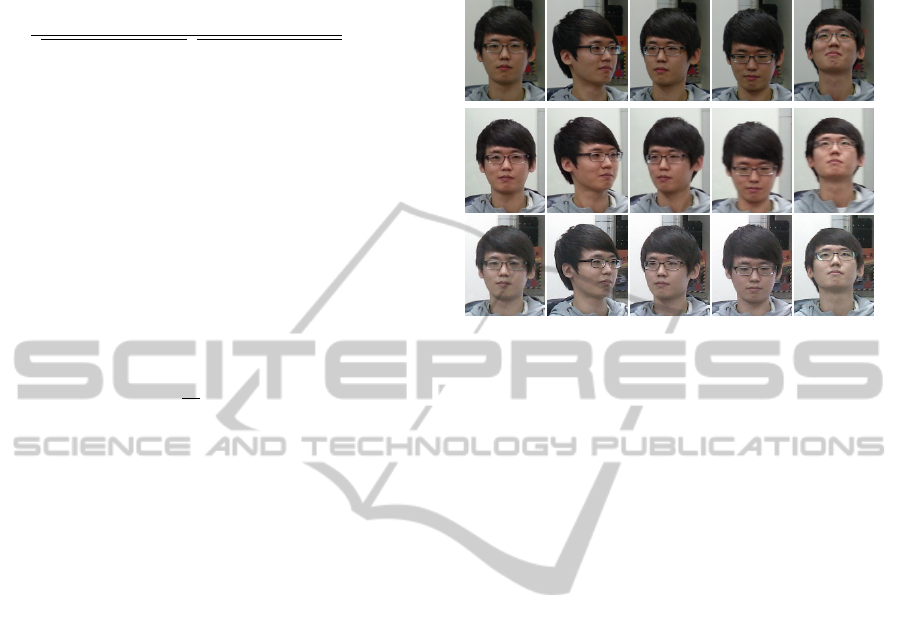
test data set, respectively. Then, the Pearson
product-moment correlation coefficient r between
and
was calculated by
∑
,
,
∑
,
∑
,
∑
,
∑
,
∑
,
∑
,
(5)
where and denote the pixel position and is the
number of pixels. We simplified this as the phi
coefficient (Guilford, 1941) if the bit depth of
and
were 1 (i.e. binary image). However, calculating
the Pearson product-moment correlation coefficient
required high cost operations. Thus, we proposed a
new correlation score method, XNOR+AND
correlation score u, as follows:
,
↓
,
,
∧
,
(6)
where ↓ and ∧ denote the XNOR and
AND operation, respectively. Since the range of
varied between 0 and 2, was normalized by
2
(7)
If the reference image is fixed, this normalization
can be skipped for faster computation. Both the
XNOR and AND operations presented correlations
between two binary images. The XNOR operation
showed information about the overlapping areas of
both the face feature regions (1s) and the non-face
feature regions (0s). On the other hand, the AND
operation considered only the face feature regions.
The non-face feature regions (0s) also provided
information on the overall face shape. However, the
AND operation considered only the facial regions.
On the other hand, the XNOR operation considered
both facial and non-facial regions. However, noise
areas in the facial regions and those in the non-facial
feature regions were treated equally. By using both
the AND operation and the XNOR operation, noise
areas in the non-face feature regions had fewer
influences on the correlation score estimation.
4.4 Head Pose Variation Compensation
Head pose estimation is one of the important issues
in face recognition. 3D model-based methods,
learning-based methods and active appearance
models are frequently used for pose-invariant face
recognition. However, these methods were not
suitable since reference sets continuously vary and
the necessary long processing times may not be
useful with mobile devices.
We assumed that a small amount of head
rotations in yaw and pitch angles can be ignored by
using binary images. Also, a dilation operation was
applied to minimize the small differences in the pose
variations. The proposed XNOR+AND score was
robust against these head pose variations.
(a)
(b) (c) (d) (e)
Figure 5: Cropped facial images taken from original
images of Samsung Galaxy S3 (upper row), Sky Vega X
(middle row) and Samsung NX10 (lower row). (a) frontal
face (b) -15 ~ -45˚ yaw (c) +15 ~ +45˚ yaw (d) -15 ~ -45˚
pitch (e) +15 ~ +45˚ pitch angle tilted.
5 EXPERIMENTS
All the reference images were manually cropped to
6464 pixels. The test image sizes were generally
larger than those of the reference images.
Recognition rates of gray images (8-bit images) with
and without the pre-processing procedures are
shown below for comparison.
Our test database consisted of 135 indoor facial
images taken from two mobile phones and a DSLR
camera. There were nine subjects with five different
head poses, including frontal faces. As shown in Fig.
5, 15 facial images were taken for each person and
they sometimes contained blurred images.
Table 1 shows a performance comparison. Scale
pyramid image registration was applied to all the
methods. The proposed method with the
XNOR+AND similarity measure achieved the best
overall performance (85.93%). The proposed
method also showed the best performance among all
pose variations. With the grayscale images, which
were produced from the RGB images without the
pre-processing procedure, the overall accuracy was
61.48%. When the pre-processing procedure was
used (Tan and Triggs 2007), the overall accuracy
improved to 68.15%. When the Pearson correlation
was used, the overall recognition rate was 83.7%.
VISAPP2014-InternationalConferenceonComputerVisionTheoryandApplications
592