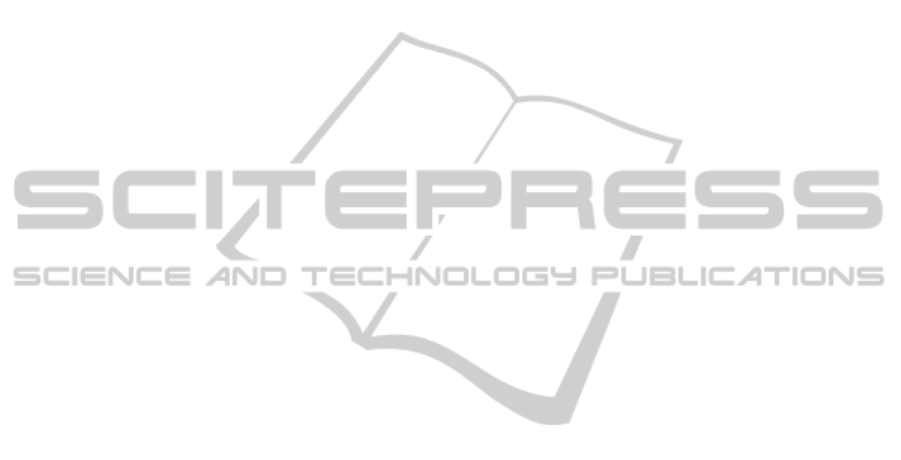
Health Education Research, 20, 275-290.
Phansalkar, S., Desai, A. A., Bell, D., Yoshida, E., Doole,
J., Czochanski, M., Middleton, B. & Bates, D. W.
2012a. High-priority drug–drug interactions for use in
electronic health records. Journal of the American
Medical Informatics Association, 19, 735-743.
Phansalkar, S., van der Sijs, H., Tucker, A. D., Desai, A.
A., Bell, D. S., Teich, J. M., Middleton, B. & Bates, D.
W. 2012b. Drug–drug interactions that should be non-
interruptive in order to reduce alert fatigue in
electronic health records. Journal of the American
Medical Informatics Association.
Quine, W. V. O. 1961. From a Logical Point of View,
Boston, MA, USA, Harvard University Press.
Rahmner, P. B., Eiermann, B., Korkmaz, S., Gustafsson,
L. L., Gruvén, M., Maxwell, S., Eichle, H.-G. & Vég,
A. 2012. Physicians' reported needs of drug
information at point of care in Sweden. British Journal
of Clinical Pharmacology, 73, 115-125.
Ramaprasad, A. 1979. Role of Feedback in
Organizational-Change - Review and Redefinition.
Cybernetica, 22, 105-113.
Ramaprasad, A. 1983. On the Definition of Feedback.
Behavioral Science, 28, 4-13.
Ramaprasad, A. 1987. Cognitive Process as a Basis for
MIS and DSS Design. Management Science, 33, 139-
148.
Ramaprasad, A. & Mitroff, I. I. 1984. On Formulating
Strategic Problems. Academy of Management Review,
9, 597-605.
Ramaprasad, A. & Syn, T. 2013. Ontological Meta-
Analysis and Synthesis. Proceedings of the Nineteenth
Americas Conference on Information Systems,
Chicago, Illinois, August 15-17, 2013.
Ramaprasad, A., Valenta, A. L. & Brooks, I. 2009.
Clinical and Translational Science Informatics:
Translating Information to Transform Health Care. In:
AZEVEDO, L. & LONDRAL, A. R. (eds.)
Proceedings of HEALTHINF 2009 – Second
International Conference on Health Informatics.
Porto, Portugal: INSTICC Press.
Saverno, K. R., Hines, L. E., Warholak, T. L., Grizzle, A.
J., Babits, L., Clark, C., Taylor, A. M. & Malone, D.
C. 2011. Ability of pharmacy clinical decision-support
software to alert users about clinically important drug–
drug interactions. Journal of the American Medical
Informatics Association, 18, 32-37.
Scharnhorst, A. 2001. Constructing Knowledge
Landscapes Within the Framework of Geometrically
Oriented Evolutionary Theories. In: MATHIES, M.,
MALCHOW, H. & KRIZ, J. (eds.) Inegrative Systems
Approaches to Natural Social Dynamics.
http://www.virtualknowledgestudio.nl/staff/andrea-
scharnhorst/documents/constructing- knowledge-
landscapes.pdf: Springer.
Seidling, H. M., Phansalkar, S., Seger, D. L., Paterno, M.
D., Shaykevich, S., Haefeli, W. E. & Bates, D. W.
2011. Factors influencing alert acceptance: a novel
approach for predicting the success of clinical decision
support. Journal of the American Medical Informatics
Association, 18, 479-484.
Smithburger, P. L., Buckley, M. S., Bejian, S.,
Burenheide, K. & Kane-Gill, S. L. 2011. A critical
evaluation of clinical decision support for the
detection of drug-drug interactions. Expert Opinion on
Drug Safety, 10, 871-882.
Spina, J. R., Glassman, P. A., Simon, B., Lanto, A., Lee,
M., Cunningham, F. & Good, C. B. 2011. Potential
Safety Gaps in Order Entry and Automated Drug
Alerts: A Nationwide Survey of VA Physician Self-
Reported Practices With Computerized Order Entry.
Medical Care, 49, 904-910.
Syed, K., Kröll, M., Sabol, V., Scharl, A., Gindl, S.,
Granitzer, M. & Weichselbraun, A. 2012. Dynamic
Topography Information Landscapes–An Incremental
Approach to Visual Knowledge Discovery. Data
Warehousing and Knowledge Discovery, 352-363.
Takarabe, M., Shigemizu, D., Kotera, M., Goto, S. &
Kanehisa, M. 2011. Network-Based Analysis and
Characterization of Adverse Drug–Drug Interactions.
Journal of chemical information and modeling, 51,
2977-2985.
Varroud-Vial, M. 2011. Improving diabetes management
with electronic medical records. Diabetes Metab, 37
Suppl 4, S48-52.
Warholak, T. L., Hines, L. E., Saverno, K. R., Grizzle, A.
J. & Malone, D. C. 2011. Assessment tool for
pharmacy drug–drug interaction software. Journal of
the American Pharmacists Association, 51, 418-424.
Yu, D. T., Seger, D. L., Lasser, K. E., Karson, A. S.,
Fiskio, J. M., Seger, A. C. & Bates, D. W. 2011.
Impact of implementing alerts about medication
blackbox warnings in electronic health records.
Pharmacoepidemiology and drug safety, 20, 192-202.
Zhang, J., Xie, J., Hou, W., Tu, X., Xu, J., Song, F.,
Wang, Z. & Lu, Z. 2012. Mapping the Knowledge
Structure of Research on Patient Adherence:
Knowledge Domain Visualization Based Co-Word
Analysis and Social Network Analysis. PLoS ONE, 7.
HEALTHINF2014-InternationalConferenceonHealthInformatics
26