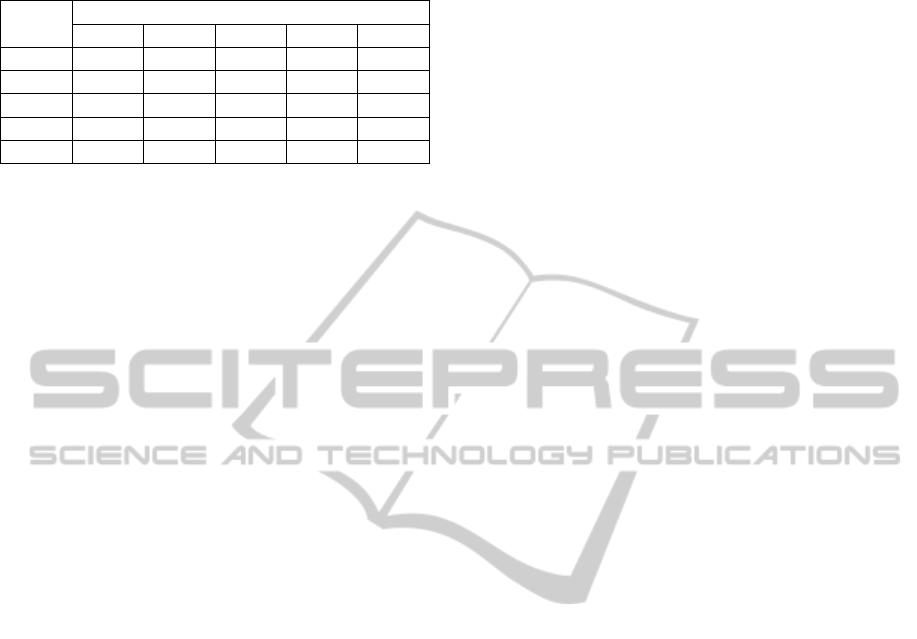
Table 5: Finger motion recognition confusion matrix of
improved recognition methodology (IED = 50 mm).
Motion Accuracy (%)
Thumb Index Middle Ri&Li Rest
Thumb
81.3
13.8 1.2 3.7 0
Index 5
91.3
0 2.5 1.2
Middle 0 0
92.5
7.5 0
Ri&Li 6.2 2.5 20
71.3
0
Rest 0 0 0 0
100
The two new features (SFR and SN) and the
mRMR together contributed to a 1.3% increase in
accuracy (87.3% compared to 86%), which is a
relatively small improvement. However, as noted in
Table 2, the mRMR ranks the SN and SFR in 1st
and 4th
place, respectively, showing they are very
effective features of the sEMG signals for finger
motion recognition. Since we did not normalize the
amplitude of the signals, SFR and SN can have a
robust performance regarding the individual differ-
ences because they are not related to the amplitude
information.
However, although the recognition results by
adopting the mRMR show that only AR4 should be
eliminated, it also indicates that if we do not need to
have the highest level of accuracy, a more compact
feature set can be selected (SN, AR1, RMS, SFR,
WL, and AR2), resulting in an accuracy of 84%.
This result shows us that by adopting the mRMR,
we can determine a relatively suitable feature set
that can significantly reduce the computing time
with only a slight decrease in accuracy.
8 CONCLUSIONS
We proposed a wearable sensing method based on
the muscle’s crosstalk information that uses only one
sEMG channel to recognize five basic finger mo-
tions (thumb flexion, index flexion, middle flexion,
ring & little flexion, and rest position) related to
daily human activities. A suitable inter-electrode
distance was defined (50 mm) from the inter-
electrode distance experiment to improve the accu-
racy. In addition, two new features were proposed
and a feature selection method was adopted, result-
ing in an accuracy of 87.3% compared to 81.5%
when using the conventional methodology with an
IED of 30 mm. Our results show that the improved
recognition methodology is not only effective for
detecting finger motions, but also is insensitive to
individual differences.
The recognition methodology still needs im-
provement. The effectiveness of our methodology in
recognizing other motions besides the five basic
motions should also be reexamined. As for its appli-
cation, we need to adopt the wearable sensing meth-
od and the improved recognition methodology for
recognizing daily human activities like typing, read-
ing, writing, and using a mobile phone.
REFERENCES
Al-Timemy, A., Bugmann, G., Escudero, J., and Outram,
N., 2013. Classification of finger movements for the
dexterous hand prosthesis control with surface elec-
tromyography. IEEE Journal of Biomedical and
Health Informatics, Vol. 17, No. 3, pages 608-618.
Cawley, G. C., 2006. Leave-one-out cross-validation
based model selection criteria for weighted LS-SVMs.
In Proceedings of the International Joint Conference
on Neural Networks (IJCNN’06), pages 1661-1668.
Hargrove, L. J., Englehart, K., and Hudgins, B., 2007. A
comparison of surface and intramuscular myoelectric
signal classification. IEEE Transactions on Biomedi-
cal Engineering, Vol. 54, No. 5, pages 847-853.
Hudgins, B., Parker, P., and Scott, R. N., 1993. A new
strategy for multifunction myoelectric control. IEEE
Transactions on Biomedical Engineering, Vol. 40, No.
1, pages 82-94.
Ishikawa, K., Toda, M., Sakurazawa, S., Akita, J., Kondo,
K., and Nakamura, Y., 2010. Finger motion classifica-
tion using surface-electromyogram signals. In Pro-
ceedings of IEEE/ACIS 9th International Conference
on Computer and Information Science (ICIS), pages
37-42.
Lee, D., and Lee, S., 2011. Vision-based finger action
recognition by angle detection and contour analy-
sis. ETRI Journal, Vol. 33, No. 3, pages 415-422.
Moore, K. L., Arthur F. D., and Anne M. R. A.,
2010. Clinically oriented anatomy. Wolters Kluwer
Health, 6
th
edition, pages 748-750.
Nagata, K., Ando, K., Magatani, K., and Yamada, M.,
2007. Development of the hand motion recognition
system based on surface EMG using suitable meas-
urement channels for pattern recognition. In Proceed-
ings of Engineering in Medicine and Biology Society,
29th Annual International Conference of the IEEE.
IEEE, pages 5214-5217.
Peng, H., Long, F., and Ding, C., 2005. Feature selection
based on mutual information criteria of max-
dependency, max-relevance, and min-redundancy.
IEEE Transactions on Pattern Analysis and Machine
Intelligence, Vol. 27, No. 8, pages 1226-1238.
Schaechter, J. D., Stokes, C., Connell, B. D., Perdue, K.,
and Bonmassar, G., 2006. Finger motion sensors for
fMRI motor studies. Neuroimage, Vol. 31 No. 4, pages
1549-1559.
Specht, D. F., 1990. Probabilistic neural networks. Neural
Networks, Vol. 3, No. 1, pages 109-118.
Tenore, F. V. G., Ramos, A., Fahmy, A., Acharya, S.,
Etienne-Cummings, and R., Thakor, N. V., 2009. De-
HEALTHINF2014-InternationalConferenceonHealthInformatics
66