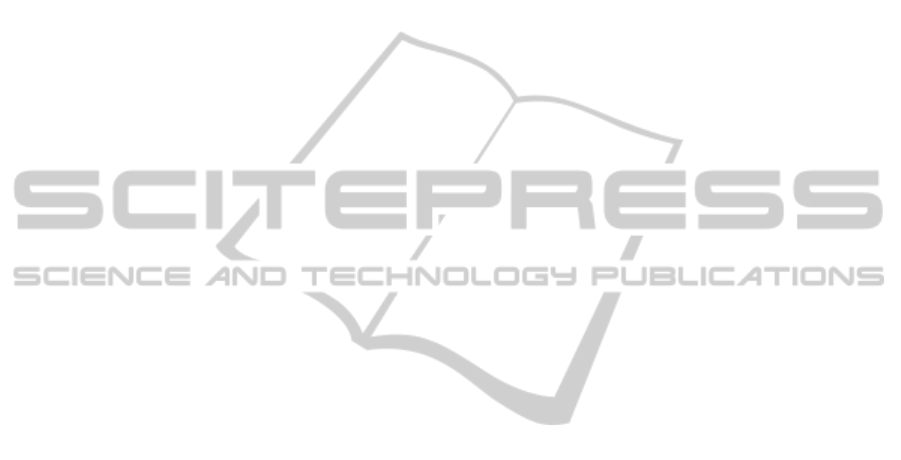
In a similar manner, the frames of the animation
illustrating the other highly-ranked employees were
investigated. We gave more attention to the 17 frames
of the animation containing periodic events. Fortu-
nately for the company, the only real evidence of
fraud existed in the fictional data added by the au-
ditors. However, the auditors had not identified all
these cases while examining the data-sets manually
and they had to make an additional investigation.
6 CONCLUSIONS AND FUTURE
WORK
We presented an integrated fraud management visual-
ization system that aims to identify patterns that may
conceal occupational fraud through a combination of
pattern recognition and visualization. Our work opens
several aspects for future work such as incorporation
of more fraud patterns, use of more statistical methods
and, extension of the system in order to identify more
complicated fraud schemes (client fraud, telecommu-
nication fraud, etc.) in a wider variety of business
systems.
ACKNOWLEDGEMENTS
The work of Evmorfia N. Argyriou has been co-
financed by the European Union (European Social
Fund ESF) and Greek national funds through the
Operational Program ”Education and Lifelong Learn-
ing” of the National Strategic Reference Framework
(NSRF) - Research Funding Program: Heracleitus II.
Investing in knowledge society through the European
Social Fund.
REFERENCES
Argyriou, E. N., Sotiraki, A., and Symvonis, A. (2013a).
Occupational fraud detection through visualization. In
ISI, pages 4–7.
Argyriou, E. N. and Symvonis, A. (2012). Detecting peri-
odicity in serial data through visualization. In ISVC,
volume 7432, pages 295–304.
Argyriou, E. N., Symvonis, A., and Vassiliou, V. (2013b).
A fraud detection visualization system utilizing ra-
dial drawings and heat-maps. Technical report,
arXiv:submit/0845288.
Association of Certified Fraud Examiners (2012). Report to
the Nation on Occupational Fraud and Abuse.
Bolton, R. J. and Hand, D. J. (2002). Statistical fraud de-
tection: A review. Statistical Science, 17:2002.
Chang, R., Lee, A., Ghoniem, M., Kosara, R., and Ribarsky,
W. (2008). Scalable and interactive visual analysis of
financial wire transactions for fraud detection. Infor-
mation Visualization, 7(1):63–76.
Didimo, W., Liotta, G., and Montecchiani, F. (2012).
Vis4aui: Visual analysis of banking activity networks.
In GRAPP/IVAPP, pages 799–802.
Didimo, W., Liotta, G., Montecchiani, F., and Palladino, P.
(2011). An advanced network visualization system for
financial crime detection. In PacificVis, pages 203–
210.
Eberle, W. and Holder, L. B. (2009). Mining for insider
threats in business transactions and processes. In
CIDM, pages 163–170.
Giacomo, E. D., Didimo, W., Liotta, G., and Palladino, P.
(2010). Visual analysis of financial crimes. In AVI,
pages 393–394.
Huang, M. L., Liang, J., and Nguyen, Q. V. (2009). A vi-
sualization approach for frauds detection in financial
market. IV ’09, pages 197–202.
Kou, Y., Lu, C.-T., Sirwongwattana, S., and Huang, Y.-P.
(2004). Survey of fraud detection techniques. In Net-
working, Sensing and Control, 2004 IEEE Int. Conf.,
volume 2, pages 749–754.
Luell, J. (2010). Employee fraud detection under real world
conditions. PhD thesis.
Phua, C., Lee, V. C. S., Smith-Miles, K., and Gayler, R. W.
(2010). A comprehensive survey of data mining-based
fraud detection research. CoRR, abs/1009.6119.
Senator, T. E., Goldberg, H. G., Shyr, P., Bennett, S.,
Donoho, S., and Lovell, C. (2002). chapter The
NASD regulation advanced detection system: inte-
grating data mining and visualization for break detec-
tion in the NASDAQ stock market, pages 363–371.
Senator, T. E., Goldberg, H. G., Wooton, J., Cottini, M. A.,
Khan, A. F. U., Klinger, C. D., Llamas, W. M., Mar-
rone, M. P., and Wong, R. W. H. (1995). The financial
crimes enforcement network ai system (fais) identify-
ing potential money laundering from reports of large
cash transactions. AI Magazine, 16(4):21–39.
Stillwell, W. G., Seaver, D. A., and Edwards, W. (1981).
A comparison of weight approximation techniques in
multiattribute utility decision making. Organ. Behav-
ior and Human Performance, 28(1):62 – 77.
SynerScope (2011). http://www.synerscope.com/.
Vlachos, M., Hadjieleftheriou, M., Gunopulos, D., and
Keogh, E. (2003). Indexing multi-dimensional time-
series with support for multiple distance measures. In
ACM SIGKDD int. conf. on Knowledge discovery and
data mining, KDD ’03, pages 216–225.
Wolverton, M., Berry, P., Harrison, I., Lowrance, J., Mor-
ley, D., Rodriguez, A., Ruspini, E., and Thomere, J.
(2003). Law: A workbench for approximate pattern
matching in relational data. In IAAI, pages 143–150.
IVAPP2014-InternationalConferenceonInformationVisualizationTheoryandApplications
160