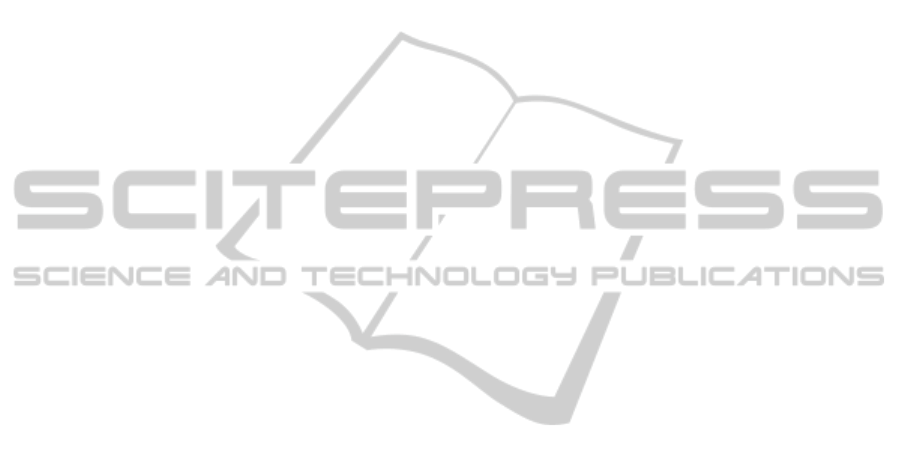
work was proposed that utilizes several visual modal-
ities and contextual constraints to group the occur-
rences of every individual across different videos. Ex-
perimental results on two challenging datasets illus-
trate the effectiveness of usage of multiple modalities.
The use of clothing colors and high-level attributes
demonstrates encouraging results and provides suffi-
cient increase in the performance. Similarly the com-
bination of all modalities (face, high-level attributes,
clothing color) showed promising results. Enhance-
ments have been achieved by enforcing the unique-
ness constraints into the clustering algorithm. The fi-
nal approach that utilizes all modalities and unique-
ness constraints exhibits a clear increase in perfor-
mance for both datasets. Experimental results vali-
date the performance of the proposed framework on
various challenging situations, emphasize on the im-
portance of face pose variations in real life scenarios
and encourage us to strive for better person represen-
tation techniques.
ACKNOWLEDGEMENTS
Authors are thankful to the band Eternal Erection and
other crowd members for allowing us to use their
videos in this research.
REFERENCES
Ahonen, T., Hadid, A., and Pietikainen, M. (2004). Face
Recognition with Local Binary Patterns. In European
Conference on Computer Vision.
Barr, J. R., Bowyer, K. W., and Flynn, P. J. (2011). Detect-
ing questionable observers using face track clustering.
In IEEE Workshop on Applications of Computer Vi-
sion.
Bauml, M., Bernardin, K., Fischer, M., Ekenel, H., and
Stiefelhagen, R. (2010). Multi-pose face recognition
for person retrieval in camera networks. In IEEE In-
ternational Conference on Advanced Video and Signal
Based Surveillance, pages 441–447.
B
¨
auml, M., Tapaswi, M., and Stiefelhagen, R. (2013).
Semi-supervised Learning with Constraints for Person
Identification in Multimedia Data. In IEEE Confer-
ence on Computer Vision and Pattern Recognition.
Bradski, G. (2000). The OpenCV Library. Dr. Dobb’s Jour-
nal of Software Tools.
Chang, C.-C. and Lin, C.-J. (2011). LIBSVM: A library
for support vector machines. ACM Transactions on
Intelligent Systems and Technology, 2.
Cinbis, R. G., Verbeek, J., and Schmid, C. (2011). Un-
supervised Metric Learning for Face Identification in
TV Video. In International Conference on Computer
Vision, Barcelona, Spain.
Comaniciu, D., Ramesh, V., and Meer, P. (2003). Kernel-
based object tracking. IEEE Transactions on Pattern
Analysis and Machine Intelligence, 25(5):564–577.
Cricri, F., Curcio, I. D. D., Mate, S., Dabov, K., and Gab-
bouj, M. (2012). Sensor-based analysis of user gener-
ated video for multi-camera video remixing. In IEEE
18th International Conference on Multimedia Model-
ing, pages 255–265.
Gou, G., Huang, D., and Wang, Y. (2012). A novel video
face clustering algorithm based on divide and conquer
strategy. In Proceedings of the 12th Pacific Rim in-
ternational conference on Trends in Artificial Intelli-
gence, pages 53–63.
Hao, P. and Kamata, S. (2012). Unsupervised people or-
ganization and its application on individual retrieval
from videos. In 21st International Conference on Pat-
tern Recognition, pages 2001–2004.
Klein, D., Kamvar, S. D., and Manning, C. D. (2002). From
instance-level constraints to space-level constraints:
Making the most of prior knowledge in data cluster-
ing. In International Conference on Machine Learn-
ing, pages 307–314, San Francisco, CA, USA.
Kumar, N., Belhumeur, P. N., and Nayar, S. K. (2008).
FaceTracer: A Search Engine for Large Collections
of Images with Faces. In European Conference on
Computer Vision, pages 340–353.
Kumar, N., Berg, A., Belhumeur, P., and Nayar, S. (2011).
Describable visual attributes for face verification and
image search. IEEE Transactions on Pattern Analysis
and Machine Intelligence, 33(10):1962–1977.
Lo Presti, L. and La Cascia, M. (2012). An on-line learning
method for face association in personal photo collec-
tion. Image and Vision Computing, 30(4-5):306–316.
Sivic, J., Zitnick, C. L., and Szeliski, R. (2006). Finding
people in repeated shots of the same scene. In British
Machine Vision Conference.
Suh, B. and Bederson, B. B. (2004). Semi-automatic image
annotation using event and torso identification. Tech-
nical report, Computer Science Department, Univer-
sity of Maryland, College Park, MD.
Tao, J. and Tan, Y.-P. (2008). Efficient clustering of face
sequences with application to character-based movie
browsing. In IEEE International Conference on Image
Processing, pages 1708–1711.
U
ˇ
ri
ˇ
c
´
a
ˇ
r, M., Franc, V., and Hlav
´
a
ˇ
c, V. (2012). Detector of fa-
cial landmarks learned by the structured output SVM.
In Proceedings of the 7th International Conference on
Computer Vision Theory and Applications.
Zhang, L., Chen, L., Li, M., and Zhang, H. (2003). Auto-
mated annotation of human faces in family albums. In
Proceedings of the eleventh ACM international con-
ference on Multimedia, pages 355–358.
WhoistheHero?-Semi-supervisedPersonRe-identificationinVideos
173