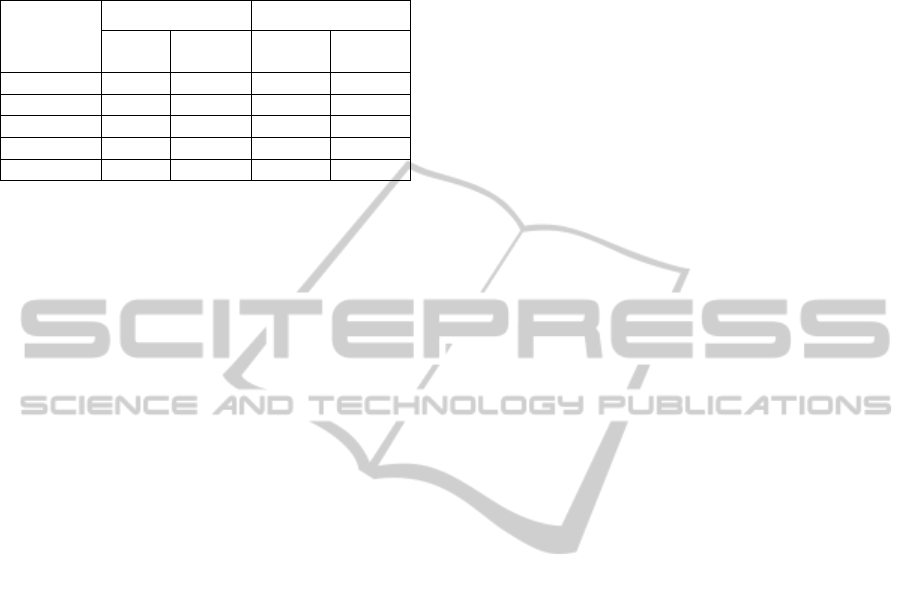
different methods for dealing with high dimensional
data.
Table 3: Experimental results for two real datasets.
Method
Iris Yeast
Time
FM-
Index
Time
FM-
Index
Single
0.020 0.7635 9.929 0.4700
Complete
0.015 0.7686 10.091 0.3160
Ward’s
0.018 0.8222 10.248 0.2689
PHA
0.020 0.8670 1.439 0.4694
TTHC
0.020 0.9234 1.468 0.4731
ACKNOWLEDGEMENTS
This work is supported by the National Science
Foundation of China (Grants No. 61272213).
REFERENCES
Assent, I., Clustering high dimensional data, WIREs Data
Mining and Knowledge Discovery, 2: 340–350, 2012.
Endo, Y., Iwata, H., Dynamic clustering based on
universal gravitation model, Modeling Decisions for
Artificial Intelligence, Lecture Notes in Computer
Science, 3558: 183–193, 2005.
Filippone, M., Camastra, F., Masulli, F., Rovetta, S., A
survey of kernel and spectral methods for clustering,
Pattern Recognition, 41(1): 176-190, 2008.
Fowlkes, E.B., Mallows, C.L., A method for comparing
two hierarchical clusterings, Journal of the American
Statistical Association, 78: 553-569, 1983.
Frank, A., Asuncion, A., UCI Machine Learning
Repository [http://archive.ics.uci.edu/ml], 2010.
Irvine, CA: University of California, School of
Information and Computer Science.
Gil-García, R., Pons-Porrata, A., Dynamic hierarchical
algorithms for document clustering, Pattern
Recognition Letters, 31: 469–477, 2010.
Gómez, J., Dasgupta, D., Nasraoui, O., A new
gravitational clustering algorithm, In Proceedings of
the 3rd SIAM International Conference on Data
Mining, pages 83–94, San Francisco, CA, USA, May
1-3, 2003.
Jain, A.K., Data clustering: 50 years beyond K-means,
Pattern Recognition Letters, 31: 651–666, 2010.
Li, J., Fu, H., Molecular dynamics-like data clustering
approach, Pattern Recognition, 44: 1721-1737, 2011.
Lu, Y., Wan, Y., PHA: a fast potential-based hierarchical
agglomerative clustering method, Pattern Recognition,
46(5): 1227–1239, 2013.
Lu, Y., Wan, Y., Clustering by sorting potential values
(CSPV): a novel potential-based clustering method,
Pattern Recognition, 45(9): 3512–3522, 2012.
Murtagh, F., Contreras, P., Algorithms for hierarchical
clustering: an overview, WIREs Data Mining and
Knowledge Discovery, 2: 86–97, 2012.
Omran, M.G., Engelbrecht, A.P., Salman, A., An
overview of clustering methods, Intelligent Data
Analysis, 11(6): 583-605, 2007.
Peng, L., Yang, B., Chen, Y., Abraham, A., Data
gravitation based classification. Information Sciences,
179(6): 809-819, 2009.
Shi, S., Yang, G., Wang, D., Zheng, W., Potential-based
hierarchical clustering, In Proceedings of the 16th
International Conference on Pattern Recognition,
pages 272-275, Quebec, Canada, August 11-15, 2002.
Wang, J., Li, M., Chen, J., Pan, Y., A fast hierarchical
clustering algorithm for functional modules discovery
in protein interaction networks, IEEE Transactions on
Computational Biology and Bioinformatics, 8(3): 607-
620, 2011.
Wright, W.E., Gravitational clustering, Pattern
Recognition, 9: 151-166, 1977.
Yu, H., Gerstein, M., Genomic analysis of the hierarchical
structure of regulatory networks, Proc. National
Academy of Sciences of USA, 103(40): 14724–14731,
October, 2006.
MeasuringClusterSimilaritybytheTravelTimebetweenDataPoints
19