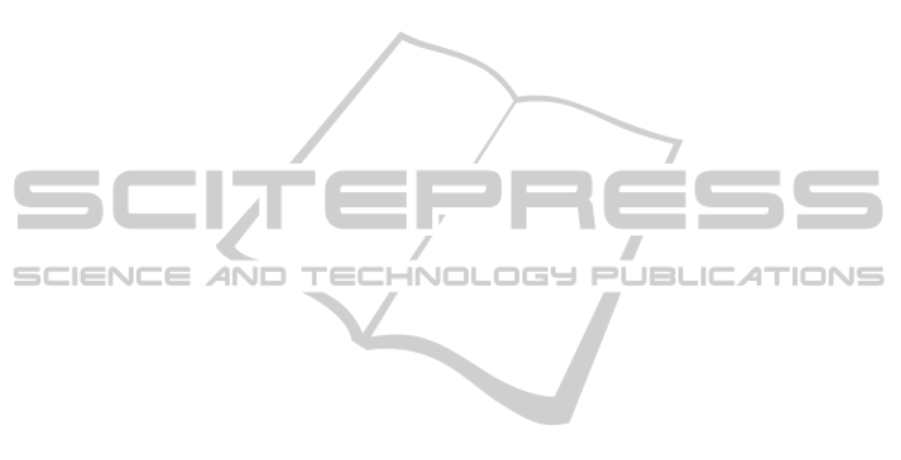
cal structure underlying the approach. It remains that
such procedure now makes tractable the processing of
peptide sequence at a scale compatible with the out-
come of the complete genome sequencing projects,
which should open new opportunities in terms of the
discovery of bioactive peptides.
REFERENCES
Altschul, S. F., Madden, T. L., Sch¨affer, A. A., Zhang,
J., Zhang, Z., Miller, W., and Lipman, D. J. (1997).
Gapped BLAST and PSI-BLAST: a new generation
of protein database search programs. Nucleic Acids
Res, 25(17):3389–402.
Camproux, A., Gautier, R., and Tuff´ery, P. (2004). A hid-
den Markov model derived structural alphabet for pro-
teins. J. Mol. Biol., 339:591–605.
Escoubas, P. and King, G. F. (2009). Venomics as a drug
discovery platform. Expert Review of Proteomics,
6(3):221–224.
Etchebest, C., Benros, C., Hazout, S., and de Brevern, A. G.
(2005). A structural alphabet for local protein struc-
tures: Improved prediction methods. Proteins: Struc-
ture, Function, and Bioinformatics, 59(4):810–827.
Foreman, L. (1992). Generalisation of the Viterbi algo-
rithm. IMA J. Management Math., 4:351–367.
Gellman, S. and Woolfson, D. (2002). Mini-proteins trp the
light fantastic. Nature Structal Biology, 9:408–410.
Hobbs, E., Fontaine, F., Yin, X., and Storz, G. (2011). An
expanding universe of small proteins. Current Opin-
ion in Microbiology, 14:167–173.
Jones, D. (1999). Protein secondary structure prediction
based on position-specific scoring matrices. J. Mol.
Biol., 292:195–202.
Kastenmayer, J. P., Ni, L., Chu, A., Kitchen, L. E., Au, W.-
C., Yang, H., Carter, C. D., Wheeler, D., Davis, R. W.,
Boeke, J. D., Snyder, M. A., and Basrai, M. A. (2006).
Functional genomics of genes withsmall open reading
frames (sORFs) in S. cerevisiae. Genome Research,
16(3):365–373.
Kaur, H., Garg, A., and Raghava, G. (2007). PEPstr: A de
novo method for tertiary structure prediction of small
bioactive peptides. Protein Pept Lett., 14:626–630.
Li, Y. and Zhang, Y. (2009). REMO: A new protocol to
refine full atomic protein models from C-alpha traces
by optimizing hydrogen-bonding networks. Proteins:
Structure, Function, and Bioinformatics, 76(3):665–
676.
Lins, L., Charloteaux, B., Heinen, C., Thomas, A., and
Brasseur, R. (2006). “De Novo” Design of Peptides
with Specific Lipid-Binding Properties. Biophysical
Journal, 90(2):470–479.
Malavolta, L. and Cabral, F. (2011). Peptides: important
tools for the treatment of central nervous system dis-
orders. Neutopeptides, 45:309–316.
Maupetit, J., Derreumaux, P., and Tuff´ery, P. (2009). PEP-
FOLD: an online resource for de novo peptide struc-
ture prediction. Journal of Computational Chemistry,
31:726–738.
Maupetit, J., Derreumaux, P., and Tuff´ery, P. (2010). A fast
method for large-scale de novo peptide and minipro-
tein structure prediction. J Comput Chem, 31(4):726–
38.
Maupetit, J., Tuff´ery, P., and Derreumaux, P. (2007). A
coarse-grained protein force field for folding and
structure prediction. Proteins, 69:394–408.
Park, B. and Levitt, M. (1995). The complexity and accu-
racy of discrete state models of protein structure. J.
Mol. Biol., 249:493–507.
Rabiner, L. (1989). A Tutorial on Hidden Markov Mod-
els and Selected Applications in Speech Recognition.
Proceedings of the IEEE, 77:257–286.
Rohl, C., Strauss, C., Misura, K., and Baker, D. (2004).
Protein structure prediction using Rosetta. Methods in
Enzymology, 383:66–69.
Suzek, B., Huang, H., McGarvey, P., Mazumder, R., and
Wu, C. (2007). UniRef: Comprehensive and Non-
Redundant UniProt Reference Clusters. Bioinformat-
ics, 23:1282–1288.
Th´evenet, P., Shen, Y., Maupetit, J., Guyon, F., Der-
reumaux, P., and Tuff´ery, P. (2012). PEP-FOLD: an
updated de novo structure prediction server for both
linear and disulfide bonded cyclic peptides. Nucleic
Acids Res., 40(Web Server issue):W288–93.
Thomas, A., Deshayes, S., Decaffmeyer, M., Eyck, M. V.,
Charloteaux, B., and Brasseur, R. (2009). PepLook:
an innovative in silico tool for determination of struc-
ture, polymorphism and stability of peptides. Adv Exp
Med Biol., 611:459–460.
Thomas, A., Deshayes, S., Decaffmeyer, M., Van Eyck,
M. H., Charloteaux, B., and Brasseur, R. (2006).
Prediction of peptide structure: How far are we?
Proteins: Structure, Function, and Bioinformatics,
65(4):889–897.
Tuffery, P., Guyon, F., and Derreumaux, P. (2005). Im-
proved greedy algorithm for protein structure recon-
struction. J Comput Chem, 26(5):506–13.
Vetter, I., Davis, J., L.D.Rash, Anangi, R., Mobli, M., Ale-
wood, P., Lewis, R., and King, G. (2011). Venomics:
a new paradigm for natural products-based drug dis-
covery. Amino Acids, 40:15–28.
Viterbi, A. (1967). Error bounds for convolutional codes.
IEEE Trans. Inform. Theory, 13:260–269.
Vlieghe, P., Lisowski, V., Martinez, J., and Khrestchatisky,
M. (2010). Systhetic therapeutic peptides: science and
market. Drug Discovery Today, 15:40–56.
Warren, A. S., Archuleta, J. S., chun Feng, W., and Se-
tubal, J. C. (2010). Missing genes in the annotation of
prokaryotic genomes. BMC Bioinformatics, 11:131.
Wu, S. and Zhang, Y. (2007). LOMETS: A local meta-
threading-server for protein structure prediction. Nu-
cleic Acids Research, 35(10):3375–3382.
Zhang, Y. (2008). I-TASSER server for protein 3D structure
prediction. BMC Bioinformatics, 9(1):40.
Zhang, Y. and Skolnick, J. (2004). SPICKER: A clustering
approach to identify near-native protein folds. Journal
of Computational Chemistry, 25(6):865–871.
BIOINFORMATICS2014-InternationalConferenceonBioinformaticsModels,MethodsandAlgorithms
30