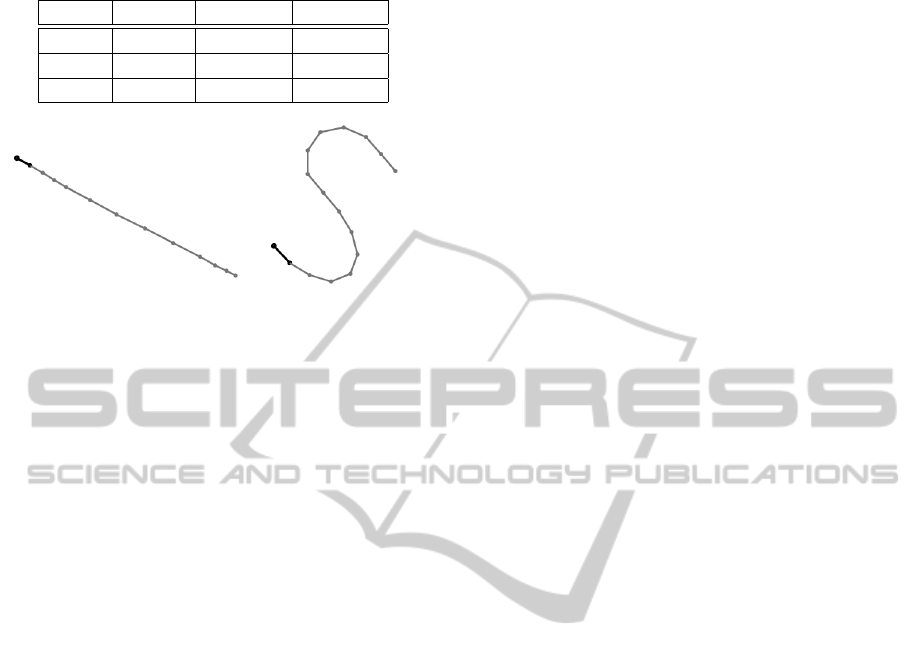
Table 1.
subject distance calculated difference
1 13.70 m 14.10 m 2.9%
2 13.30 m 13.96 m 4.9%
3 12.90 m 13.38 m 3.7%
Figure 10: Movement paths, top view. left: walking over a
straight line with different step length. right: walking an s-
shape trajectory. The black line marks the beginning. Each
step ends with a dot, so that different step lengths can be
seen.
s
r
. As can be seen in Table 1, there is an average error
of 3.8% percent between the actual walked distance
and the calculated distance. That is about 4 cm of
error measurement at every step.
Additionally sensor orientation is used to detect
the direction of movement. One subject was re-
quested to walk in a straight line with small steps
at the beginning, normal step size in the middle and
small steps at the end. As can be seen in fig. 10
the route and the variability in step length can easily
be determined. The developed algorithm works with
non-linear walking routes as well, as can be seen to
the right of fig. 10. For this figure, the subject was
asked to walk an s-shape trajectory with normal step
size. The walking route was determined using the al-
gorithm presented here.
4 CONCLUSIONS
Using raw IMU information and IMU information fil-
tered with a Madgwick filter we were able to outline
a new application to extensively characterize gait pa-
rameters that provide new information about leg ori-
entation and movement direction.
In the future we suggest more studies to outline
the limitations of the developed application. The al-
gorithm for gait analysis needs to be tested with vari-
ous pathological patients and with the results of such
tests, there will be a possibility to automatise long-
term gait analysis. With that in mind, patients suffer-
ing from conditions whose severity can be at least par-
tially assessed by continuous gait analysis can profit
from the monitoring application presented in this pa-
per.
ACKNOWLEDGEMENTS
The authors would like to thank Karl Dubies and
Michael Stuber for assistance with the data acquisi-
tion. This work was supported by a grant from the
Ministry of Science, Research and the Arts of Baden-
Wuerttemberg (Az: 33-7533-7-11.6-10/2).
REFERENCES
Begg, R., Palaniswami, M., and Owen, B. (2005). Sup-
port vector machines for automated gait classifica-
tion. Biomedical Engineering, IEEE Transactions on,
52(5):828–838.
Bishop, C. M. (2006). Pattern recognition and machine
learning. Springer, New York, NY.
Chung, P.-C., Hsu, Y.-L., Wang, C.-Y., Lin, C.-W., Wang,
J.-S., and Pai, M.-C. (2012). Gait analysis for patients
with alzheimer’s disease using a triaxial accelerome-
ter. In Circuits and Systems (ISCAS), 2012 IEEE In-
ternational Symposium on, pages 1323–1326.
El-Gohary, M. and McNames, J. (2012). Shoulder
and elbow joint angle tracking with inertial sen-
sors. Biomedical Engineering, IEEE Transactions on,
59(9):2635–2641.
Fischer, C., Talkad Sukumar, P., and Hazas, M. (2012). Tu-
torial: implementation of a pedestrian tracker using
foot-mounted inertial sensors. Pervasive Computing,
IEEE, PP(99):1–1.
Gafurov, D., Snekkenes, E., and Bours, P. (2007). Spoof
attacks on gait authentication system. Informa-
tion Forensics and Security, IEEE Transactions on,
2(3):491–502.
Jung, Y., Kang, D., and Kim, J. (2010). Upper body mo-
tion tracking with inertial sensors. In Robotics and
Biomimetics (ROBIO), 2010 IEEE International Con-
ference on, pages 1746–1751.
Madgwick, S., Harrison, A. J. L., and Vaidyanathan, R.
(2011). Estimation of imu and marg orientation us-
ing a gradient descent algorithm. In Rehabilitation
Robotics (ICORR), 2011 IEEE International Confer-
ence on, pages 1–7.
Patterson, M. and Caulfield, B. (2011). A novel approach
for assessing gait using foot mounted accelerometers.
In Pervasive Computing Technologies for Healthcare
(PervasiveHealth), 2011 5th International Conference
on, pages 218–221.
Perry, J. (2003). Ganganalyse: Norm und Pathologie des
Gehens. Urban & Fischer, Munich, 1. aufl. edition.
Sant’Anna, A., Salarian, A., and Wickstrom, N. (2011). A
new measure of movement symmetry in early parkin-
son’s disease patients using symbolic processing of
inertial sensor data. Biomedical Engineering, IEEE
Transactions on, 58(7):2127–2135.
Terada, S., Enomoto, Y., Hanawa, D., and Oguchi, K.
(2011). Performance of gait authentication using an
acceleration sensor. In Telecommunications and Sig-
nal Processing (TSP), 2011 34th International Con-
ference on, pages 34–36.
BIODEVICES2014-InternationalConferenceonBiomedicalElectronicsandDevices
132