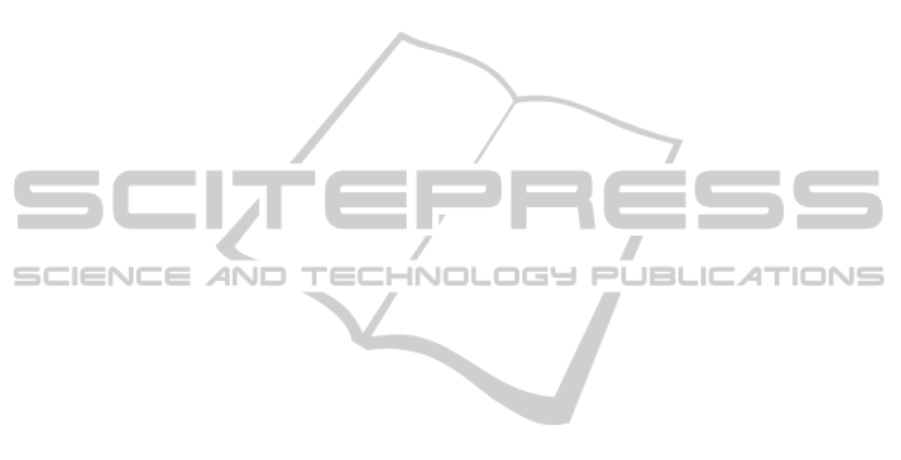
haviours or routines of people at home. The exper-
iments carried out suggest that the approach may be
useful in this scenario thanks to its flexibility, simplic-
ity and robustness.
ACKNOWLEDGEMENTS
This work has been partially supported by the
CIE BioTIC CEI2013-P-3, the Fellow FPU pro-
gram of the Ministry of Education (Spain) AP2010-
6075, the Contrato-Programa 2013-2014 of the
Faculty of Humanities and Education of Ceuta,
and Innoviris, the Brussels Institute for Research
and Innovation through the ICT4Rehab project
(http://www.ict4rehab.org ).
REFERENCES
(2007). Actions as Space-Time Shapes. IEEE Transac-
tions on Pattern Analysis and Machine Intelligence,
29(12):2247–2253.
Azary, S. and Savakis, A. E. (2010). View invariant activity
recognition with manifold learning. volume 6454 of
Lecture Notes in Computer Science, pages 606–615.
Springer.
Berndt, D. J. and Clifford, J. (1994). Using dynamic time
warping to find patterns in time series. In KDD work-
shop, volume 10, pages 359–370. Seattle, WA.
Corradini, A. (2001). Dynamic time warping for off-line
recognition of a small gesture vocabulary. In Recog-
nition, Analysis, and Tracking of Faces and Gestures
in Real-Time Systems, 2001. Proceedings. IEEE ICCV
Workshop on, pages 82–89.
Crandall, A. S. and Cook, D. J. (2010). Using a hidden
markov model for resident identification. In Proceed-
ings of the 6th Int. Conf. on Intelligent Environments,
IE ’10, pages 74–79.
Gao, Q. and Sun, S. (2013a). Human activity recognition
with beta process hidden markov models. In Pro-
ceedings of the International Conference on Machine
Learning and Cybernetics, pages 1–6.
Gao, Q. and Sun, S. (2013b). Trajectory-based human activ-
ity recognition with hierarchical dirichlet process hid-
den markov models. In Proceedings of the 1st IEEE
China Summit and International Conference on Sig-
nal and Information Processing, pages 1–5.
Giles, J. (2010). Inside the race to hack the kinect. New
Scientist, 208(2789):22–23.
Hein, A. and Kirste, T. (2008). Activity recognition for am-
bient assisted living : Potential and challenges. Sen-
sors Peterborough NH, pages 263–268.
Keogh, E., Chakrabarti, K., Pazzani, M., and Mehrotra, S.
(2001). Dimensionality Reduction for Fast Similarity
Search in Large Time Series Databases. Knowledge
and Information Systems, 3(3):263–286.
Laptev, I. (2005). On space-time interest points. Int. J.
Comput. Vision, 64:107–123.
Minhas, R., Baradarani, A., Seifzadeh, S., and
Jonathan Wu, Q. M. (2010). Human action
recognition using extreme learning machine based on
visual vocabularies. Neurocomput., 73:1906–1917.
Mitsa, T. (2010). Temporal Data Mining (Chapman &
Hall/CRC Data Mining and Knowledge Discovery Se-
ries). Chapman and Hall/CRC.
Mokhber, A., Achard, C., and Milgram, M. (2008).
Recognition of human behavior by space-time sil-
houette characterization. Pattern Recognition Letters,
29(1):81–89.
Rabiner, L. and Juang, B. (2003). An introduction to hidden
Markov models. ASSP Magazine, IEEE, 3(1):4–16.
Raheja, J. L., Chaudhary, A., and Singal, K. (2011). Track-
ing of fingertips and centers of palm using kinect. In
Computational Intelligence, Modelling and Simula-
tion (CIMSiM), 2011 Third International Conference
on, pages 248–252. IEEE.
Rantz, M., A., G., A., Oliver, D., M., M., S., J., K., Z., H.,
M., P., G., D., and S., M. (2008). An innovative edu-
cational and research environment. volume 17, page
8491.
Reifinger, S., Wallhoff, F., Ablassmeier, M., Poitschke, T.,
and Rigoll, G. (2007). Static and dynamic hand-
gesture recognition for augmented reality applica-
tions. In Int. Conf. on Human-computer interaction,
HCI’07, pages 728–737. Springer-Verlag.
Salas, J. and Tomasi, C. (2011). People detection using
color and depth images. In Proc. of the 3rd Mexican
conference on Pattern recognition, MCPR’11, pages
127–135. Springer-Verlag.
Shotton, J., Sharp, T., Kipman, A., Fitzgibbon, A., Finoc-
chio, M., Blake, A., Cook, M., and Moore, R. (2013).
Real-time human pose recognition in parts from sin-
gle depth images. Communications of the ACM,
56(1):116–124.
Storf, H., Becker, M., and Riedl, M. (2009). Rule-based ac-
tivity recognition framework: Challenges, technique
and learning. In Pervasive Computing Technologies
for Healthcare, 2009. PervasiveHealth 2009. 3rd In-
ternational Conference on, pages 1–7.
Xia, L., Chen, C.-C., and Aggarwal, J. K. (2012). View
invariant human action recognition using histograms
of 3d joints. In CVPR Workshops, pages 20–27. IEEE.
Yang, X. and Tian, Y. (2012). Eigenjoints-based action
recognition using naive-bayes-nearest-neighbor. In
Computer Vision and Pattern Recognition Workshops
(CVPRW), 2012 IEEE Computer Society Conference
on, pages 14–19. IEEE.
Yang, X. and Tian, Y. (2013). Effective 3d action recogni-
tion using eigenjoints. Journal of Visual Communica-
tion and Image Representation.
Yang, X., Zhang, C., and Tian, Y. (2012). Recognizing ac-
tions using depth motion maps-based histograms of
oriented gradients. In Babaguchi, N., Aizawa, K.,
Smith, J. R., Satoh, S., Plagemann, T., Hua, X.-S., and
Yan, R., editors, ACM Multimedia, pages 1057–1060.
ACM.
ICPRAM2014-InternationalConferenceonPatternRecognitionApplicationsandMethods
494