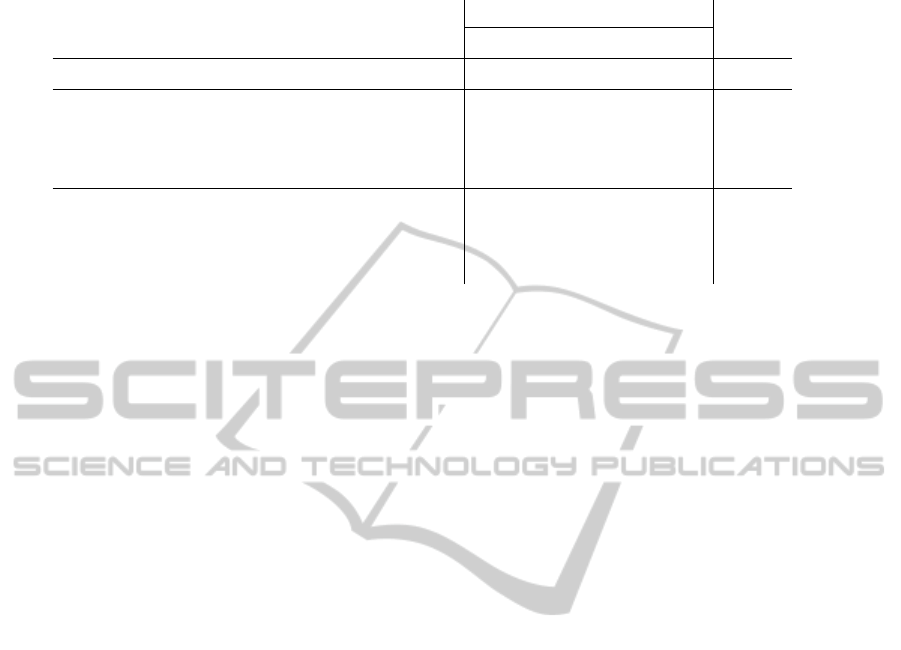
Table 1: Results on MH3DOT tuning versus performance and accuracy. A ‘cup’ sequence and model is used with (partial)
ground truth data. As a reference for these test results we use the BLORT software implementation.
Total Error (952 frames) Time
Roll Pitch Yaw Scale (msec)
BLORT (max = 100) 11.1 11.7 3.5 2.3 141
MH3DOT (LM iter = 10) max hyp: n = 20 14.4 12.9 7.1 2.4 97
max hyp: n = 30 11.2 11.9 5.2 2.2 122
max hyp: n = 50 2.8 5.8 2.4 1.9 158
max hyp: n = 100
2.8 5.8 2.3 1.9 225
MH3DOT (LM iter = 20) max hyp: n = 20 13.9 11.5 6.5 2.1 103
max hyp: n = 30 9.8 7.7 4.4 1.9 179
max hyp: n = 50 2.7 5.8 2.3 1.8 254
max hyp: n = 100 2.7 5.6 2.3 1.8 335
REFERENCES
Azad, P., M
¨
unch, D., Asfour, T., and Dillmann, R. (2011).
6-DoF model-based tracking of arbitrarily shaped 3D
objects. In IEEE Int. Conf. on Robotics and Automa-
tion, pages 5204–5209.
Baltzakis, H. and Argyros, A. (2009). Propagation of pixel
hypotheses for multiple objects tracking. In Advances
in Visual Computing, volume 5876 of Lecture Notes
in Computer Science, pages 140–149.
Chetverikov, D., Stepanov, D., , and Krsek, P. (2005).
Robust euclidean alignment of 3D point sets: the
trimmed iterative closest point algorithm. Image and
Vision Computing, 23:299–309.
Chliveros, G., Pateraki, M., and Trahanias, P. (2013). Ro-
bust multi-hypothesis 3d object pose tracking. In
Computer Vision Systems, volume 7963 of Lecture
Notes in Computer Science, pages 234–243.
Choi, C. and Christensen, H. I. (2012). Robust 3D vi-
sual tracking using particle filtering on the special Eu-
clidean group: A combined approach of keypoint and
edge features. The International Journal of Robotics
Research, 31(4):498–519.
Ciocarlie, M. Household objects database. accessed 19-
July-2012.
Drost, B., Ulrich, M., Navab, N., and Ilic, S. (2010). Model
globally, match locally: Efficient and robust 3d object
recognition. IEEE Transactions on Computer Vision
and Pattern Recognition (CVPR), pages 998 – 1005.
Figueiredo, R., Moreno, P., and Bernardino, A. (2013). Fast
3d object recognition of rotationally symmetric ob-
jects. In Pattern Recognition and Image Analysis,
volume 7887 of Lecture Notes in Computer Science,
pages 125–132.
Fitzgibbon, A. (2003). Robust registration of 2D and
3D point sets. Image and Vision Computing,
21(13):1145–1153.
Franklin, W. (2006). Nearest point query on 184,088,599
points with a uniform grid. Technical report, Rensse-
laer Polytechnic Institute, USA.
Haralick, R. (1994). Propagating covariance in computer
vision. In Proceedings of the 12th IAPR International
Conference on Pattern Recognition, volume 1, pages
493–498.
Harris, C. (1992). Tracking with rigid objects. MIT press.
Johnson, A. E. and Hebert, M. (1999). Using spin images
for efficient object recognition in cluttered 3D scenes.
IEEE Transactions on Pattern Analysis and Machine
Intelligence, pages 433–449.
Koller, D., Daniilidis, K., and Nagel, H. (1993). Model-
based object tracking in monocular image sequences
of road traffic scenes. International Journal of Com-
puter Vision, 10:257–281.
Lourakis, M. (2010). Sparse non-linear least squares opti-
mization for geometric vision. In European Confer-
ence on Computer Vision, pages 43–56.
Mian, A. S., Bennamoun, M., and Owens, R. (2006). Three-
dimensional model-based object recognition and seg-
mentation in cluttered scenes. IEEE Transactions on
Pattern Anal. Mach. Intell, 28:1584–1601.
Muja, M. and Lowe, D. G. (2009). Fast approximate near-
est neighbors with automatic algorithm configuration.
In Int. Conf. on Computer Vision Theory and Applica-
tions (VISAPP), pages 331–340.
Pateraki, M., Sigalas, M., Chliveros, G., and Trahanias, P.
(2013). Visual human-robot communication in social
settings. In IEEE Int. Conf. on Robotics and Automa-
tion.
Puppili, M. and Calway, A. (2006). Real time camera track-
ing using known 3D models and a particle filter. In
IEEE Int. Conf. on Pattern Recognition.
Rousseeuw, P. J. (1984). Least median of squares regres-
sion. Journal of the American Statistical Association,
79(388):871–880.
Wahl, E., Hillenbrand, U., and Hirzinger, G. (2003). Surflet-
pair-relation histograms: A statistical 3D-shape repre-
sentation for rapid classification. 3D Digital Imaging
and Modeling, International Conference on, page 474.
AFrameworkfor3DObjectIdentificationandTracking
677