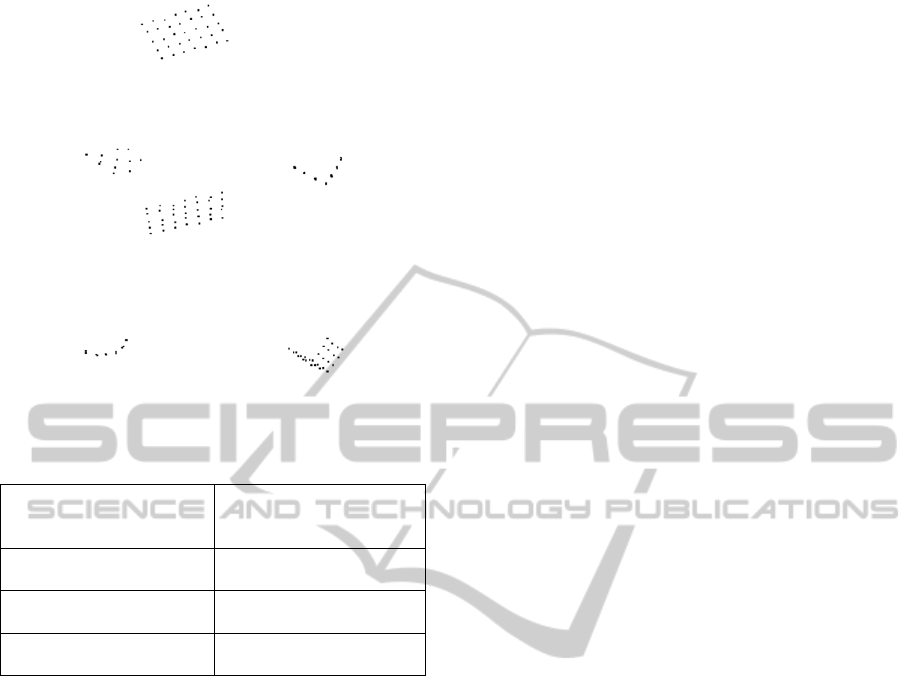
Figure 14: Two views of 3D points (indoor).
Table 3: Optimization results (indoor): reprojection error
and focal length estimation, units for all values are pixel.
RMS reprojection error
1st loop: 59.5622
2nd loop: 1.4326
3rd loop: 0.5576
Initial focal length
estimation
,
(3613.5, 3613.5)
Optimal focal length of
1st camera
(3620.6, 3652.6)
Optimal focal length of
2nd camera
(3646.6, 3410.2)
From the data showed in Table 2 and 3, it can be
known that the final reconstruction result is
reasonably high accurate, whose RMS reprojection
error is about 0.5 pixels. Meanwhile, the non-linear
optimization step converged very fast, which only
used 2 and 3 iterations for outdoor and indoor scenes
respectively. One of the reasons for this fast
convergence is highly accurate initial estimation for
focal length provided by our method.
For conclusion, both for outdoor and for indoor
environments, the new method can provide a close
estimation of focal length, which can dramatically
facilitate the most time-consuming optimization step
of 3D reconstruction.
5 CONCLUSIONS
In this paper, a special planar camera motion, called
SV motion, is introduced to the problem of 3D
reconstruction from two uncalibrated views. An
efficient solution to this problem is proposed. This
solution uses a new method for estimating camera’s
focal length and relative pose. Both synthetic and
real data tests showed that this method could provide
close initial values. As a result, the non-linear
optimization, which is the most time-consuming step
of 3D reconstruction, could converge very fast. In a
conclusion, an efficient solution is achieved to the
problem of 3D reconstruction from two uncalibrated
views under Standard Vergence (SV) constraint.
The main contribution of this paper is that it is
the first time to introduce SV constraint into 3D
reconstruction problem, and an efficient solution
which utilizes this constraint is proposed.
REFERENCES
Alexiadis, D. S., Zarpalas, D. and Daras, P. (2013) Real-
time, full 3-D reconstruction of moving foreground
objects from multiple consumer depth cameras. IEEE
Trans. Multimedia. 15(2):339-358.
Bougnoux, S. (1998) From projective to Euclidean space
under any practical situation, a criticism of self-
calibration. In: Proc. 6th Int. Conf. Comput. Vision,
Bombay, India, 790-796.
Brooks, M. J., de Agaptio, L., Huynh, D. Q. and Baumela,
L. (1998) Towards robust metric reconstruction via a
dynamic uncalibrated stereo head. Image Vision
Comput. 16(14):989-1002.
Goesele, M., Curless, B. and Seitz, S. M. (2006) Multi-
view stereo revisited. In: Proc. 2006 IEEE Comput.
Society Conf. Comput. Vision Pattern Recog. New
York, NY, USA. 2:2403-2409.
Hartley, R. I. (1992) Estimation of relative camera
positions for uncalibrated cameras. In: Proc. 2nd Euro.
Conf. Comput. Vision, Santa Margherita Ligure, Italy,
579-587.
Hartley, R. and Zisserman, A. (2004) Multiple view
geometry in computer vision. 2nd ed. Cambridge:
Cambridge University Press.
Hartley, R. and Li H. (2012) An efficient hidden variable
approach to minimal-cal camera motion estimation.
IEEE Trans. Pattern Anal. Mach. Intell. 34(12):2303-
2314.
Kanatani, K. and Matsunaga, C. (2000) Closed-form
expression for focal lengths from the fundamental
matrix. In: Proc. 4th Asian Conf. Comput. Vision,
Taipei, Taiwan, 1:128-133.
Kanatani, K., Nakatsuji, A. and Sugaya, Y. (2006)
Stabilizing the focal length computation for 3-D
reconstruction from two uncalibrated views. Int. J.
Comput. Vision. 66(2):109-122.
Kitware Inc., Sandia National Laboratories and
Computational Simulation Software, LLC. (2013)
ParaView (Version 4.0.1) [Computer program].
Available from http://www.paraview.org [Accessed 22
Jul. 2013].
Kukelova, Z., Bujnak, M. and Pajdla, T. (2008)
Polynomial eigenvalue solutions to the 5-pt and 6-pt
VISAPP2014-InternationalConferenceonComputerVisionTheoryandApplications
670