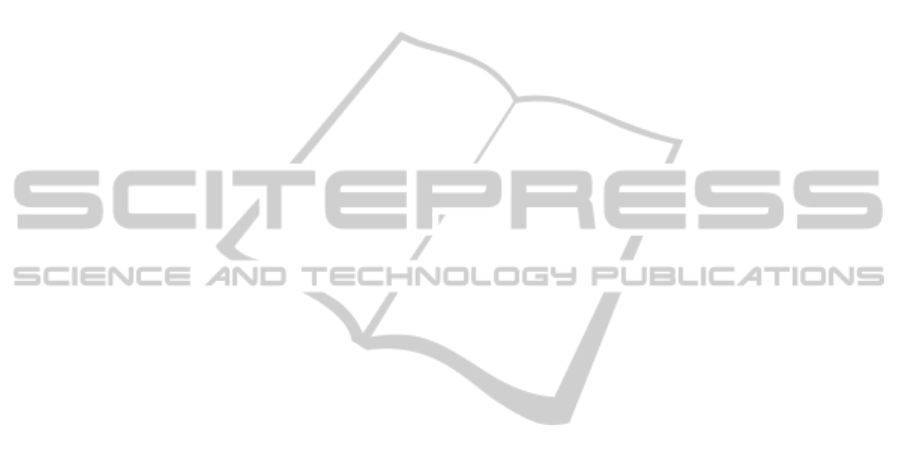
with the best matching criteria (currently a winner-
takes-all is considered). Additionally, ground truth
values will be considered for such a validation. As
mentioned in Section 1, current manuscript is in-
tended to show preliminary results from our approach
and how the proposed scheme can represent an alter-
native to overcome the lack of correlation, or to im-
prove the results from cross-spectral correspondence
search.
ACKNOWLEDGEMENTS
This work has been partially supported by the Span-
ish Government under Research Project TIN2011-
25606 and PROMETEO Project of the ”Secretar´ıa
Nacional de Educaci´on Superior, Ciencia, Tecnolog´ıa
e Innovaci´on de la Rep´ublica del Ecuador”. Cristhian
A. Aguilera-Carrasco was supported by a grant from
”Universitat Aut`onoma de Barcelona”.
REFERENCES
Barrera, F., Lumbreras, F., and Sappa, A. D. (2012). Mul-
timodal stereo vision system: 3d data extraction and
algorithm evaluation. J. Sel. Topics Signal Process-
ing, 6(5):437–446.
Barrera, F., Lumbreras, F., and Sappa, A. D. (2013). Mul-
tispectral piecewise planar stereo using manhattan-
world assumption. Pattern Recognition Letters,
34(1):52–61.
Bouguet, J.-Y. (2010). Camera calibration toolbox for mat-
lab.
Faugeras, O., Hotz, B., Mathieu, H., Vieville, T., Zhang, Z.,
Fua, P., Theron, E., Moll, L., Berry, G., Vuillemin, J.,
Bertin, P., and Proy, C. (1993). Real time correlation-
based stereo: algorithm, implementations and appli-
cations. In INRIA Technical Report, No. 2013, August
1993, page 56.
Horn, B. K. P. and Schunk, B. G. (1981). Determining op-
tical flow. Artificial Intelligence, 17:185–203.
Krotosky, S. J. and Trivedi, M. M. (2007). On color-,
infrared-, and multimodal-stereo approaches to pedes-
trian detection. IEEE Trans. on Intelligent Transporta-
tion Systems, 8(4):619–629.
Krotosky, S. J. and Trivedi, M. M. (2008). Person surveil-
lance using visual and infrared imagery. IEEE Trans.
on Circuits and Systems for Video Technology, 18(8).
Onkarappa, N. and Sappa, A. D. (2013). Laplacian deriva-
tive based regularization for optical flow estimation in
driving scenario. In 15th International Conference on
Computer Analysis of Images and Patterns, York, UK,
August 27-29, 2013, pages 483–490.
Pistarelli, M. D., Sappa, A. D., and Toledo, R. (2013). Mul-
tispectral stereo image correspondence. In 15th Inter-
national Conference on Computer Analysis of Images
and Patterns, York, UK, August 27-29, 2013, pages
217–224.
Psarakis, E. Z. and Evangelidis, G. D. (2005). An enhanced
correlation-based method for stereo correspondence
with sub-pixel accuracy. In 10th IEEE International
Conference on Computer Vision, 17-20 October 2005,
Beijing, China, pages 907–912.
Scharstein, D. and Szeliski, R. (2002). A taxonomy and
evaluation of dense two-frame stereo correspondence
algorithms. International Journal of Computer Vision,
47(1-3):7–42.
Shimizu, M. and Okutomi, M. (2001). Precise sub-pixel
estimation on area-based matching. In International
Conference on Computer Vision, pages 90–97.
Snidaro, L., Foresti, G. L., Niu, R., and Varshney, P. K.
(2004). Sensor fusion for video surveillance. In Elec-
trical Engineering and Computer Science.
Socolinsky, D. A. and Wolff, L. B. (2009). Face recogni-
tion in low-light environments using fusion of ther-
mal infrared and intensified imagery. In Hammoud,
R. I., editor, Augmented Vision Perception in Infrared,
Advances in Pattern Recognition, pages 197–211.
Springer London.
Torabi, A., Najafianrazavi, M., and Bilodeau, G. (2011).
A comparative evaluation of multimodal dense stereo
correspondence measures. In IEEE Int’l Symp. on
Robotic and Sensors Environments, volume 1, pages
143–148.
Yang, R. and Chen, Y. (2011). Design of a 3-D infrared
imaging system using structured light. IEEE Trans. on
Instrumentation and Measurement, 60(2):608 –617.
Cross-spectralStereoCorrespondenceusingDenseFlowFields
617