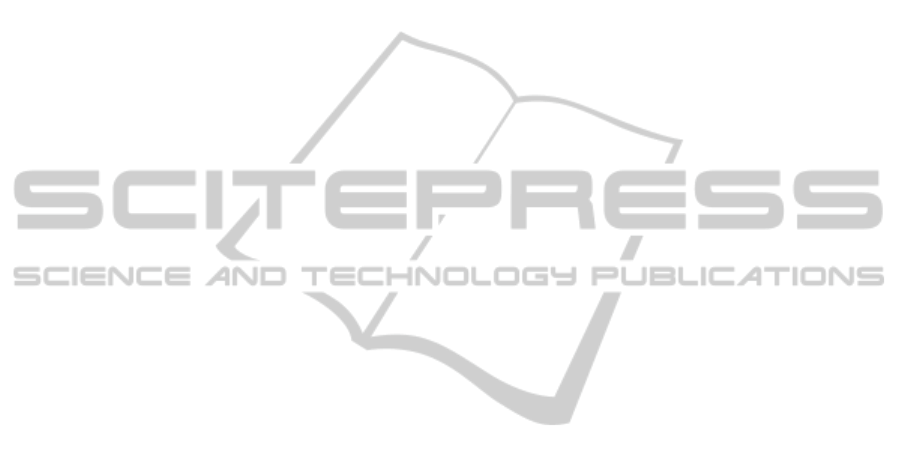
REFERENCES
Carreira, J. and Sminchisescu, C. (2010). Constrained Para-
metric Min-Cuts for Automatic Object Segmentation. In
IEEE International Conference on Computer Vision and
Pattern Recognition.
Chen, N., Zhou, Q.-Y., and Prasanna, V. (2012). Under-
standing web images by object relation network. In Pro-
ceedings of the 21st international conference on World
Wide Web, WWW ’12, pages 291–300, New York, NY,
USA. ACM.
Deschacht, K. and Moens, M.-F. (2007). Text analysis
for automatic image annotation. In Proceedings of the
45th Annual Meeting of the Association of Computa-
tional Linguistics, pages 1000–1007, Prague.
Exner, P. and Nugues, P. (2012). Constructing large propo-
sition databases. In Proceedings of the Eight Interna-
tional Conference on Language Resources and Evalua-
tion (LREC’12), Istanbul.
Fan, R.-E., Chang, K.-W., Hsieh, C.-J., Wang, X.-R., and
Lin, C.-J. (2008). LIBLINEAR: A library for large linear
classification. Journal of Machine Learning Research,
9:1871–1874.
Felzenszwalb, P. F., Girshick, R. B., McAllester, D., and
Ramanan, D. (2010). Object detection with discrimina-
tively trained part based models. IEEE Transactions on
Pattern Analysis and Machine Intelligence, 32(9):1627–
1645.
Gupta, A., Verma, Y., and Jawahar, C. (2012). Choosing
linguistics over vision to describe images. In Proc. of the
twenty-sixth AAAI conference on artificial intelligence.
J
¨
orgensen, C. (1998). Attributes of images in describing
tasks. Information Processing and Management, 34(2-
3):161–174.
Kulkarni, G., Premraj, V., Dhar, S., Siming, L., Choi, Y.,
Berg, A., and Berg, T. (2011). Baby talk: Understand-
ing and generating image descriptions. In Proc. Conf.
Computer Vision and Pattern Recognition.
Ladicky, L., Russell, C., Kohli, P., and Torr, P. H. S. (2010).
Graph cut based inference with co-occurrence statistics.
In Proceedings of the 11th European conference on Com-
puter vision: Part V, ECCV’10, pages 239–253, Berlin,
Heidelberg. Springer-Verlag.
Markkula, M. and Sormunen, E. (2000). End-user search-
ing challenges indexing practices in the digital newspa-
per photo archive. Information retrieval, 1(4):259–285.
Marszalek, M. and Schmid, C. (2007). Semantic hierarchies
for visual object recognition. In Proc. Conf. Computer
Vision and Pattern Recognition.
Moscato, V., Picariello, A., Persia, F., and Penta, A. (2009).
A system for automatic image categorization. In Seman-
tic Computing, 2009. ICSC’09. IEEE International Con-
ference on, pages 624–629. IEEE.
Myeong, H., Chang, J. Y., and Lee, K. M. (2012). Learning
object relationships via graph-based context model. In
CVPR, pages 2727–2734.
Paek, S., Sable, C., Hatzivassiloglou, V., Jaimes, A., Schiff-
man, B., Chang, S., and Mckeown, K. (1999). Integra-
tion of visual and text-based approaches for the content
labeling and classification of photographs. In ACM SI-
GIR, volume 99.
Palmer, M., Gildea, D., and Kingsbury, P. (2005). The
Proposition Bank: an annotated corpus of semantic roles.
Computational Linguistics, 31(1):71–105.
Stamborg, M., Medved, D., Exner, P., and Nugues, P.
(2012). Using syntactic dependencies to solve corefer-
ences. In Joint Conference on EMNLP and CoNLL -
Shared Task, pages 64–70, Jeju Island, Korea. Associ-
ation for Computational Linguistics.
Westman, S. and Oittinen, P. (2006). Image retrieval by end-
users and intermediaries in a journalistic work context. In
Proceedings of the 1st international conference on Infor-
mation interaction in context, pages 102–110. ACM.
Wikipedia (2012). Wikipedia statistics English.
http://stats.wikimedia.org/EN/TablesWikipediaEN.htm.
ICPRAM2014-InternationalConferenceonPatternRecognitionApplicationsandMethods
486