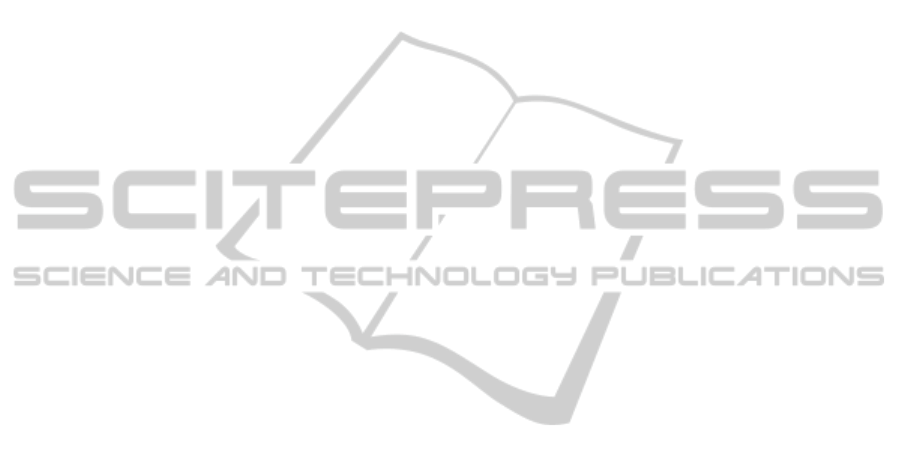
indicate that the likelihood term (1) alone is quite ca-
pable of identifying the most relevant, distinct seg-
mentations.
REFERENCES
Boykov, Y. and Kolmogorov, V. (2004). An experi-
mental comparison of min-cut/max- flow algorithms
for energy minimization in vision. Pattern Analy-
sis and Machine Intelligence, IEEE Transactions on,
26(9):1124–1137.
Boykov, Y., Veksler, O., and Zabih, R. (2001). Fast ap-
proximate energy minimization via graph cuts. Pat-
tern Analysis and Machine Intelligence, IEEE Trans-
actions on, 23(11):1222–1239.
Chen, S., Cao, L., Wang, Y., Liu, J., and Tang, X. (2010).
Image segmentation by map-ml estimations. Image
Processing, IEEE Transactions on, 19(9):2254–2264.
Cui, Y., Fern, X., and Dy, J. (2007). Non-redundant multi-
view clustering via orthogonalization. In Proceedings
of Seventh IEEE International Conference on Data-
Mining (ICDM 2007), pages 133 – 142.
Ghahramani, Z. and Jordan, M. (1997). Factorial hidden
Markov models. Machine Learning, 29(2-3):245–
273.
Hoiem, D., Efros, A., and Hebert, M. (2005). Geometric
context from a single image. In Computer Vision,
2005. ICCV 2005. Tenth IEEE International Confer-
ence on, volume 1, pages 654–661 Vol. 1.
Jaeger, M., Lyager, S. P., Vandborg, M. W., and Wohlge-
muth, T. (2011). Factorial clustering with an ap-
plication to plant distribution data. In Proceed-
ings of the 2nd MultiClust Workshop: Discover-
ing, Summarizing and Using Multiple Clusterings,
pages 31–42. Online proceedings http://dme.rwth-
aachen.de/en/MultiClust2011.
Jain, P., Meka, R., and Dhillon, I. S. (2008). Simultaneous
unsupervised learning of disparate clusterings. Statis-
tical Analysis and Data Mining, 1(3):195–210.
Kato, Z., Pong, T.-C., and Qiang, S. G. (2003). Unsuper-
vised segmentation of color textured images using a
multilayer mrf model. In Proceedings or the IEEE
International Conference on Image Processing (ICIP
2003), volume 1, pages 961–964. IEEE.
Kim, J. and Zabih, R. (2002). Factorial Markov random
fields. In Heyden, A., Sparr, G., Nielsen, M., and Jo-
hansen, P., editors, Computer Vision – ECCV 2002,
volume 2352 of Lecture Notes in Computer Science,
pages 321–334. Springer Berlin Heidelberg.
Kolmogorov, V. and Zabin, R. (2004). What energy func-
tions can be minimized via graph cuts? Pattern Anal-
ysis and Machine Intelligence, IEEE Transactions on,
26(2):147–159.
M¨uller, E., G¨unnemann, S., F¨arber, I., and Seidl, T. (2012).
Discovering multiple clustering solutions: Grouping
objects in different views of the data. In Proceedings
of 28th International Conference on Data Engineer-
ing (ICDE-2012), pages 1207–1210.
Poon, L. K. M., Zhang, N. L., Chen, T., and Wang, Y.
(2010). Variable selection in model-based clustering:
To do or to facilitate. In Proceedings of the 27th In-
ternational Conference on Machine Learning (ICML-
2010, pages 887–894.
Qi, Z. and Davidson, I. (2009). A principled and flexi-
ble framework for finding alternative clusterings. In
Proceedings of the 15th ACM SIGKDD international
conference on Knowledge discovery and data mining
(KDD-09), pages 717–725.
Rother, C., Minka, T., Blake, A., and Kolmogorov, V.
(2006). Cosegmentation of image pairs by histogram
matching-incorporating a global constraint into mrfs.
In Computer Vision and Pattern Recognition, 2006
IEEE Computer Society Conference on, volume 1,
pages 993–1000. IEEE.
Russell, B., Freeman, W., Efros, A., Sivic, J., and Zisser-
man, A. (2006). Using multiple segmentations to dis-
cover objects and their extent in image collections. In
Computer Vision and Pattern Recognition, 2006 IEEE
Computer Society Conference on, volume 2, pages
1605–1614.
Strehl, A. and Ghosh, J. (2003). Cluster ensembles — a
knowledge reuse framework for combining multiple
partitions. J. Mach. Learn. Res., 3:583–617.
MultipleSegmentationofImageStacks
13