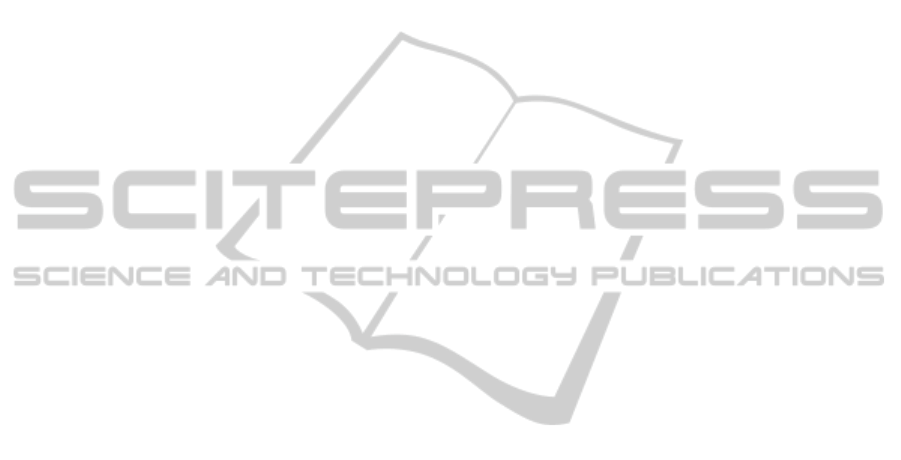
of the part being manufactured. Also, model B
produced good results with a success rate of 96.0 %,
but an increased number of false negatives. This
model had only two inputs (1 AE and 1 VIB).
Nonetheless, the hardware implementation of model
B would be more interesting. Model C, however,
presented low success rate of classification and must
be discarded.
From model A and B, one can be argued that
frequency band of 62 kHz – 68 kHz for AE and 1
kHz – 3 kHz for vibration are good in the feature
extraction for this work.
As discussed in the previous section, the results
of this work have proved superior when compared
with the investigations of (Kwak & Ha 2004) and
(Spadotto et al. 2008). The extraction of the best
signals features from the spectra as well as the use of
vibration signal along with AE produced better
classification results.
Based on one of the best models obtained, it
would be possible to implement it into a hardware
that could provide information in real-time to
operator in order to make adjustments and possibly
avoid burning. In the case of burn classification has
been made, the part should be discarded.
ACKNOWLEDGEMENTS
The authors are indebted to FAPESP, CNPq and
CAPES, Brazilian agencies that have supported this
work. Also, thanks go to the NORTON Company,
from Saint Gobain Group, for donating the wheel.
REFERENCES
Aguiar, P.R., Bianchi, E.C. & Oliveira, J.F.G., 2002. A
method for burning detection in grinding process using
acoustic emission and effective electric power signals.
Manufacturing Systems, 31(3), pp.253–257.
Aguiar, P.R. De, Paula, W.C.F. De & Bianchi, E.C., 2010.
Analysis of Forecasting Capabilities of Ground
Surfaces Valuation Using Artificial Neural Networks.
J. of the Braz. Soc. of Mech. Sci. & Eng., XXXII(2),
pp.146–153.
Ahmadzadeh, F. & Lundberg, J., 2013. Remaining useful
life prediction of grinding mill liners using an artificial
neural network. Minerals Engineering, 53, pp.1–8.
Axinte, D. a et al., 2004. Process monitoring to assist the
workpiece surface quality in machining. International
Journal of Machine Tools and Manufacture, 44(10),
pp.1091–1108.
Babel, R., Koshy, P. & Weiss, M., 2013. Acoustic
emission spikes at workpiece edges in grinding: Origin
and applications. International Journal of Machine
Tools and Manufacture, 64, pp.96–101.
Dimla, Snr., D.E., 2002. The Correlation of Vibration
Signal Features to Cutting Tool Wear in a Metal
Turning Operation. The International Journal of
Advanced Manufacturing Technology, 19(10), pp.705–
713.
Dotto, F. et al., 2003. Automatic detection of thermal
damage in grinding process by artificial neural
network. Revista Escola de Minas, 56(4), pp.295–300.
Hassui, a. et al., 1998. Experimental evaluation on
grinding wheel wear through vibration and acoustic
emission. Wear, 217(1), pp.7–14.
Hassui, a. & Diniz, a. E., 2003. Correlating surface
roughness and vibration on plunge cylindrical grinding
of steel. International Journal of Machine Tools and
Manufacture, 43(8), pp.855–862.
Irani, R. a., Bauer, R.J. & Warkentin, a., 2005. A review
of cutting fluid application in the grinding process.
International Journal of Machine Tools and
Manufacture, 45(15), pp.1696–1705.
Kim, H.. et al., 2001. Process monitoring of centerless
grinding using acoustic emission. Journal of Materials
Processing Technology, 111(1-3), pp.273–278.
Kwak, J.-S. & Ha, M.-K., 2004. Neural network approach
for diagnosis of grinding operation by acoustic
emission and power signals. Journal of Materials
Processing Technology, 147(1), pp.65–71.
Liao, T.W. et al., 2008. Grinding wheel condition
monitoring with boosted minimum distance classifiers.
Mechanical Systems and Signal Processing, 22(1),
pp.217–232.
Liu, Q., Chen, X. & Gindy, N., 2005. Fuzzy pattern
recognition of AE signals for grinding burn.
International Journal of Machine Tools and
Manufacture, 45(7-8), pp.811–818.
Marzi, H., 2008. Modular Neural Network Architecture
for Precise Condition Monitoring. IEEE Transactions
on Instrumentation and Measurement, 57(4), pp.805–
812.
Spadotto, M.M. et al., 2008. Classification of burn degrees
in grinding by neural nets. In IASTED international
conference on artificial intelligence and applications.
Innsbruck: ACTA Press, pp. 1–6.
Teti, R. et al., 2010. Advanced monitoring of machining
operations.
CIRP Annals - Manufacturing Technology,
59(2), pp.717–739.
MonitoringofGrindingBurnbyAEandVibrationSignals
279