of pulmonary emphysema using
local binary patterns. IEEE Transactions on Medical
Imaging, 29(2):559–569.
Sørensen, L., Shaker, S. B., and de Bruijne, M. (2013).
Computed Tomography Emphysema Database.
http://image.diku.dk/emphysema database/.
Sprawls, P. (1995). The Physical Principles of Medical
Imaging. Medical Physics Publishing, USA, 2nd edi-
tion.
Uppaluri, R., Hoffman, E., M.Sonka, Hartley, P. G.,
W.Hunninghake, G., and McLennan, G. (1999). Com-
puter recognition of regional lung disease patterns.
160 (2):648–54.
Vasconcelos, V., Silva, J. S., Marques, L., and Barroso,
J. (2010). Statistical textural features for classifica-
tion of lung emphysema in CT images: A compara-
tive study. In Information Systems and Technologies
(CISTI), pages 1–5. IEEE.
Xu, Y., Sonka, M., McLennan, G., Guo, J., and Hoffman,
E. A. (2006). MDCT-based 3-D texture classifica-
tion of emphysema and early smoking related lung
pathologies. 156 (1):25.
Zhao, Y., Zhang, L., Li, P., and Huang, B. (2007). Classi-
fication of high spatial resolution imagery using im-
proved Gaussian Markov random-field-based texture
features. IEEE Trans. on Geoscience and Remote
Sensing, 45(5):1458–1468.
QuantitativeAnalysisofPulmonaryEmphysemausingIsotropicGaussianMarkovRandomFields
53