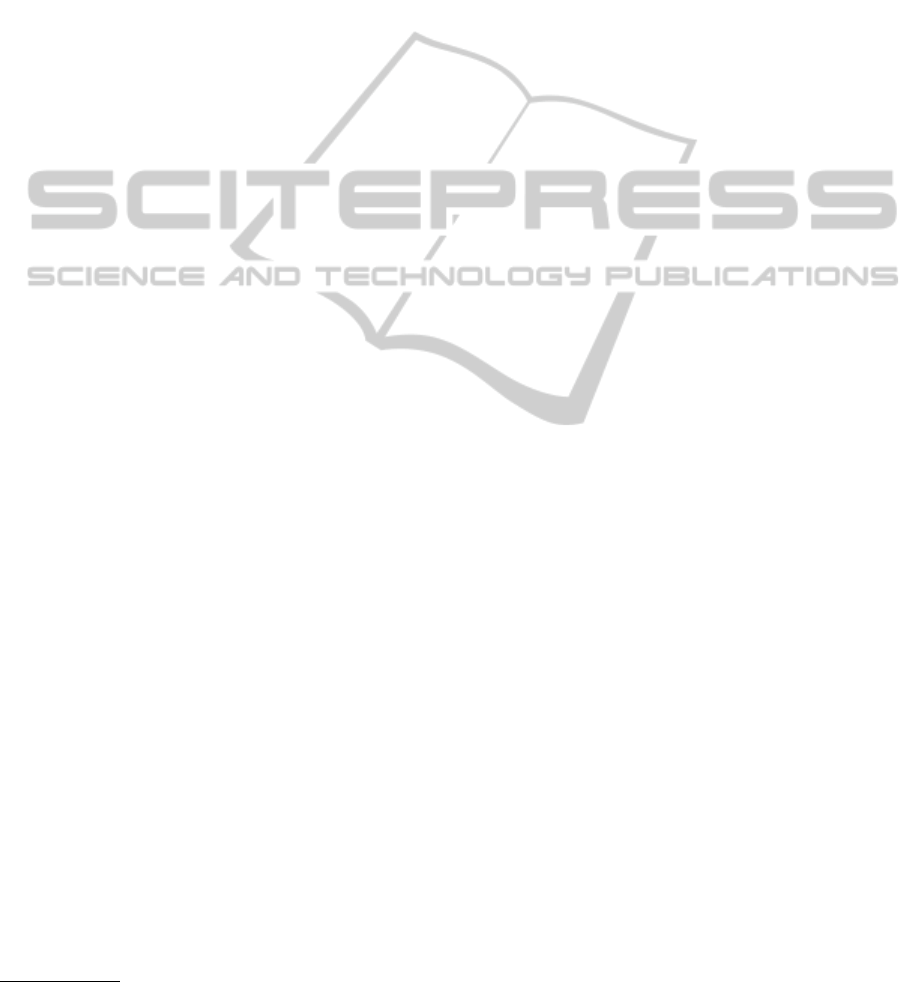
Reference) for modeling people and things perspec-
tive variability. The Activiti Designer is an open-
source and light-weight Business Process Manage-
ment (BPM) platform which offers among other fea-
tures a powerful designer and a solid BPMN2 pro-
cess engine. Clafer is also a lightweight modeling
language which enables to express variability among
products and systems.
Following the presented approach, we model the
variability of an elevators remote predictive main-
tenance and monitoring process in an SGB domain
using two separated variation models and techniques
(In-PP, In-TP and SharedBy): one for people pers-
pective (e.g. maintenance contracts) and the other for
things perspective (e.g. elevator’s modules - door and
pulley) variability modeling.
8
Overall, our prototype shows encouraging results
to cope with complex large models, by adopting base
models, variation models (representing things and
people perspective separately) and process fragments
for representing multi-perspective process variability.
However, it is restricted in the sense that it purely fo-
cuses on the control-flow.
5 CONCLUSIONS
Likewise in SPLE, process variability needs to con-
sider the multiple dimensions coming from smart en-
vironments, i.e., it needs to support the multiplicity of
stakeholders, devices and data derived from process-
intensive applications. This premise is the underly-
ing idea of our paper that presents a novel approach
for supporting multi-perspective process variability
by using a fragment-based re-use approach. After dis-
cussing the impact of multiple dimensions in SGBs
with respect to multi-perspective process variability,
we pointed out the related work and also described
a methodology by means of a meta-model for sup-
porting multi-perspective process variability adopting
a fragment-based re-use approach.
In our future work we plan to consider an au-
tomated resolution of multi-perspective process va-
riability by processing over context data streams, as
well as evaluating the presented perspective resolu-
tion strategies against different modeling methods.
REFERENCES
Baresi, L., Guinea, S., and Pasquale, L. (2012). Service-
Oriented Dynamic Software Product Lines. IEEE
8
Detailed information at:
http://aitormurguzur.com/projects/lateva/multi-perspective
Computer, 45(10):42–48.
Bayer, J., G
´
erard, S., Haugen, O., Mansell, J., Moller-
Pedersen, B., Oldevik, J., Tessier, P., Thibault, J., and
Widen, T. (2006). Consolidated product line variabi-
lity modeling. In SPLC.
Berger, T., Rublack, R., Nair, D., Atlee, J. M., Becker, M.,
Czarnecki, K., and Wasowski, A. (2013). A Survey of
Variability Modeling in Industrial Practice. In VaMoS.
Castro, M., Jara, A. J., and Skarmeta, A. F. (2012). An anal-
ysis of m2m platforms: Challenges and opportunities
for the internet of things. In IMIS.
Classen, A., Hubaux, A., and Heymans, P. (2009). A for-
mal semantics for multi-level staged configuration. In
VaMoS.
Czarnecki, K., Helsen, S., and Eisenecker, U. (2004).
Staged configuration using feature models. In SPLC.
Garc
´
ıa-Gal
´
an, J., Trinidad, P., and Ruiz-Cort
´
es, A. (2013).
Multi-user variability configuration: A game theoretic
approach. In ASE.
Gurgen, L., Gunalp, O., Benazzouz, Y., and Gallissot, M.
(2013). Self-aware cyber-physical systems and appli-
cations in smart buildings and cities. In DATE.
Hallerbach, A., Bauer, T., and Reichert, M. (2010). Cap-
turing Variability in Business Process Models : The
Provop Approach. Software Maintenance and Evolu-
tion, 22(6-7):519–546.
Hubaux, A., Heymans, P., Schobbens, P.-Y., Deridder, D.,
and Abbasi, E. (2011). Supporting multiple perspecti-
ves in feature-based configuration. Software and Sys-
tems Modeling, pages 1–23.
Kolb, J. and Reichert, M. (2013). A flexible approach for
abstracting and personalizing large business process
models. Applied Computing Review, 13(1):6–17.
Meerkamm, S. (2012). Staged configuration of multi-
perspectives variants based on a generic data model.
In BPM Workshops.
Mendonc¸a, M., Cowan, D., Malyk, W., and Oliveira, T.
(2008). Collaborative Product Configuration: For-
malization and Efficient Algorithms for Dependency
Analysis. Journal of Software, 3(2):69–82.
Murguzur, A., Sagardui, G., Intxausti, K., and Trujillo, S.
(2013). Process variability through automated late se-
lection of fragments. In CAiSE Workshops.
Rosa, M. L., Dumas, M., ter Hofstede, A. H., and
Mendling, J. (2011). Configurable multi-perspective
business process models. Information Systems :
Databases : Their Creation, Management and Utiliza-
tion, 36(2):313–340.
Rosenm
¨
uller, M., Siegmund, N., Th
¨
um, T., and Saake, G.
(2011). Multi-dimensional variability modeling. In
VaMoS.
Schnieders, A. and Puhlmann, F. (2006). Variability mech-
anisms in e-business process families. In BIS.
Schroeter, J., Lochau, M., and Winkelmann, T. (2012).
Multi-perspectives on feature models. In MODELS.
Wang, K.-Y., Lin, G., Chou, P., and Chou, A. (2012). Lever-
age smart system services technology for smart green
building management. In EGG.
MODELSWARD2014-InternationalConferenceonModel-DrivenEngineeringandSoftwareDevelopment
554