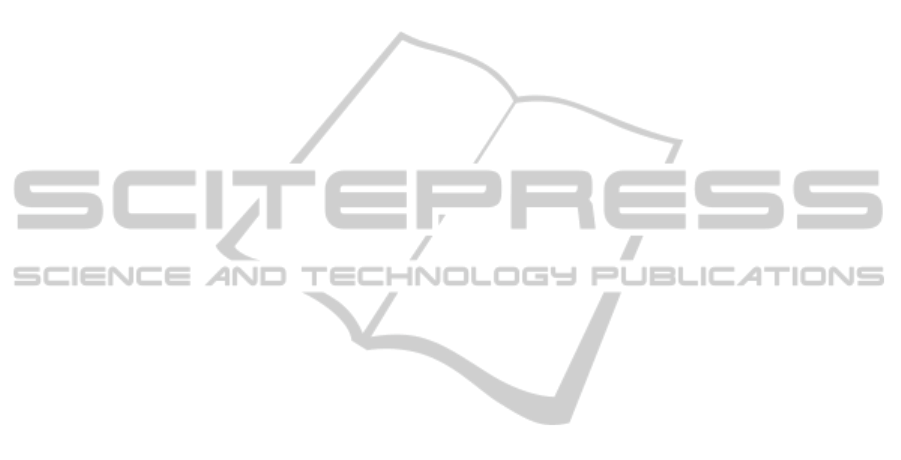
is summarized in Figure 1.
A total of 15 subjects (5 male and 10 female, age
25-35) with no pathologic tremor conditions were re-
cruited. All subjects were screened as per confiden-
tial medical questionnaire. During the test the pan-
ellist was seated, with the right arm placed on the
rest of their chair. The tremor response was mea-
sured by means of a 3-axis GENEA device attached
to the back of the subject’s hand. The GENEA is an
acceleration sensor developed by Unilever Discover
(Colworth, UK) which is now distributed by Activin-
sights Limited (Kimbolton, UK). The reliability and
performance of this sensor has been validated in sev-
eral studies (Esliger et al., 2011; Zhang et al., 2012).
The sensor is wireless, storing all measured data in a
flash memory. Data download and initialization of the
sensor is accomplished by means of a bespoke hub.
During the exploratory analysis it was found that one
panellist is a potential outlier. An inspection of the
study records revealed that this panellist did not fol-
low the PASAT protocol - thus this panellist has been
removed from the subsequent analysis, leaving data
from 14 subjects (8 control, 6 intervention).
3 SIGNAL PROCESSING AND
FEATURE EXTRACTION
The accelerations were recorded with a sampling rate
of 80 Hz. For the following only the vertical acceler-
ation of the hand, a
z
, is considered. After smoothing
the signal by means of a moving average, the empiri-
cal mode decomposition (EMD) is used to extract the
tremor signal (see (de Lima et al., 2006)). Because
EMD employs an adaptive process to compute the
signal envelope, it is appropriate for the analysis of
nonstationary signals such as tremor. It is not a priori
clear which intrinsic mode functions (IMF) contain
the tremor signal. An inspection of the resulting fre-
quency spectra was used as a guide, whereupon it was
decided to use the sum of the first two IMF as tremor
signal.
Typical tremor signals appear as noisy oscillations
with one or two dominating frequencies (see Fig. 2).
Such time series s(t) can be modelled by a damped
noise-driven oscillator model (Timmer et al., 2000).
To accomodate spectra with two peaks we adopt a 4th
order autoregressive model as follows:
s(t) = a
1
s(t − 1) + a
2
s(t − 2) + a
3
s(t − 3)
+a
4
s(t − 4) + ξ(t) ;
(1)
where ξ(t) is the driving noise. A test using the partial
autocorrelation of the signals confirmed the validity
of this model. The coefficients a
i
reflect mechanical
properties of the hand and attached muscles, which is
not of interest here. The strength of the driving noise,
however, defines the amplitude of the tremorous oscil-
lations, which suggests to use the variance as measure
of the tremor strength:
SD = Var (ξ) . (2)
The noise variance is estimated by standard statistical
methods.
To measure changes of the tremor signal structure,
the fractal dimension FD for one dimensional time se-
ries can be used. This measure is related to the rough-
ness of the signal. The fractal dimension equals 1 for
smooth signals, however, for rough signals it takes
values between 1 and 2. It has been used success-
fully for the modelling and analysis of multi-scaled
random processes and ecological data (Halley et al.,
2004). A change of the fractal dimension may indi-
cate a change in the underlying mechanism of tremor
generation. For the computation of the fractal dimen-
sion we used the R package fractaldim, selecting the
madogram estimator (Gneiting et al., 2010).
4 RESULTS
Typical frequency (power) spectra of the tremor sig-
nal before and after the stress intervention are shown
in Figure 2 for the control and stress condition. The
effect of the the stress intervention is clearly visible,
resulting in an increase of the total power and an en-
hancement of lower frequencies around 6 Hz. In con-
trast, the control condition (listening to classical mu-
sic) leads to a reduction of the total power.
The effect of the stress intervention on the tremor
strength SD is compared with the control in the box-
plot Figure 3. Here SD
1
and SD
2
are the tremor
strength before (baseline) and after the intervention.
These results confirm that the stress condition in-
creases the magnitude of SD, in contrast to the
control condition that tends to decrease the tremor
strength. In order to validate the effect of stress on
the tremor strength SD, ANOVA (analysis of vari-
ance) is used to quantify the difference between the
log-ratios log(SD
2
/SD
1
) of the stress and the con-
trol condition. The ANOVA, based on 1 and 12 de-
grees of freedom and F
1,12
= 8.34, results in a p-
value of 0.014, i.e., good evidence that the stress con-
dition indeed increases the strength of tremor. We
now consider the fractal dimension FD. As shown
in Figure 4, the stress condition leads to a decrease
of FD. Because the distributions of FD are slightly
skewed and have different variance for stress and con-
trol, we first use the non-parametric Kruskal-Wallis
AControlledStudyforMeasuringStressInducedChangesofPhysiologicTremorwithaWearableActivitySensor
179