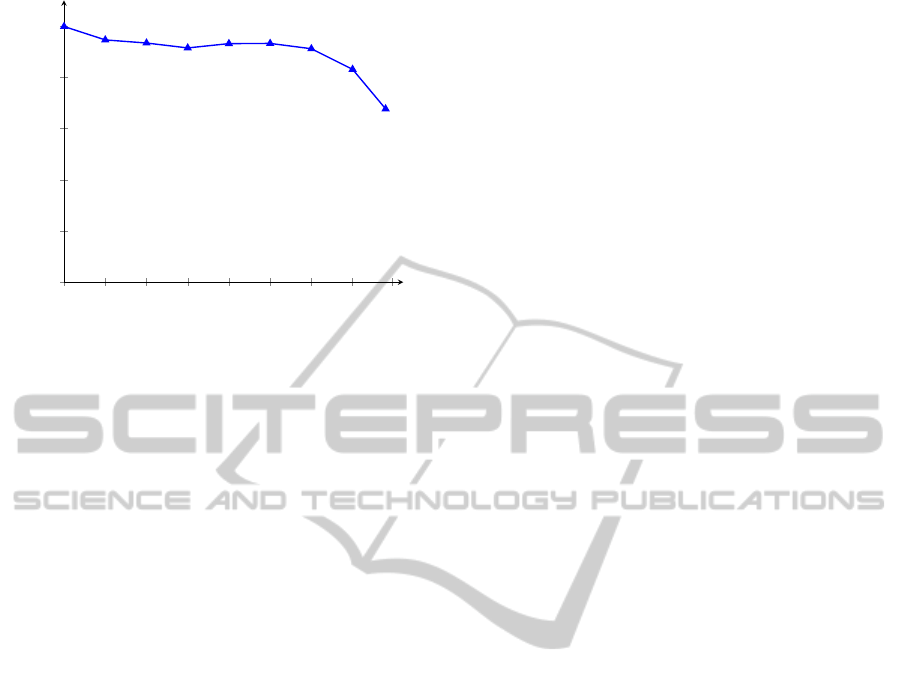
10
0
10
0.3
10
0.6
10
0.9
10
1.2
10
1.51
10
1.81
10
2.11
10
2.4
0
0.2
0.4
0.6
0.8
1
Amount o f dimensionality reduction (log)
Correlation
Figure 8: The correlation measurement value of SDR is
very high (which is equal to 0.998) when the amount of di-
mensionality reduction is equal to 1. The efficiency of SDR
is reduced when this reduction amount is greater than 1,
and the lowest correlation value is (0.676) when reduction
amount is equal to 223.
the speed of SDR on GPU is much faster than it is on
CPU.
ACKNOWLEDGEMENTS
It would not have been possible to write this paper
without the help and support of the Ministry of Higher
Eduction and Scientific Research in Iraq. The authors
would like to acknowledge the financial and academic
support of the Basrah and Bangor Universities.
REFERENCES
Agrafiotis, D. K., Xu, H., Zhu, F., Bandyopadhyay, D.,
and Liu, P. (2010). Stochastic proximity embedding:
Methods and applications. Molecular Informatics,
29:758–770.
Ameer, P. M. and Jacob, L. (2012). Localization us-
ing stochastic proximity embedding for underwater
acoustic sensor networks. In 2012 National Confer-
ence on Communications (NCC).
AVIRIS (2013). http://aviris.jpl.nasa.gov/index.html.
Bachmann, C. M., Ainsworth, T. L., and Fusina, R. A.
(2005). Exploiting manifold geometry in hyperspec-
tral imagery. IEEE transaction on geoscience and re-
mote sensing, 43:441–454.
Belkin, M. and Niyogi, P. (2002). Laplacian eigenmaps
and spectral techniques for embedding and clustering.
Advances in Neural Information Processing Systems,
14:585591.
Blum, R. S. and Liu, Z. (2006). Multi-sensor Image Fusion
and its Applications. Taylor & Francis Group.
Borg, I. and Groenen, P. J. (1997). Modern Multidimen-
sional Scaling : Theory and Applications. Springer-
Verlag, NewYork.
Bunte, K., Haase, S., Biehl, M., and Villmann, T. (2012).
Stochastic neighbor embedding (sne) for dimension
reduction and visualization using arbitrary diver-
gences. Neurocomputing, 90:23–45.
Darlington, R. B. (1999). Factor analysis. Technical report,
Cornell University,.
Demartines, P. and Hrault, J. (1997). Curvilinear compo-
nent analysis: a self-organizing neural network for
nonlinear mapping of data sets. IEEE Transactions
on Neural Networks, 8:148–154.
Donoho, D. L. and Grimes, C. (2005). Hessian eigenmaps:
New locally linear embedding techniques for high-
dimensional data. In The National Academy of Sci-
ences, 102(21):74267431.
Duda, R. O., Hart, P. E., and Stork, D. G. (2001). Pattern
Classification. Wiley Interscience Inc.
He, X., Cai, D., Yan, S., and Zhang, H.-J. (2005). Neigh-
borhood preserving embedding. In Tenth IEEE Inter-
national Conference on Computer Vision (ICCV) (Vol-
ume:2 ).
Jolliffe, I. (2002). Principal Component Analysis. Springer
Verlag, New York, Inc.
Kaski, S. and Peltonen, J. (2011). Dimensionality reduction
for data visualization. IEEE signal processing maga-
zine, 28:100–104.
Lawrence, J., Arietta, S., Kazhdan, M., Lepage, D., and
OHagan, C. (2011). A user-assisted approach to
visualizing multidimensional images. IEEE Trans-
action on Visualization and Computer Graphics,
17(10):1487–1498.
Lee, J. A., Lendasse, A., and Verleysen, M. (2004). Non-
linear projection with curvilinear distances: Isomap
versus curvilinear distance analysis. Neurocomputing,
57:49–76.
Lespinats, S. and Aupetit, M. (2011). Checkviz: Sanity
check and topological clues for linear and non-linear
mappings. Comput. Graph. Forum, 30:113–125.
Maaten, L. V. D. and Hinton, G. (2008). Visualizing data us-
ing t-sne. Machine Learning Research, 9:2579–2605.
Maaten, L. V. D., Postma, E., and Herik, H. V. D. (2009).
Dimensionality reduction: A comparative review. Ma-
chine Learning Research, 10:1–41.
Mignotte, M. (2012). A bicriteria-optimization-approach-
based dimensionality-reduction model for the color
display of hyperspectral images. IEEE Transactions
on Geoscience and Remote Sensing, 50:501–513.
Mokbel, B., Lueks, W., Gisbrecht, A., and Hammera, B.
(2013). Visualizing the quality of dimensionality re-
duction. Neuocomputing, 112:109–123.
Peltonen, J. (2009). Visualization by linear projections as
information retrieval. Advances in Self-Organizing
Maps Lecture Notes in Computer Scienc, 5629:237–
245.
Roweis, S. T. and Saul, L. K. (2000). Nonlinear dimension-
ality reduction by locally linear embedding. science,
290:2323–2326.
Sanders, J. and Kandrot, E. (2011). CUDA by example.
Addison-Wesley Professional.
IVAPP2014-InternationalConferenceonInformationVisualizationTheoryandApplications
78