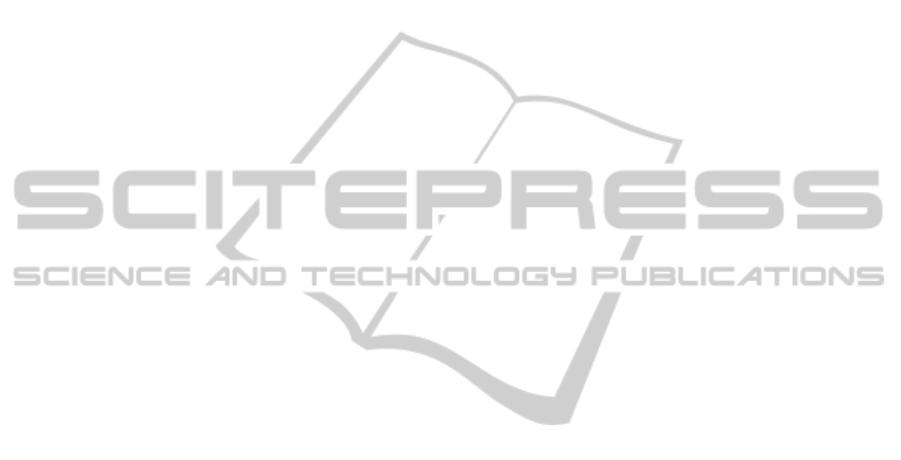
REFERENCES
Adolphs, R., Tranel, D., and Damasio, A. R. (2003). Disso-
ciable neural systems for recognizing emotions. Brain
and Cognition, 52(1):61–69.
Alves, N. T., Fukusima, S. S., and Aznar-Casanova, J. A.
(2008). Models of brain asymmetry in emotional pro-
cessing. Psychology & Neuroscience, 1(1):63–66.
Arnold, M. (1950). An Excitatory Theory of Emotion.
McGraw-Hill, New York, xxiii edition.
Arvaneh, M., Guan, C., Ang, K. K., and Quek, C. (2011).
Optimizing the channel selection and classification
accuracy in eeg-based bci. IEEE Transactions on
Biomedical Engineering, 58(6):1865–1873.
Borod, J. C. (1992). Interhemispheric and intrahemispheric
control of emotion: a focus on unilateral brain dam-
age. J Consult Clin Psychol, 60(3):339–348.
Chu, C., Hsu, A.-l., Chou, K.-h., Bandettini, P., and Lin, C.
(2012). NeuroImage Does feature selection improve
classification accuracy? Impact of sample size and
feature selection on classification using anatomical
magnetic resonance images. NeuroImage, 60(1):59–
70.
Constantinou, E., Bogaerts, K., Diest, L. V., and den Bergh,
O. V. (2013). Inducing symptoms in high symptom re-
porters via emotional pictures: The interactive effects
of valence and arousal. Journal of Psychosomatic Re-
search, 74(3):191–196.
Darwin, C. (1872). The Expression of the Emotions in Man
and Animals, with an Introduction, Afterword, and
Commentaries by Paul Ekman. Oxford Univ Press,
Oxford, 3rd edition.
Heller, W. (1993). Neuropsychological mechanisms of
individual differences in emotion, personality, and
arousal. Neuropsychology, 7,:476–489.
Holland, J. H. (1975). Adaptation in Neural and Artificial
Systems. University of Michigan Press, Ann Arbor,
Michigan, 1st edition.
Hosseini, S. A. (2011). Emotion recognition method using
entropy analysis of eeg signals. International Journal
of Images, Graphics, and Signal Processing, 3(5):30–
36.
Jolliffe, I. T. (2002). Principal Component Analysis.
Springer,, 2nd edition.
Katsis, C., Katertsidis, N., and Fotiadis, D. (2011). An in-
tegrated system based on physiological signals for the
assessment of affective states in patients with anxiety
disorders. Biomedical Signal Processing and Control,
6:261–268.
Lang, P. J., Bradley, M. M., and Cuthbert, B. N. (2008). In-
ternational affective picture system (iaps): Technical
manual and affective ratings. Technical Report A-8.
Liu, J. and Xu, J. (2010). Compared study of the analyzing
methods for eeg data. In Proc. of 3rd IEEE Interna-
tional Conference on Computer Science and Informa-
tion Technology, volume 9, pages 445–448.
Morlet, J. (1984). Decomposition of hardy functions into
square integrable wavelets of constant shape. SIAM.
Math. Anal, 15(4):723–736.
Murugappan, M., Ramachandran, N., and Sazali, Y. (2010).
Classification of human emotion from eeg using dis-
crete wavelet transform. Journal of Biomedical Sci-
ence and Engineering, 3:390–396.
Murugappan, M., Rizon, M., Nagarajan, R., Yaacob, S., Zu-
naidi, I., and Hazry, D. (2007). Eeg feature extraction
for classifying emotions using fcm and fkm. Inter-
national Journal of Computers and Communications,
1(2):299–304.
Nardi, D. (2011). Neuroscience of Personality: Brain Savvy
Insights for All Types of People. Radiance House, 1.0
edition.
Picard, R. W., Vyzas, E., and Healey, J. (2001). Toward
machine emotional intelligence: analysis of affective
physiological state. IEEE Transactions on Pattern
Analysis and Machine Intelligence, 23(1):1175–1191.
Russell, J. (1980). A circumplex model of affect. Journal of
Personality and Social Psychology, 39(6):1161–1178.
Salovey, P. and Rothman, A. J. (2000). Emotional states
and physical health. The American psychologist,
55(1):110–121.
Schaaff, K. and Schultz, T. (2009). Towards emotion recog-
nition from electroencephalography signals. In 3rd
International Conference on Affective Computing and
Intelligent Interaction. Institute of Electrical and Elec-
tronics Engineers.
Sherwood, J. (2009). On classifiability of wavelet fea-
tures for eeg-based brain-computer interfaces. In Pro-
ceedings of International Joint Conference on Neural
Networks. Institute of Electrical and Electronics Engi-
neers.
Sirois, B. C. (2003.). Negative emotion and coronary heart
disease. Behavior Modification, 27(1):83–102.
Sivakumar, A. and .Kannan, K. (2009). A novel feature
selection technique for number classification problem
using pnn - a plausible scheme for boiler flue gas anal-
ysis. Sensors and Actuators B: Chemical, 139:280–
286.
Specht, D. F. (1990). Probabilistic neural networks. Neural
Networks, 3:110–118.
Tahon, M., Degottex, G., and Devillers, L. (2012). Usual
voice quality features and glottal features for emo-
tional valence detection. In the 6th International Con-
ference of Speech Prosody. Tongji University Press.
Takahashi, K. and Tsukaguchi, A. (2003). Remarks on emo-
tion recognition from multi-modal bio-potential sig-
nals. In Proc. of IEEE International Conference on
Systems, Man, and Cybernetics. Institute of Electrical
and Electronics Engineers.
Van den Broek, E. L. and Westerink, J. H. D. M. (2009).
Considerations for emotion-aware consumer products.
Applied ergonomics, 40(6):1055–64.
Wundt, W. (1905). Grundriss der Psychologie [Fundamen-
tals of Psychology]. Engelman, Leipzig, 7th edition.
EmotionalValenceDetectionbasedonaNovelWaveletFeatureExtractionStrategyusingEEGSignals
59