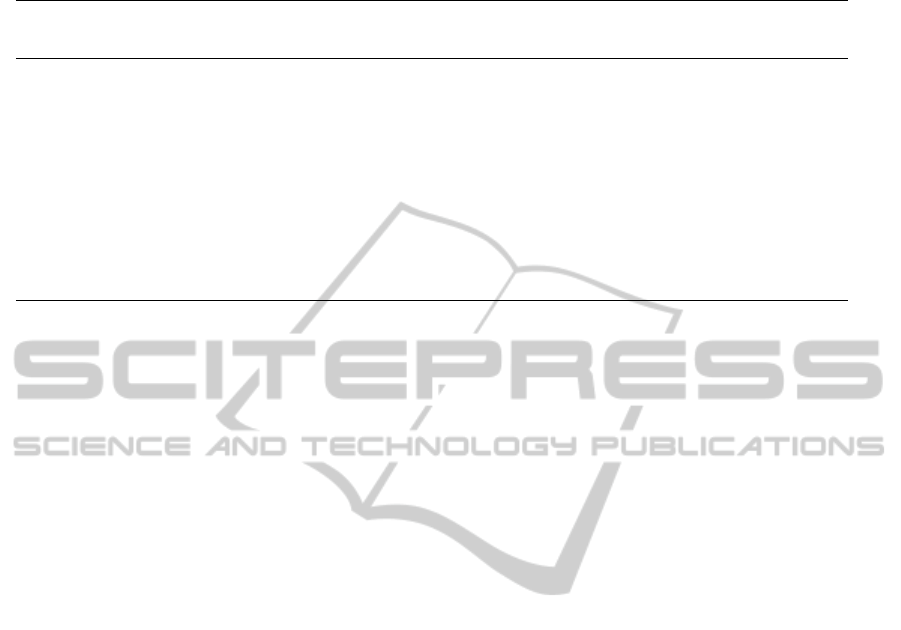
Table 4: Confusion Matrix, in percentage, for concatenated data, where Lying Down
(1)
is lying down (belly up), Lying
Down
(2)
is lying down (right side down) and Lying Down
(3)
is lying down (left side down).
Standing Sitting Walking Running Lying
Down
(1)
Lying
Down
(2)
Lying
Down
(3)
Standing 92.1±3.2 0.0±0.0 0.0±0.0 0.0±0.0 5.4± 2.3 1.3±0.9 1.1±0.8
Sitting 28.3±6.9 68.0±5.9 1.1±0.6 0.3±0.7 0.1± 0.3 1.6±0.7 0.6±1.3
Walking 0.0±0.0 0.4±0.5 99.5±0.5 0.1±0.3 0.0±0.0 0.0±0.0 0.0±0.0
Running 0.0±0.0 0.0±0.0 0.3±0.4 99.4±0.7 0.3±0.4 0.1±0.3 0.0±0.0
Lying Down
(1)
0.9±0.6 2.0±1.1 0.1±0.3 0.0±0.0 82.1±1.9 7.5±1.4 7.4±1.3
Lying Down
(2)
0.0±0.0 0.0±0.0 0.1±0.3 0.5±1.0 1.1±0.0 90.4±0.9 8.0±1.3
Lying Down
(3)
0.0±0.0 0.0±0.0 0.0±0.0 0.0±0.0 0.1±0.3 0.4±0.5 99.5±0.5
The main challenge for future work in this area
will be the development of features and recognition
strategies that can work in an ambient assisted living
under a wide variety of environmental conditions.
REFERENCES
Ankerst, M., Breunig, M. M., Kriegel, H.-P., and Sander, J.
(1999). Optics: ordering points to identify the cluster-
ing structure. SIGMOD Rec., 28(2):49–60.
Bandini, L. G., Gleason, J., Curtin, C., Lividini, K., An-
derson, S. E., Cermak, S. A., Maslin, M., and Must,
A. (2013). Comparison of physical activity between
children with autism spectrum disorders and typically
developing children. Autism, 17(1):44–54.
Bouten, C. V., Koekkoek, K. T., Verduin, M., Kodde, R.,
and Janssen, J. D. (1997). A triaxial accelerometer
and portable data processing unit for the assessment of
daily physical activity. IEEE Transactions on Biomed-
ical Engineering, 44(3):136–147.
Bugan
´
e, F., Benedetti, M. G., Casadio, G., Attala, S., Biagi,
F., Manca, M., and Leardini, A. (2012). Estimation
of spatial-temporal gait parameters in level walking
based on a single accelerometer: Validation on normal
subjects by standard gait analysis. Comput. Methods
Prog. Biomed., 108(1):129–137.
Crockford, D. (2006). The application/json media type for
javascript object notation (json). RFC 4627, IETF.
Donalek, C. (2011). Supervised and Unsupervised Learn-
ing. (April).
Ester, M., peter Kriegel, H., S, J., and Xu, X. (1996).
A density-based algorithm for discovering clusters in
large spatial databases with noise. pages 226–231.
AAAI Press.
Foerster, F. and Fahrenberg, J. (2000). Motion pattern and
posture: correctly assessed by calibrated accelerome-
ters, page 32(3):450457.
Frey, B. J. and Dueck, D. (2007). Clustering by
passing messages between data points. Science,
315(5814):972–976.
Gomes, R., Nunes, N., Sousa, J., and Gamboa, H. (2012).
Long term biosignals visualization and processing. In
BIOSIGNALS, pages 402–405.
Heer, J., Kong, N., and Agrawala, M. (2009). Sizing the
horizon: The effects of chart size and layering on the
graphical perception of time series visualizations. In
ACM Human Factors in Computing Systems (CHI).
I. H. Witten, E. Frank and Hall, M. A. (2011). Data Mining:
Practical Machine Learning Tools and Techniques.
Khan, A. M., Lee, Y.-K., Lee, S. Y., and Kim, T.-
S. (2010). A triaxial accelerometer-based physical-
activity recognition via augmented-signal features and
a hierarchical recognizer. Trans. Info. Tech. Biomed.,
14(5):1166–1172.
Liao, L., Fox, D., and Kautz, H. (2005). Location-based ac-
tivity recognition using relational markov networks.
In Proceedings of the 19th international joint confer-
ence on Artificial intelligence, IJCAI’05, pages 773–
778, San Francisco, CA, USA. Morgan Kaufmann
Publishers Inc.
Liu, A. D. (2013). The Effect of Sensor Mass, Sensor Lo-
cation, and Delamination Location Of. PhD thesis,
California Polytechnic State University.
Lloyd, S. P. (1982). Least squares quantization in pcm.
IEEE Transactions on Information Theory, 28:129–
137.
Mannini, A., Intille, S. S., Rosenberger, M., Sabatini, A. M.,
and Haskell, W. (2013). Activity recognition using a
single accelerometer placed at the wrist or ankle. Med
Sci Sports Exerc.
Mannini, A. and Sabatini, A. (2010). Machine learn-
ing methods for classifying human physical activities
from on-body sensors.
Mathie, M. (2003). Monitoring and interpreting human
movement patterns using a triaxial accelerometer.
Oliphant, T. (2006). Guide to Numpy. Tregol Publishing.
Palmerini, L., Mellone, S., Avanzolini, G., Valzania, F., and
Chiari, L. (2013). Classification of early-mild subjects
with parkinson’s disease by using sensor-based mea-
sures of posture, gait, and transitions. In AIME, pages
176–180.
HumanActivityRecognitionfromTriaxialAccelerometerData-FeatureExtractionandSelectionMethodsforClustering
ofPhysicalActivities
161