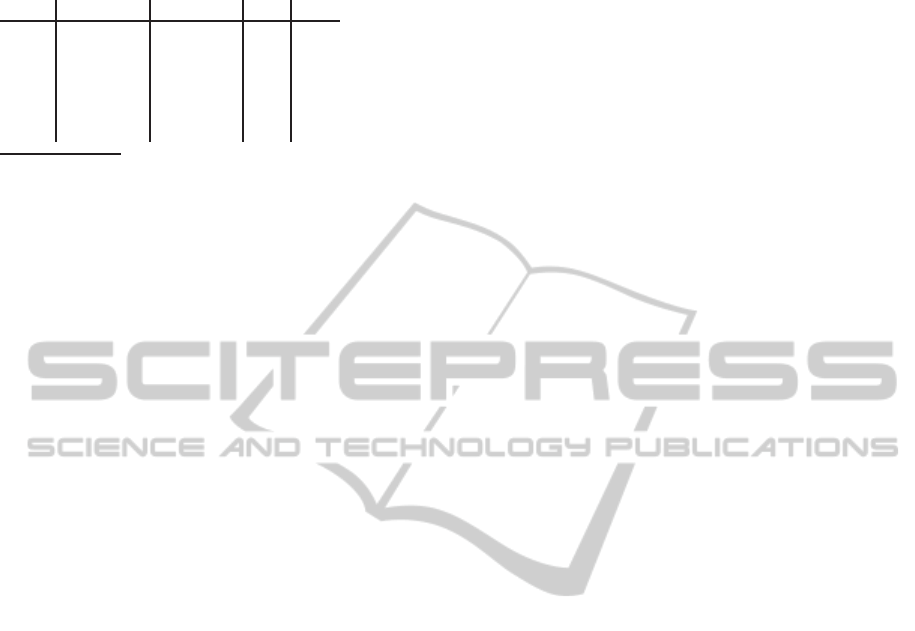
Table 4: Questionnaire and Frequency of the Participants’
Answers with the P-Value of the Wilcoxon Signed Rank
Test.
Score
Q1
a
Q2
b
Q3
c
Q4
d
Q5
e
Q6
f
5 10 19 6 17 11 13
4
13 10 16 12 15 15
3
4 1 3 0 3 1
2
2 0 5 1 1 1
1
1 0 0 0 0 0
P
g
0.012 0.001 N/A N/A
a
How easy is the text-based interface to use
b
How easy is the visual interface to use
c
How helpful is the text-based interface in assigning senti-
ment values
d
How helpful is the visual interface in assigning sentiment
values
e
How useful is the tree cloud in making the task easier
f
How appealing are the visual components
g
P-value of the Wilcoxon signed rank test
7 CONCLUSIONS
Our main contribution in this paper is a novel visual-
ization framework for involving the user in the pro-
cess of generating sentiment lexicon. The visualiza-
tion shows sentiment pairs and allows the user to as-
sign polarity to them. To evaluate the generated lexi-
con, we ran a user study with 30 people. The results
show the extent to which the engagement of the user
in the polarity assignment improves the quality of the
lexicon and this improvement is statistically signifi-
cant. In addition, based on the users’ answers to the
questionnaire at the end of the study, we can say that
the visual interface was preferred by the participants
and it was useful for the polarity assignment task. We
note that polarity assignment for large lexicons is a
very tedious task and we speculate that offering an
improved interface that user’s prefer would like re-
duce fatigue and user disinterest.
As a future work, we would like to add user in-
teractions that enable users to accept or reject the
extracted aspects or even add new aspects that are
missed. In addition, since we needed a gold standard
to evaluate the generated lexicon, we chose a fairly
small dataset. Another way to evaluate the generated
lexicon is to use it in an application such as sentiment
classification and see how much it improves the re-
sults of the classifier. However, this type of evaluation
may add errors of the classifier to the results. Using
larger datasets and evaluating the generated lexicon in
this way is also considered as future work.
ACKNOWLEDGEMENTS
This research was funded by the Boeing Company
and the Natural Sciences and Engineering Research
Council of Canada. We thank Dr. Aminul Islam
for making available his software from (Islam et al.,
2012).
REFERENCES
Annett, M. and Kondrak, G. (2008). A Comparison of Sen-
timent Analysis Techniques: Polarizing Movie Blogs.
In Proceedings of the CSCSI, pages 25–35.
Choi, Y. and Cardie, C. (2009). Adapting a Polarity Lex-
icon Using Integer Linear Programming for Domain-
Specific Sentiment Classification. In Proceedings of
the 2009 Conf. on EMNLP: Volume 2, pages 590–598.
Ding, X., Liu, B., and Yu, P. S. (2008). A Holistic Lexicon-
Based Approach to Opinion Mining. In Proceedings
of the 2008 WSDM, pages 231–240. ACM.
Esuli, A. and Sebastiani, F. (2006). Sentiwordnet: A Pub-
licly Available Lexical Resource for Opinion Mining.
In In Proceedings of LREC, pages 417–422.
Gambette, P. and Veronis, J. (2010). Visualizing a Text with
a Tree Cloud. Studies in Classification, Data Analysis,
and Knowledge Organization, Part 3, pages 561–569.
Gregory, M. L., Chinchor, N., Whitney, P., Carter, R., Het-
zler, E., and Turner, A. (2006). User-Directed Senti-
ment Analysis: Visualizing the Affective Content of
Documents. In Proceedings of the Workshop on Sen-
timent and Subjectivity in Text, pages 23–30. ACL.
Hu, M. and Liu, B. (2004). Mining and Summarizing Cus-
tomer Reviews. In Proceedings of the Tenth ACM
SIGKDD Int. Conf. on KDD, pages 168–177.
Husaini, M., Koc¸yi˘git, A., Tapucu, D., Yanikoglu, B., and
Saygin, Y. (2012). An Aspect-Lexicon Creation and
Evaluation Tool for Sentiment Analysis Researchers.
In Proceedings of the 2012 ECML, pages 804–807.
Islam, A., Milios, E., and Keˇselj, V. (2012). Text Similarity
Using Google Tri-Grams. In Proceedings of Cana-
dian AI’12, pages 312–317.
Li, F., Pan, S. J., Jin, O., Yang, Q., and Zhu, X. (2012).
Cross-Domain Co-Extraction of Sentiment and Topic
Lexicons. In Proceedings of the ACL, pages 410–419.
Lu, Y., Castellanos, M., Dayal, U., and Zhai, C. (2011). Au-
tomatic Construction of a Context-Aware Sentiment
Lexicon: An Optimization Approach. In Proceedings
of the 20th Int.l Conf. on WWW, pages 347–356.
Marneffe, M. D., Maccartney, B., and Manning, C. D.
(2006). Generating Typed Dependency Parses from
Phrase Structure Parses. In LREC, pages 449–454.
Qiu, G., Liu, B., Bu, J., and Chen, C. (2009). Expanding
Domain Sentiment Lexicon Through Double Propa-
gation. In Proceedings of IJCAI, pages 1199–1204.
Qiu, G., Liu, B., Bu, J., and Chen, C. (2011). Opinion
Word Expansion and Target Extraction Through Dou-
ble Propagation. Comput. Linguist., 37(1):9–27.
Context-SpecificSentimentLexiconExpansionviaMinimalUserInteraction
185