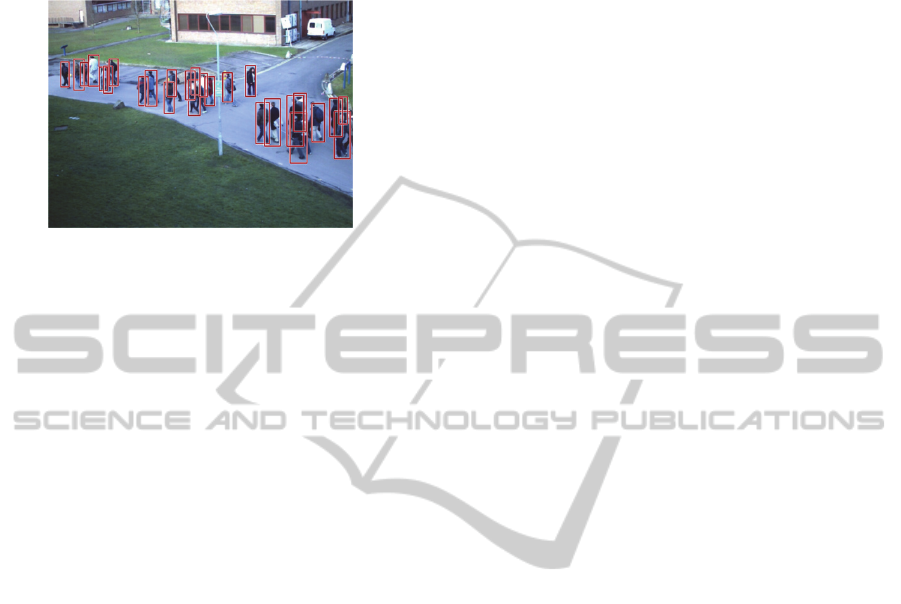
Figure 8 illustrates some examples of the detec-
tion results of our approach on the PETS 2009 data
set when the threshold is set to be 0.
Figure 8: Illustration of the detection results of the pro-
posed approach on the PETS 2009 data set.
For each frame, our proposed approach takes
about 15% more time to calculate the part detection
scores than the full body detector, whereas the com-
putational time for the hypotheses formation and
optimization is quite short and can be neglected. The
VOC 2007 body part model cost more than twice the
time cost by our approach, due to that body part
filters are not well shared among different body part
detectors.
6 CONCLUSIONS
We have developed an approach to adapting the
deformable part based pedestrian detector to crowd-
ed scenes by considering both body part detection
responses and detections' mutual spatial relationship,
without enforcing much additional computational
overhead through part response sharing, while im-
proving the detection results significantly.
Our future wok includes enriching the part detec-
tors with higher discriminability; estimating the
pedestrians' size distribution over the image online
so that size constraint can be enforced on the detec-
tion of future coming frames; designing an efficient
global optimization method that considers both con-
flicts between overlapping visible parts and detec-
tion scores so that decisions can be made more
properly.
ACKNOWLEDGEMENTS
This work is supported by National Natural Science Foun-
dation of China 61202258, Program for New Century
Excellent Talents in University of China NCET-09-0071,
and National Science and Technology Support Program of
China 2013BAK02B01-02.
REFERENCES
Beleznai, C., Bischof, H., 2009. Fast human detection in
crowded scenes by contour integration and local shape
estimation. In CVPR, pp. 2246-2253.
Duan, G., Ai, H., Lao, S., 2010. A Structural Filter Ap-
proach to Human Detection. In ECCV, pp. 238-251.
Dollar, P., Wojek, C., Schiele, B., Perona, P., 2012. Pedes-
trian detection: an evaluation of the state of the art.
TPAMI vol. 34, pp. 743-761.
Felzenszwalb, P. F., Girshick, R. B., McAllester, D., Ra-
manan, D., 2010. Object Detection with Discrimina-
tively Trained Part-Based Models. TPAMI, vol. 32, pp.
1627-1645.
Ge, W., Collins, R., 2009. Marked point processes for
crowd counting. In CVPR, pp. 2913-2920.
Lin, Z., Davis, L. S., Doermann, D., DeMenthon, D., 2007.
Hierarchical part-template matching for human detec-
tion and segmentation. In CVPR, 2007, pp. 1-8.
Ouyang, W., Wang, X., 2012. A discriminative deep mod-
el for pedestrian detection with occlusion handling. In
CVPR, pp. 3258-3265.
Rittscher, J., Tu, P. H., Krahnstoever, N., 2005. Simulta-
neous estimation of segmentation and shape. In CVPR,
pp. 486-493.
Rujikietgumjorn, S., Collins,R. T., 2013. Optimized pe-
destrian detection for multiple and occluded people. In
CVPR.
Shu, G., Dehghan, A., Oreifej, O., Hand, E., Shah, M.,
2012. Part-based multiple-person tracking with partial
occlusion handling. In CVPR, pp. 1815-1821.
Tu, P., Sebastian, T., Doretto, G., Krahnstoever, N.,
Rittscher, J., Yu, T., 2008. Unified crowd segmenta-
tion. In ECCV.
Wang, L., Yung N. H. C., 2012. Three-dimensional mod-
el-based human detection in crowded scenes. TITS,
vol. 13, pp. 691-703.
Wang, X., Han, T., Yan, S., 2009. An HOG-LBP human
detector with partial occlusion handling. In CVPR, pp.
32-39.
Wu, B., Nevatia, R., 2005. Detection of multiple, partially
occluded humans in a single image by Bayesian com-
bination of edgelet part detectors. In CVPR, pp. 90-97.
Yan, J., Lei, Z., Yi, D., Li, S. Z., 2012. Multi-pedestrian
detection in crowded scenes: a global view. In CVPR,
pp. 3124-3129.
Zhao, T., Nevatia, R., 2003. Bayesian human segmenta-
tion in crowded situations. In CVPR, pp. 459-466.
VISAPP2014-InternationalConferenceonComputerVisionTheoryandApplications
604