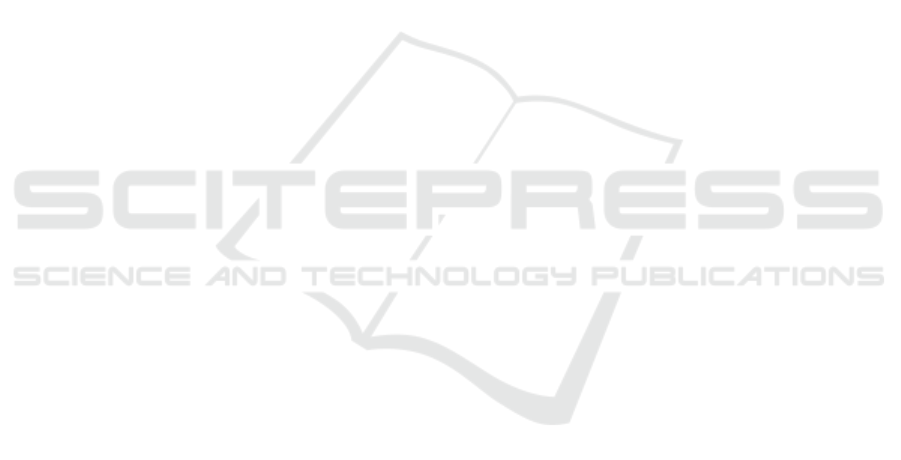
1-D Temporal Segments Analysis for Traffic Video Surveillance
M. Brulin,
C. Maillet and H. Nicolas
Labri, University of Bordeaux, Talence, France
Keywords: Video Surveillance, Traffic, Temporal Segment, Behavior.
Abstract: Traffic video surveillance is an important topic for security purposes and to improve the traffic flow
management. Video surveillance can be used for different purposes such as counting of vehicles or to detect
their speed and behaviors. In this context, it is often important to be able to analyze the video in real-time.
The huge amount of data generated by the increasing number of cameras is an obstacle to reach this goal. A
solution consists in selecting in the video only the regions of interest, essentially the vehicles on the road
areas. In this paper, we propose to extract significant segments of the regions of interest and to analyze them
temporally to count vehicles and to define their behaviors. Experiments on real data show that precise
vehicle’s counting and high recall and precision are obtain for vehicle’s behavior and traffic analysis.
1 INTRODUCTION
For several years, traffic video-surveillance is under
fast development and is important for security
purposes and to improve the traffic flow
management (Kastrina et al., 2003); (Buch et al.,
2011); (Tian et al., 2011). The aim consists in the
extraction from the video data of information related
to the vehicles behaviors and to the traffic flow. In
order to be really efficient, such a video surveillance
system has to be fully automatic and able to provide
in real time information concerning the object’s
behaviors in the scene. Events of interest are
essentially: vehicles entering or exiting the scene,
vehicle collisions, accident, too fast (or too low)
vehicles' speed, stopped vehicles or objects
obstructing part of the road, vehicle’s classification
(car, trucks, bicycle, pedestrian …), objects in
forbidden areas, normal and abnormal trajectories or
for statistical purposes (estimation of the number of
vehicles, their average speed, and the number of
vehicles which change their traffic lane…) (Zhu et
al., 2000); (Yoneyama et al., 2005); (Rodriguez and
Garcia, 2010). This requires obtaining information
concerning the vehicle’s texture and contours
(Bissacco et al., 2004), motion (Adam et al., 2008),
trajectories and speed (Stauffer, 2003).
To reach the real-time constraint while keeping
efficient analysis, the computational cost has to be
reduced. For that purpose, two solutions can be
investigated. A first solution is to reduce as much as
possible the computational load of the motion
estimation and object based segmentation
algorithms, with the risk to get sub optimal
estimations. A second solution consists in the
reduction of the amount of video data which has to
be treated. This solution appears to be interesting for
traffic video surveillance applications for which
large parts of the images do not contain any
interesting information.
In this context, a first solution is to define the
region of interest (ROI) in the images. For traffic
video surveillance, these areas correspond generally
to the road areas. The other parts of the images are
useless and can be eliminated. Frame skipping is
also a potential solution. Nevertheless, the increased
temporal distance does not simplify the spatio-
temporal analysis. If these solutions are interesting,
they are not sufficient to reduce sufficiently the
amount of original data which have to be analyzed.
In order to better reach our goal, it is necessary to
take into account the kind of information really
useful for the vehicle’s behavior analysis, i.e., the
trajectories and the size of the moving vehicles. A
promising solution consists in the extraction of the
temporal evolution of selected spatial segments (or
scanlines) in the image (Malinovski et al., 2009);
(Zhu et al., VISITRAM). Such 1-D segments
represent a very low amount of data and can
therefore be quickly analyzed. If they are chosen
wisely they can contain enough information
concerning the moving vehicles to allow an analysis
557
Brulin M., Maillet C. and Nicolas H..
1-D Temporal Segments Analysis for Traffic Video Surveillance.
DOI: 10.5220/0004733905570563
In Proceedings of the 9th International Conference on Computer Vision Theory and Applications (VISAPP-2014), pages 557-563
ISBN: 978-989-758-003-1
Copyright
c
2014 SCITEPRESS (Science and Technology Publications, Lda.)