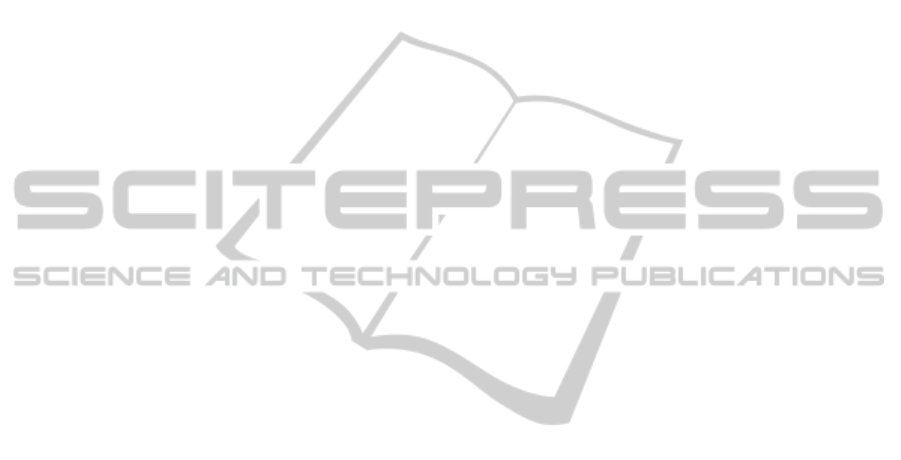
Dijkstra, E. (1959). A note on two problems in connexion
with graphs. Numerische Mathematik, 1(1):269–271.
Domingos, P. and Pazzani, M. (1997). On the optimality
of the simple bayesian classifier under zero-one loss.
Machine learning, 29(2):103–130.
Endres, F., Hess, J., Engelhard, N., Sturm, J., Cremers, D.,
and Burgard, W. (2012). An evaluation of the rgb-
d slam system. In Robotics and Automation (ICRA),
2012 IEEE International Conference on, pages 1691–
1696.
Ganapathi, V., Plagemann, C., Thrun, S., and Koller, D.
(2010). Real time motion capture using a single time-
of-flight camera. In Proceedings of the IEEE Con-
ference on Computer Vision and Pattern Recognition
(CVPR), pages 755–762, San Francisco, CA, USA.
Gonzalez, R. C. and Woods, R. E. (2008). Digital Image
Processing. Prentice Hall, 3rd edition.
Greff, K., Brand
˜
ao, A., Krauß, S., Stricker, D., and Clua,
E. (2012). A comparison between background sub-
traction algorithms using a consumer depth camera.
In Proceedings of International Conference on Com-
puter Vision Theory and Applications - VISAPP, vol-
ume 1, pages 431–436, Rome, Italy. SciTePress.
Henry, P., Krainin, M., Herbst, E., Ren, X., and Fox, D.
(2012). Rgb-d mapping: Using kinect-style depth
cameras for dense 3d modeling of indoor environ-
ments. The International Journal of Robotics Re-
search, 31(5):647–663.
Lai, K., Bo, L., Ren, X., and Fox, D. (2011). Sparse dis-
tance learning for object recognition combining rgb
and depth information. In IEEE International Confer-
ence on on Robotics and Automation.
May, S., Droeschel, D., Holz, D., Wiesen, C., and Fuchs, S.
(2008). 3d pose estimation and mapping with time-of-
flight cameras. In IEEE/RSJ International Conference
on Intelligent Robots and Systems (IROS), Workshop
on 3D Mapping, pages 1–6, Nice, France.
Morel, J.-M. and Yu, G. (2009). Asift: A new framework
for fully affine invariant image comparison. SIAM J.
Img. Sci., 2(2):438–469.
Mota, V., Perez, E., Vieira, M., Maciel, L., Precioso, F., and
Gosselin, P. (2012). A tensor based on optical flow
for global description of motion in videos. In Graph-
ics, Patterns and Images (SIBGRAPI), 2012 25th SIB-
GRAPI Conference on, pages 298–301.
Plagemann, C., Ganapathi, V., Koller, D., and Thrun, S.
(2010). Real-time identification and localization of
body parts from depth images. In Proceedings of
the IEEE International Conference on Robotics &
Automation (ICRA), pages 3108–3113, Anchorage,
Alaska, USA.
Quinlan, J. R. (1993). C4.5: programs for machine learn-
ing. Morgan Kaufmann Publishers Inc., San Fran-
cisco, CA, USA.
Schwarz, L. A., Mkhitaryan, A., Mateus, D., and Navab, N.
(2012). Human skeleton tracking from depth data us-
ing geodesic distances and optical flow. Image Vision
Comput., 30(3):217–226.
Shotton, J., Fitzgibbon, A., Cook, M., Sharp, T., Finocchio,
M., Moore, R., Kipman, A., and Blake, A. (2011).
Real-time human pose recognition in parts from single
depth images. In Proceedings of the IEEE Conference
on Computer Vision and Pattern Recognition (CVPR),
pages 1297–1304, Colorado Springs, CO, USA.
Silberman, N. and Fergus, R. (2011). Indoor scene segmen-
tation using a structured light sensor. In Proceedings
of the International Conference on Computer Vision
- Workshop on 3D Representation and Recognition,
pages 601–608.
Stone, E. and Skubic, M. (2011). Evaluation of an inex-
pensive depth camera for passive in-home fall risk as-
sessment. In 5th International Conference on Perva-
sive Computing Technologies for Healthcare (Perva-
siveHealth), 2011, pages 71 –77.
Ye, M., Wang, X., Yang, R., Ren, L., and Pollefeys, M.
(2011). Accurate 3d pose estimation from a single
depth image. In Proceedings of International Confer-
ence on Computer Vision, pages 731–738. IEEE.
M5AIE-AMethodforBodyPartDetectionandTrackingusingRGB-DImages
377