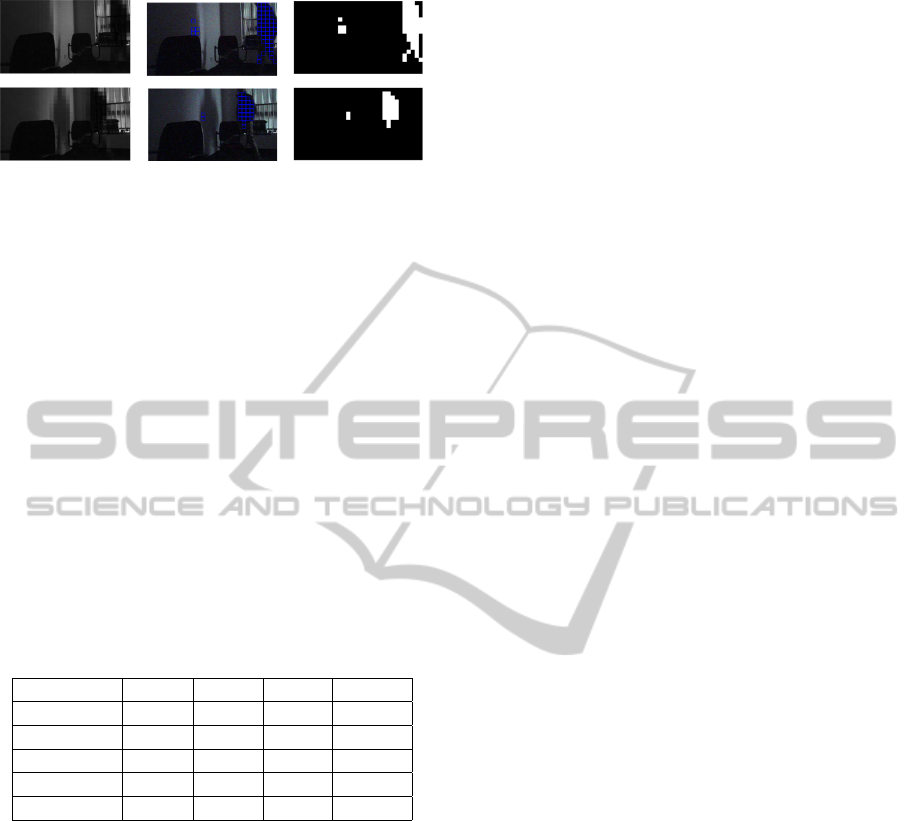
(a) (b) (c)
Figure 10: The experimental results with 0.01-0.05 lx.
Fig. 10(a): The grey scale images of reconstructed im-
ages. Fig. 10(b): The results indicated by the blue boxes.
Fig. 10(c): The results of segmenting the foreground. In or-
der to facilitate observation, the paper increases the bright-
ness of Fig. 10(a) and Fig. 10(b).
small objects suddenly appearing in the background,
the present method can also effectively detect the tar-
get, as shown in Fig. 9. The small objects in the Fig. 9
are a foam cross and a paper box and take about 20
frames to across the whole scene. However, since
the dictionary is learned from the background images
without foreground, the recovered blocks are close to
the background when the colours of foreground and
background are similar. This circumstance increases
the difficulty in detecting, such as the human legs in
the second row of Fig. 10.
Table 1: Detection results under different illumination of
environment and sizes of moving target. The size of the
block is 40 ×40 pixels.
1 lx 0.5 lx 0.1 lx 0.01 lx
5 blocks 100% 100% 40% 20%
10 blocks 100% 90% 70% 60%
30 blocks 89% 85% 83% 70%
50 blocks 86% 90% 80% 68%
100 blocks 85% 88% 79% 76%
In Table 1, we simply census the detection results
under different illumination of environment and sizes
of moving target. The left-most column of the Table
1 presents the number of blocks of the moving object
occupying in the image and the size of the blocks is
40 ×40 pixels. These values are approximation. The
top row in Table 1 shows the different values of illu-
mination. The percent describes the proportion of the
object that the proposed method can detect. We can
find that when the illumination is above 0.5 lx, the
proposed method can detect near 90% of the blocks
of the moving target holding in the image. With the
illumination decreasing to 0.01 lx, it can still iden-
tify about 70% of the blocks while it is difficult for
human vision to distinguish most of them, such as in
Fig. 7. The Table 1 sufficiently reflects the robust of
the proposed method in extreme low illumination en-
vironment.
5 DISCUSSION
Most of the existing motion detection algorithms do
not adequately take into account the extreme low il-
lumination situation. This paper proposes a motion
detection algorithm based on dictionary learning on
video images captured under low light. The experi-
ments show that compared to the mixture of Gaussian
model and the ViBe method, the proposed method
achieves a better detection results even in the case
that human eyes are difficult to distinguish. When
a portion of the moving object is close to the back-
ground, it is difficult to detect this region which is the
inadequacy of this paper. In addition, the paper also
carries out small objects detection under low light ex-
periment. Smaller and faster motion detection in low
illumination can be considered as the future direction
of this work.
ACKNOWLEDGEMENTS
This research was partially supported by National
Science Foundation of China (NSFC) under project
No.61175006 and No.61175015.
REFERENCES
Aharon, M., Elad, M., and Bruckstein, A. (2006). K-svd: an
algorithm for designing overcomplete dictionaries for
sparse representation. Signal Processing, IEEE Trans-
actions on, 54(11):4311–4322.
Barnich, O. and Droogenbroeck, M. V. (2011). Vibe: A
universal background subtraction algorithm for video
sequences. Image Processing, IEEE Transactions on,
20(6):1709–1724.
Chen, S. S., Donoho, D. L., and Saunders, M. A. (1998).
Atomic decomposition by basis pursuit. scientific
computing, 20(1):33–61.
Efron, B., Hastie, T., Johnstone, I., and Tibshirani, R.
(2004). Least angle regression. The Annals of statis-
tics, 32(2):407–499.
Friedman, N. and Russell, S. (1997). Image segmentation
in video sequences: a probabilistic approach. In Pro-
ceedings of the Thirteenth Conference on Uncertainty
in Artificial Intelligence, pages :1232–1245.
Hui, K. C. and Siu, W. C. (2007). Extended analysis
of motion-compensated frame difference for block-
based motion prediction error. Image Processing,
IEEE Transactions on, 16(5):1232–1245.
Lee, H., Battle, A., Raina, R., and Ng, A. (2006). Efficient
sparse coding algorithms. In Advances in neural in-
formation processing systems, pages :801–808.
Mairal, J., Bach, F., and J. Ponce, G. S. (2010). Online
learning for matrix factorization and sparse coding.
Machine Learning Research, 11:19–60.
ALowIlluminationEnvironmentMotionDetectionMethodbasedonDictionaryLearning
153