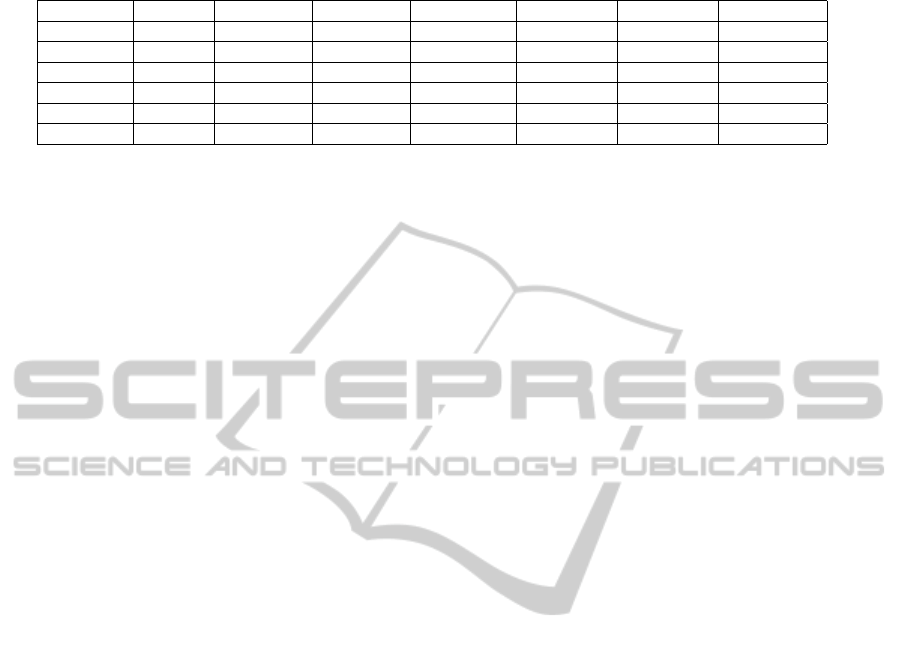
Table 2: Comparison of the 3D CLM, the symmetric and non-rigid and the generic non-rigid reconstruction.
Type 3DCLM Gen (K=1) Gen (K=5) Gen (K=10) Sym (K=1) Sym (K=5) Sym (K=10)
2D Err. 2.73162 2.72951 2.77952 2.78255 2.72853 2.72853 2.72853
2D Impr. 0.0 0.0772 -1.7535 -1.8644 0.1131 0.1131 0.1131
3D Err. 1.03933 0.89338 4.56524 2.50865 0.880928 0.880915 0.880910
3D Impr. 0.0 14.0427 -339.24 -141.37 15.2407 15.2420 15.2425
Pose Err. 0.3443 0.2756 0.5317 0.5974 0.2829 0.2807 0.2908
Pose Impr. 0.0 19.9535 -54.429 -73.5115 17.8332 18.4722 15.5387
reached, however, without the symmetry constraint
this can yield an invalid solution. Our proposed sym-
metric method keeps stable even with a high number
of non-rigid components (K).
One can also see that the head pose error of our
proposed method outperforms the 3D CLM, however,
the generic rigid reconstruction (Gen (K=1)) provides
the best results. We believe that the rigid model can
better fit to the CLM features due to the lack of the
symmetry constraint.
On the other hand the best 3D registration errors
are provided by our proposed method. It shows again
that the symmetry constraint does not allow the re-
construction to converge toward a solution with less
reprojection error, but with a deviated 3D structure.
The table also shows that the 2D registration is
best by our proposed method, however, the gain is
very little and the performance of the methods are ba-
sically similar.
5 CONCLUSIONS
It has been shown in this study that the precision of
the human face pose estimation can be significantly
enhanced if the symmetric (anatomical) property of
the face is considered. The novelty of this paper is
twofold: we have proposed here an improved eye
corner detector as well as a novel non-rigid SfM al-
gorithm for quasi-symmetric objects. The methods
are validated on both real and rendered image se-
quences. The synthetic test were generated by the
basel face model, therefore, ground truth data have
been available for evaluating both our eye corner de-
tector and non-rigid and symmetric SfM algorithms.
The test results have convinced us that the proposed
methods outperforms the compared ones and a precise
head pose estimation is possible for real web-cam se-
quences even if the head is rotated by large angles.
REFERENCES
Arun, K. S., Huang, T. S., and Blostein, S. D. (1987). Least-
squares fitting of two 3-D point sets. PAMI, 9(5):698–
700.
Cootes, T., Taylor, C., Cooper, D. H., and Graham, J.
(1992). Training models of shape from sets of exam-
ples. In BMVC, pages 9–18.
Cootes, T. F., Edwards, G. J., and Taylor, C. J. (1998). Ac-
tive appearance models. In PAMI, pages 484–498.
Springer.
Cristinacce, D. and Cootes, T. F. (2006). Feature detection
and tracking with constrained local models. In BMVC,
pages 929–938.
Hajder, L., Pernek,
´
A., and Kaz
´
o, C. (2011). Weak-
perspective structure from motion by fast alternation.
The Visual Computer, 27(5):387–399.
Harris, C. and Stephens, M. (1988). A combined corner
and edge detector. In Fourth Alvey Vision Conference,
pages 147–151.
Hartley, R. I. and Zisserman, A. (2003). Multiple View Ge-
ometry in Computer Vision. Cambridge University
Press.
He, Z., Tan, T., Sun, Z., and Qiu, X. (2009). Towards ac-
curate and fast iris segmentation for iris biometrics.
PAMI, 31(9):1670–1684.
Jank
´
o, Z. and Hajder, L. (2012). Improving human-
computer interaction by gaze tracking. In Cognitive
Infocommunications, pages 155–160.
Matthews, I. and Baker, S. (2004). Active appearance mod-
els revisited. IJCV, 60(2):135–164.
P. Paysan and R. Knothe and B. Amberg and S. Romdhani
and T. Vetter (2009). A 3D Face Model for Pose and
Illumination Invariant Face Recognition. AVSS.
Pernek,
´
A., Hajder, L., and Kaz
´
o, C. (2008). Metric recon-
struction with missing data under weak perspective. In
BMVC. British Machine Vision Association.
Santos, G. M. M. and Proenc¸a, H. (2011). A robust eye-
corner detection method for real-world data. In IJCB,
pages 1–7. IEEE.
Saragih, J. M., Lucey, S., and Cohn, J. (2009). Face align-
ment through subspace constrained mean-shifts. In
ICCV.
Tan, T., He, Z., and Sun, Z. (2010). Efficient and robust
segmentation of noisy iris images for non-cooperative
iris recognition. IVC, 28(2):223–230.
Tomasi, C. and Kanade, T. (1992). Shape and Motion from
Image Streams under orthography: A factorization ap-
proach. IJCV, 9:137–154.
Viola, P. and Jones, M. (2001). Rapid object detection using
a boosted cascade of simple features. CVPR, 1:I–511–
I–518 vol.1.
Wang, Y., Lucey, S., and Cohn, J. (2008). Enforcing con-
vexity for improved alignment with constrained local
models. In CVPR.
Xiao, J., Chai, J.-X., and Kanade, T. (2004). A Closed-Form
Solution to Non-rigid Shape and Motion Recovery. In
ECCV, pages 573–587.
Precise3DPoseEstimationofHumanFaces
625